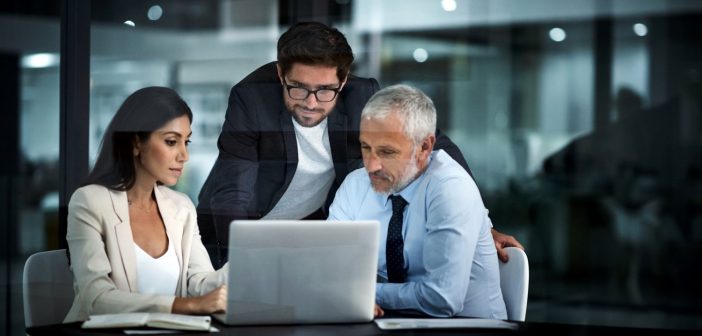
There's been a lot of hype regarding using machine learning (ML) for demand forecasting, and rightfully so, given the advancements in data collection, storage, and processing along with improvements in technology. There's no reason why machine learning can't be utilized as another forecasting method among the collection of forecasting methods