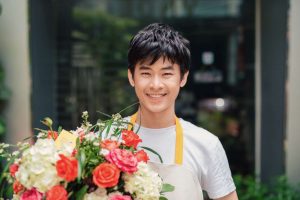
The term customer data management refers to the ways in which businesses keep track of their customer information and survey their customer base to obtain feedback. Customer data management has been around since the 1990s and is still relevant today. After all, if you don’t have good data about your customers, how can you understand who they are and make good decisions about them? So, you may ask: How do I get good data about my customers? The answer – good data quality – sounds deceivingly simple.
In a recent survey by Experian, 72 percent of organizations globally say data quality issues affect consumer trust and perception. And a lack of customer or citizen trust costs organizations – in terms of lost revenue, substandard customer service, increased risk, barriers to innovations and new initiatives, and issues with compliance. Acknowledging how long customer data management has been around, it's notable that trust in customer data is still a major issue for many organizations.
How do we fix data quality issues for customer data management?
Many organizations try to resolve data quality issues by writing code. If this is your approach, you're missing the mark. Writing code to resolve data quality issues is not a best practice for a sustainable, enterprise approach to customer data management. A time-consuming, one-off approach will never get your company the reliable customer data needed for the long-term. It also won't allow you to effectively build a single view of the customer. There are other challenges with writing code to fix data quality issues, too:
- Most organizations are not good at documenting their code generation process. When the person who wrote the code to fix the data leaves the company or changes jobs, their code has to be reverse engineered, or the department has to start over from scratch.
- Visibility into what actions were taken to clean the data is virtually nonexistent for the user community. Executives and individuals in business units don't have the skills to look at the code and understand how the data was cleansed. This creates a lack of trust in the data that's used for decision making.
Logic dictates that good data quality management is a key enabler for any customer data management initiative. But data quality is a complex operation. So you'll need proven, comprehensive data quality software to give you the repeatable, scalable approach you need to resolve bad customer data.
What does data quality software do for customer data management?
Good data quality tools have built-in, industry-proven transformations that reveal the relationships across various data elements. The data quality environment can quickly understand the tokens of the data. As a result, they can put it in the right place for processing regardless of what the system of record says the data is – and without manual effort.
Standardize the data
Industry-leading data quality software can standardize data into a consistent format and meaning. Such software knows that Peg, Peggy and Marg are nicknames for Margaret in the US, Canada and the UK. So it can standardize the name to a simple value, like Margaret, for better data alignment and matching. Such data quality software also needs to consider the country of origin for the data (without requiring human intervention). For example, in the US, Don is a nickname for Donald – but in Spain, Mexico and Italy it’s an honorific. Data quality tools need to understand the entire data row and apply the correct data standardization and tokenization processes in an automated, repeatable way.
Enrich the data
Enriching the data is another important piece of successful customer data management. Data quality software should verify and correct addresses for an accurate view of the customer and to better align the data for the matching process. Other enrichment capabilities are desirable, too. For example, assigning "gender" to a record can enhance marketing efforts because it could help you understand the demographics of customers. Latitude and longitude information appended to the address may help you understand things like distance to a store, tax rate assignments and delivery planning.
Use matching to combine records
Bringing the data together via matching is another vital element of successful customer data management. Matching will enable you to combine all the records you have for a given customer into a single best record for that individual. By seeing the details of your relationship with a single customer, you can put in place the best possible marketing or customer service program for that customer. Better yet, you can automate this process so you won’t have to do it manually.
The bottom line: If you want to have good customer data management, you first need to use trusted data quality technology. By empowering you to truly know your customers, data quality is key to improving customers' trust and the overall perception of your company.
Download a paper to learn how SAS supports multiple facets of big data governance, management and analytics