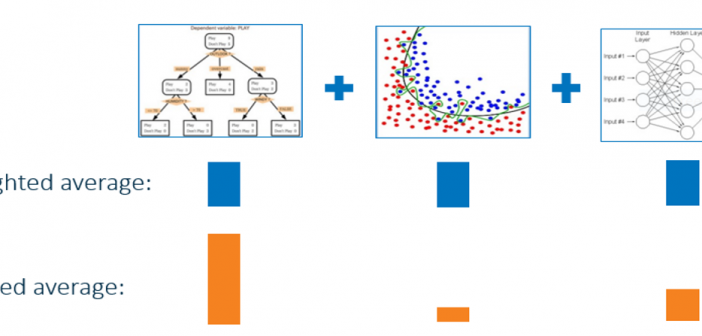
This is the third post in my series of machine learning techniques and best practices. If you missed the earlier posts, read the first one now, or review the whole machine learning best practices series. Data scientists commonly use machine learning algorithms, such as gradient boosting and decision forests, that automatically build