In the last few days, I have heard the term “data lake” bandied about in various client conversations. As with all buzz-term simplifications, the concept of a “data lake” seems appealing, particularly when it is implied to mean “a framework enabling general data accessibility for enterprise information assets.” And of course, as with all simplifications, the data lake comes bundled with presumptions, mostly centered on the deployment platform (that is: Hadoop and HDFS) that somewhat ignore two key things: who is the intended audience for data accessibility, and what do they want to accomplish by having access to that data.
I’d suggest that the usage scenario is obviously centered on reporting and analytics; the processes access the data used for transaction processing or operational systems would already be engineered to the application owners’ specifications. Rather, the data lake concept is for empowering analysts to bypass IT when there are no compelling reasons to have to endure the bottlenecks and bureaucracy that have become the IT division’s typical modus operandi.
As I suggested in my last post, we can be thoughtful in separating the execution of the data lake strategy from the decision about platform. Different analysts perform different kinds of analyses, using different methods and tools. Some use visualization and discovery tools, while others iteratively interleave ad hoc queries with reviews of the result sets to narrow the scope of a particular investigation. Some data requests just filter the data, others reorganize the data by dimensions, and others involve more complex algorithmic clustering, segmentation, or sequence mining, for example.
In other words, from a consumer perspective one size does not necessarily fit all, and the a priori choice of a single platform indicates a misunderstanding of
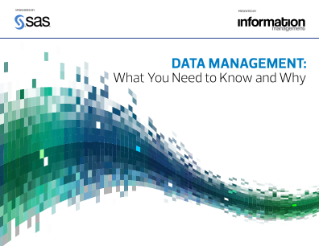
the conflation of adoption of technology with a default expectation of its advertised performance. The problem occurs down the line, when the budgets have been spent, and the advertised performance never materializes.
There is a simple suggestion to avoiding this pitfall: assess the consumer data usage scenarios and understand the users’ collective performance variables and expected thresholds before deciding on a single deployment platform. You will most likely find that the idea of a monolithic data lake (a little bit of a mixed metaphor I’ll admit) will give way to a hybridized environment for data management that will need some additional tooling to retain the opacity for data accessibility.
And as I suggested in my prior note, my next post will look at two facets of those tools: data ingestion and integration (on the one side), and facilitation of access (on the other).