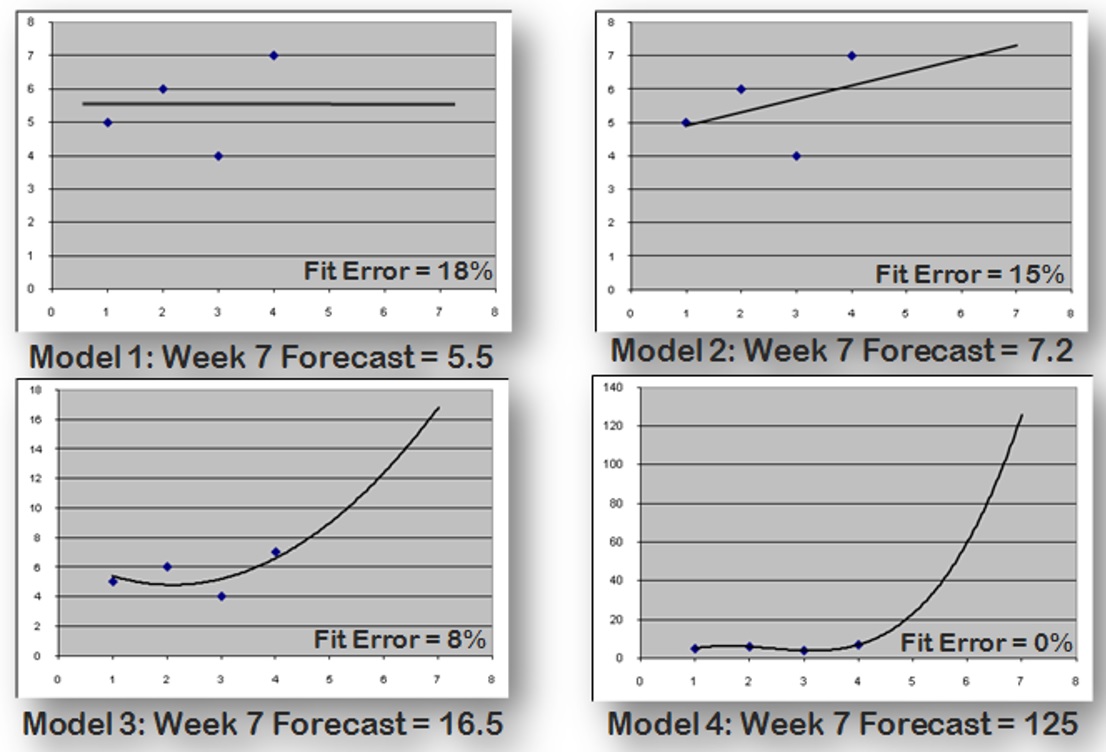
Why the Attraction for the Offensive Paradigm? In addition to the reasons provided by Green and Armstrong, I'd like to add one more reason for the lure of complexity: You can always add complexity to a model to better fit the history. In fact, you can always create a model