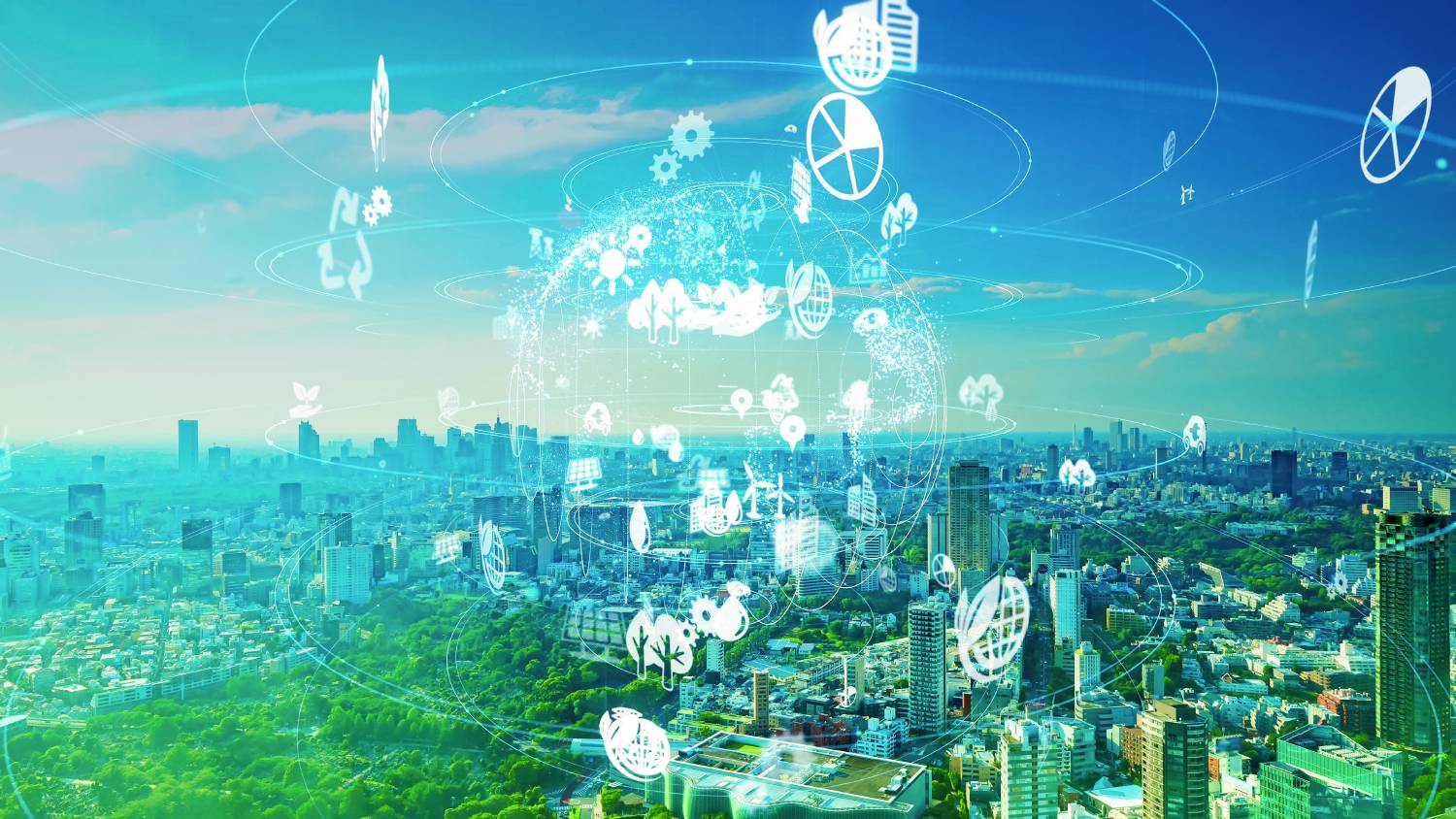
The concept of sustainability has evolved significantly over the past few years. It is no longer just a trendy buzzword but has become an essential element of business models. Major multinational companies such as IKEA, PepsiCo and Amazon lead sustainability transformation by setting ambitious goals and implementing new initiatives. IKEA