Soccer has been on my mind since the recent tournament in Russia, and fraud is always on my mind. Fighting fraud today means thinking about artificial intelligence (AI) and machine learning (ML). Everyone says so. Like me, you probably wonder how AI and ML work – especially in fraud.
What is AI and ML?
There is a difference between AI and ML.
Artificial intelligence is training machines to perform human tasks, essentially to emulate humans, and uses modeling. Modeling is repeatedly running a scenario to predict the most likely outcomes – maybe thousands of times.
Machine learning is a subset of AI and focuses on training a machine how to learn. There is a machine learning life cycle. This includes collecting data, training the algorithm, trying it out, collecting feedback, using feedback to improve, and repeating. This is a continuous improvement loop for the algorithm.
Algorithm is a scary math word for a set of directions for performing a task. Both AI and ML use them. To improve, adjust the algorithm to get better results.
Let’s think about ML and AI by comparing them to soccer and adding a fraud spin.
Photo by Jannes Glas on Unsplash
Goalkeeping with Advanced Analytics
My daughter was a goalkeeper, now a coach, and thinking about her training helps me understand AI and ML.
In the fight against fraud, you would be the goalkeeper of Team Fraudbusters. You defend, but as goalkeeper you also organize and run the defense – a key position.
A goalkeeper needs to spot danger, predict what might happen, react and adjust until the scoring threat is gone. AI is similar, taking the intelligence garnered from machine learning and applying it the way a person might.
My daughter was trained on goalkeeping. Training a goalkeeper is like training a ML algorithm.
Giovanni, the head goalkeeper coach, ran her through drills on positioning, diving, tackling, catching, punching, etc. in isolation. The drills were observed, feedback given, adjustments made, and the process was repeated. She learned and improved over time. This is like the machine learning life cycle.
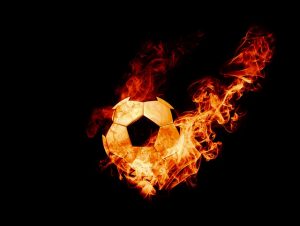
A second coach, Remus, helped during team-play practice. This would be scrimmages against the older youth team which was nationally ranked. He would stand beside her goal and point out offensive formations and attackers. He would also tell her what to watch for – a pass, cross, or shot. When the attack was over, Remus would go over what had just happened and tell her what to do to improve the next time. So, she could use this knowledge in a real game to be a better goalkeeper.
This is like training an AI algorithm to recognize visual patterns and verbal cues, spot changes, predict next moves, and react to them.
Goalkeeping Against Fraud with ML and AI
Machine learning algorithms can also analyze historical data for patterns and significance.
In your match against Team Fraud, which opposing players are the biggest threat to you? Who is assisting the Team Fraud strikers? What combination of opposing players is the most lethal to your defense? This is like scouting the other team using the available team and player stats data.
You need machine learning to be the best defender possible.
Clearly, there is tons of data on each opposing player that you could study before the game to help you be a better fraud defender – but which data is most important?
If you had ML driving your defensive analytics, how might this give you an advantage? With ML, your complex data is converted into intelligence to help you prepare.
Strikers are more likely to score goals, but with ML you might discover Team Fraud has a quick defender that can also strike which might otherwise be lost in your mountain of data – leaving you unprepared and your goal an easier target.
With ML, you would know their style of play and can better predict what you might be facing when the first whistle blows. You might learn Team Fraud often scores off set pieces. So, avoid giving up corner kicks if possible. Prepare defenders to recover quickly if your opponent likes to counter attack.
And like the real world, a soccer match isn’t a static point-in-time event – it’s complex, moving and changing in real time. Team Fraud will adjust its own attack to your defense to try to defeat you. How will you respond? You’ll need AI for identifying changes in real-time to help you.
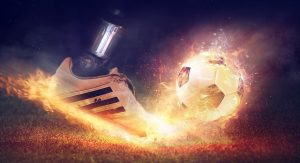
Using AI would be like replacing yourself in the goal with the machine – spotting patterns, reacting, and defending. AI can adjust itself to updated data allowing you to see new patterns and new attacks as they are forming, which you might not be to able analyze yourself while playing the game - and why every player isn't a professional.
Defense Using ML and AI for Predictive Analytics
Human goalkeepers don't start working only when there is a shot at their goal, they organize the defense by watching formations, calling off marks to defenders, and trying to predict their opponents next moves to prevent shots on their goal. This is how predictive analytics works using ML and AI which can stop fraudulent payments before your rules-based system pays them. This limits pay-and-chase costs and increases return on investment.
This deep insight makes you an even better fraud defender – and more likely to win!
In soccer, you defend each shot. Imagine the best goalkeeper in the world defending with ten balls in play during the game. The human brain couldn’t process that much data quickly enough. Even the spectators couldn’t keep up.
Automated payment processing may mean millions of transactions each day. You couldn’t defend them yourself, even with rule-based systems which fraudsters frequently circumvent by making fraudulent claims look real.
You need the super-goalies ML and AI to help you fight fraud effectively.
Team Fraud is playing to win, are you ready with ML and AI to defend your organization?
This post was inspired by Shaun Barry.