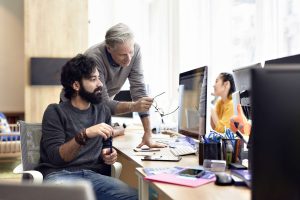
In my previous post I shared a story about a recent hiking trip ruined by spotty cell reception. My group decided to overcome those technical challenges on our next hiking trip by heading to Saylorville Lake, a man-made lake constructed as a reservoir and flood control system for the Des Moines River. The lake is surrounded by a series of hiking trails with various degrees of difficulty. My group chose the Lakeshore Trail, a five-mile looping trail near the water’s edge offering great views of the lake. We had trail maps in hand and solid cell reception.
Unfortunately, during the previous month, near-record amounts of rain rose the river well above flood levels for almost two weeks. Although the flood waters had long since receded, no matter which trail head we tried (and we tried them all) we kept finding large sections of the hiking trail underwater or washed away.
This made me think of the rising popularity of data lakes, a storage repository holding a vast amount of raw data in its native format – structured, semistructured and unstructured. A data lake is essentially a reservoir and flood control system for big data, allowing for the rapid ingestion of large data volumes before data structures and business requirements have been defined for its use. Implementing a data lake can enable a business to gain access to data they haven't been able to get in the past, and make it available for data discovery, proofs of concept, visualizations and advanced analytics.
That’s the theory at least.
In practice, many data lakes quickly devolve into a poorly managed and ungoverned data dumping ground akin to a data swamp. An undocumented and disorganized data lake is like those underwater and washed away trails at the flooded lake we tried to hike. It’s nearly impossible to navigate. It’s also difficult to trust the data that can be found, and it's challenging to make the data useful for enterprise applications.
It’s important to understand that a data lake is not a platform for data. A data lake is a container for multiple collections of varied data coexisting in one convenient location. You need a comprehensive platform to generate the most business value from a data lake – and that requires integration, cleansing, metadata management and governance. This approach to data lake management enables analytics to correlate diverse data from diverse sources in diverse structures resulting in more comprehensive insights for the business to leverage.
Download a paper – SAS: A Comprehensive Approach to Big Data Governance, Data Management and Analytics