Medication adherence is the degree to which a patient correctly follows medical advice. In this post, we will be referring to medication or drug compliance. It has long measured patient health outcomes for commercial and government health care organizations. However, per Dr. Marie T. Brown and Dr. Jennifer K. Bussell, only half of patients adhere to their chronic medication. The SAS Applied AI and Modeling division within SAS R&D will soon release the SAS Medication Adherence Risk model. This model will help end users identify patients at risk of being non-adherent at the start of medication therapy. This post will provide an overview of the SAS Medication Adherence Risk model. Additionally, we will also cover how it can be used to help improve medication adherence.
What makes the SAS Medication Adherence Risk model unique?
The SAS Medication Adherence Risk model uses machine learning to identify patients’ risk of being non-adherent based on past patient behavior, patient demographics, and social determinants of health data. It is designed to make modeling easy and accessible for everyone, regardless of their statistical background. Some key features include:
- Building a prediction model and scoring code tailored to the end user’s specific population and drug class
- Determining the patient’s risk of being non-adherent starting from the first fill for a new drug class using a score code produced by the prediction model
- Establishing both the pharmacy and ordering provider most frequently associated with a patient to help aid in care coordination using historic pharmacy claims before starting a new medication
- Identifying geographic areas containing large concentrations of high-risk patients based on the results of the model
- Using multiple model validation checks to help aid in running the model and prevent end-user error
- Incorporating social determinant data to help mitigate the inherent bias associated with health care data
How does the model work?
The SAS Medication Adherence Risk model consists of both a model pipeline and risk scoring. The model pipeline uses historical data to build a gradient-boosting model. That, in turn, generates a score code specific to the end user’s population and drug class of interest. Once the score code is generated, end users can supply a list of new users initiating therapy with the drug class(es) of interest and obtain the new user’s probability of being non-adherent.
What are the results of the SAS Medication Adherence Risk model?
Results include the following:
- Model Scoring Code -> Model scores based on historical data
- Model Fit Statistics -> Table containing fit statistics from both the testing and training data
- Variable Importance Statistics -> Table containing variable importance statistics from the final model
- New User Risk Scores -> List of patients initiating medication therapy, patients’ probability of being non-adherent, and model parameters used to score the patient
The model results can be easily incorporated into reports, allowing for dissemination in an easy-to-consume format. For SAS Viya users, the SAS Medication Adherence Risk product includes a SAS Visual Analytics JSON file that can be imported and configured using the model results. Results can also be incorporated into tools like Tableau or Microsoft PowerBI. However, the report JSON file included in the SAS Medication Adherence Risk model will only produce a report when incorporated into SAS Visual Analytics.
Figure 1 is a screenshot from an example report in SAS Visual Analytics. It was created based on the SAS Medication Adherence Risk model results. The JSON file that creates the report shown in Figure 1 is included in the offering container.
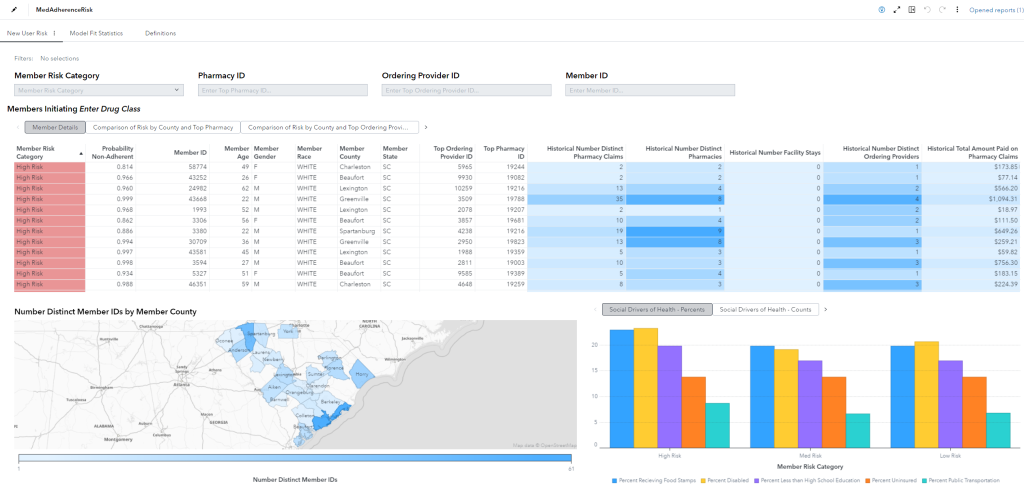
Use Cases
Results from the SAS Medication Adherence Risk model can be used to determine which providers, patients, or geographic regions might need additional resources to help improve medication adherence rates. Output can be used to help answer the following questions posed by end users such as health care plan directors/managers, pharmacy benefit managers, and/or patient care coordinators:
- Which pharmacies or ordering providers are associated with the largest number of high-risk patients?
- Which patients are at high risk of being non-adherent?
- Which geographic areas have the highest concentration of high-risk patients?
- What factors seem to be predictive of non-adherence?
- Which high-risk patients are most likely to benefit from additional resources?
Summary
The SAS Medication Adherence Risk model enables end users to identify which patients are at high risk of being non-adherent to their medication therapy. By knowing patients’ risk, interventions such as increased care coordination, patient education, or patient behavioral support can be provided to help patients remain adherent. Results from the model enable end users the ability to identify where resources are needed to help prevent adverse outcomes caused by non-adherence. For more information about strategies to improve medication adherence, please see the following recommendations from the “Millions Hearts” initiative.