Who has time to be a nutritionist between work deadlines and swim practice? Not this working mom! But my tiny human needs her fuel, you know? This is why I’m thankful for nutrition labels.
A quick scan at the grocery store tells me if that cereal is all sugar bombs or has some whole grains hiding in there. The same goes for those yogurt tubes – I need to make sure they are packed with protein, not just artificial flavors. Those labels are my secret weapon for ensuring my family eats healthy, even on busy nights.
You might be thinking: Nutrition labels are great for moms on the go, but what do they have to do with fancy AI models? While AI models might not need a balanced diet, the concept of a “nutrition label” for AI can be surprisingly useful. Model cards are those nutrition labels used by everyone from AI developers to decision makers.
Through model cards, users can get a comprehensive overview of an AI model’s components, like how a nutrition label details food ingredients and nutritional value. Model cards provide transparency and insight into models, helping AI users make wise and ethical choices.
Even if you’re not counting calories, we’ve all encountered report cards, user reviews or instruction manuals. These examples capture the fundamental aspects of model cards and why we need them. So, let’s discuss the value of model cards using nutrition labels as an analogy.
Model cards are nutrition labels for AI models
Model cards serve as a transparency vehicle, benefiting everyone involved in the analytic life cycle, from creators and modelers to decision makers. This transparency promotes the reliable and ethical use of technology.
Just as a nutrition label lists a food’s ingredients, a model card details an AI model’s training data, development process, accuracy, model drift, related fairness assessments and governance details. These elements are crucial in keeping things transparent, as they comprehensively understand how the model was created and performed. This transparency helps identify the model’s strengths and limitations, promoting trust and encouraging responsible and ethical use by all stakeholders.
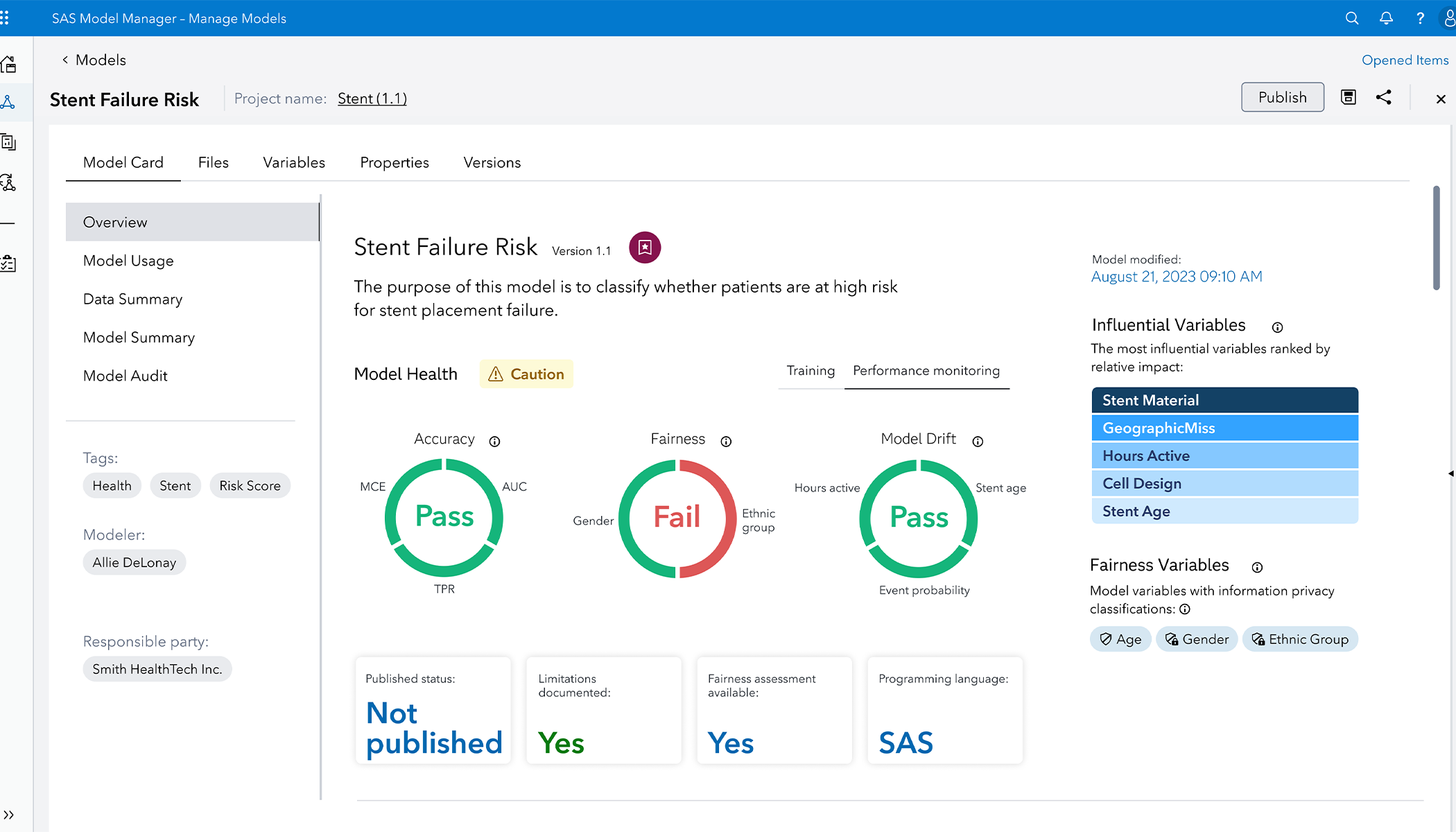
Understanding your AI model's "serving size"
When reading the serving size on a nutrition label, you understand how much food you should consume. Similarly, the model usage section of a model card helps you understand the intended use case of an AI model. Just as serving size informs you about portion control, the intended use case tells you what specific problems the model is designed to address.
Food labels also list potential allergens or warnings for specific dietary needs. In the same way, the model usage section of a model card highlights the model’s limitations and out-of-scope use cases. Model cards inform users of inappropriate applications and show what the model can and cannot do. This helps prevent misuse and promotes responsible deployment.
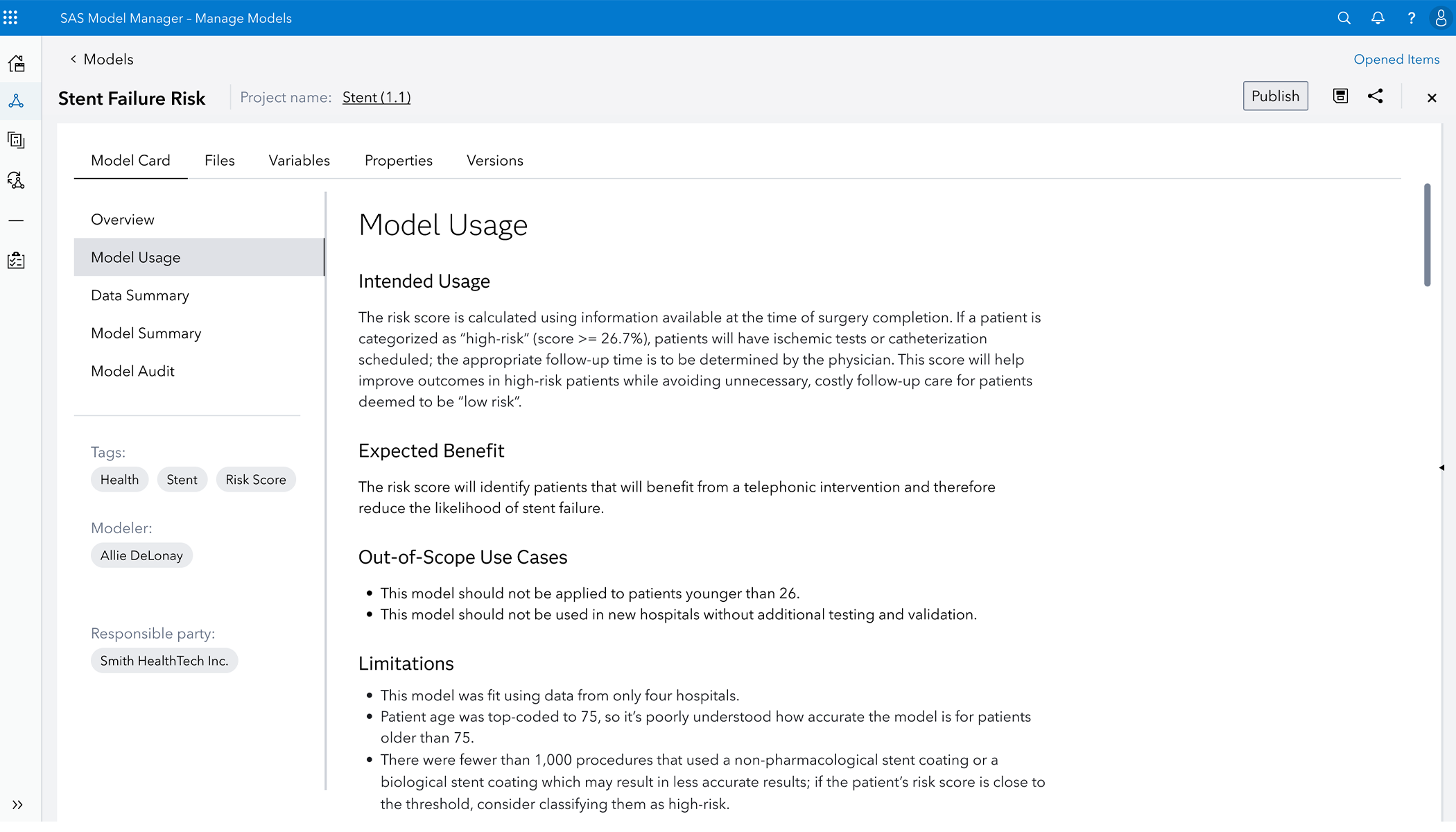
Unveiling the recipe for good data
In the analogy of a nutrition label, the data summary in a model card is like the ingredients list on a food package. An ingredients list details the components of your food, helping you understand what you’re consuming. Similarly, a data summary in a model card outlines the specific analysis of the data the AI model was trained on, providing insight into the foundation of the AI’s decision-making process.
Key components of a data summary include:
- Source of the data: Just as you might check if the ingredients in your food are organic or locally sourced, the data summary reveals where the data comes from. Knowing whether a model is sourcing data from reliable and ethical origins is crucial for trust and transparency.
- Privacy classifications: Like food ingredients labeled to indicate potential allergens, data elements in a model card come with privacy classifications. This tells users about the sensitivity of the data, addressing privacy concerns.
- Data set tags: These tags provide additional context about the data, much like nutrition labels provide details on vitamins and minerals. Tags can indicate the type of data, its relevance and the data’s role in training the model. These could be useful to convey if synthetic data was used in training the model.
- Relevant analysis: As food labels might note things like calorie count and sugar content, the data summary includes completeness, approval status and indication that the data contains outliers. This helps users understand any anomalies or significant relationships within the data that might affect performance.
Understanding these components helps users gauge the reliability and suitability of the AI model for their specific needs.
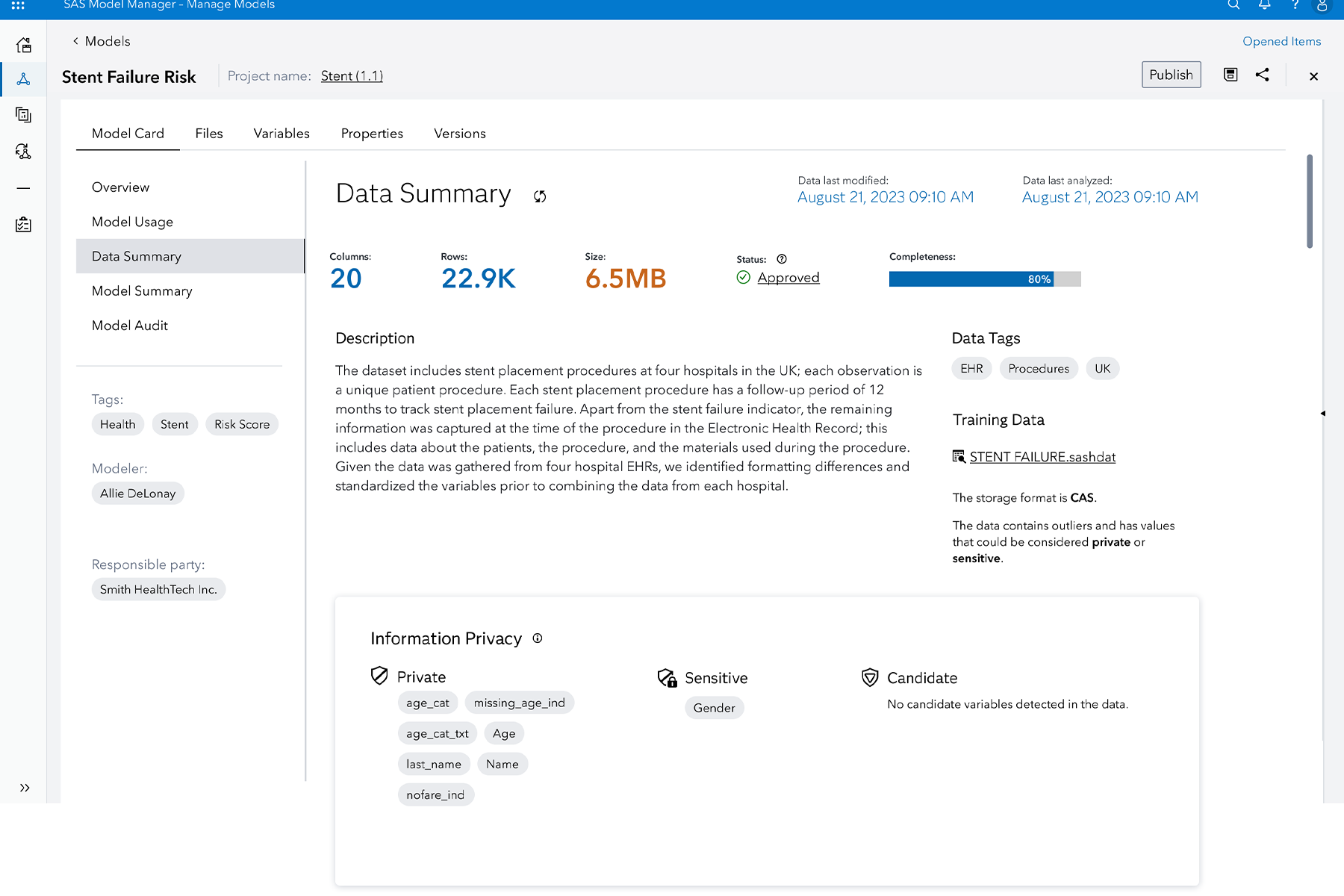
Model summaries and percent daily values
Like metrics for percent daily values metrics on food labels, the model summary of a model card offers crucial information about an AI model’s performance. Like data summaries – but different – model summaries include accuracy testing percentages, performance and prediction-bias metrics, and information about model generalizability. These details are crucial for understanding how well the AI model performs and where it might have limitations.
For an AI model, the model summary might include:
- Accuracy metrics indicate how reliable the AI model is, similar to daily nutritional values on food labels.
- Performance metrics that detail various performance metrics akin to calorie counts or vitamin content on food labels.
- Prediction bias metrics highlight biases in the AI model’s predictions, much like checking for unhealthy ingredients.
- Generalizability shows how well the AI model performs across different data sets and conditions. This is like getting the proper nutritional value across different meals.
- Model interpretability includes variable importance plots that indicate which predictor variables are most useful for predicting the response variable for the model.
- Fig. 4.1: The model summary section of the model cards offers a concise overview of the model’s architecture, training process, and performance evaluation.
- FIg. 4.2: A continuation of Fig. 4.1.
Balance your AI palate with model audits
Just like a balanced diet keeps your family healthy, a model audit ensures that your AI functions correctly, remain unbiased and delivers reliable results. Like a healthy family, the AI will be better equipped to tackle challenges effectively.
A model audit provides detailed information on key aspects of AI, such as the recency of results, performance metrics, fairness assessments, and model-drift metrics. It also lets users select the metrics that meet their specific audit requirements, like choosing ingredients for a balanced meal.
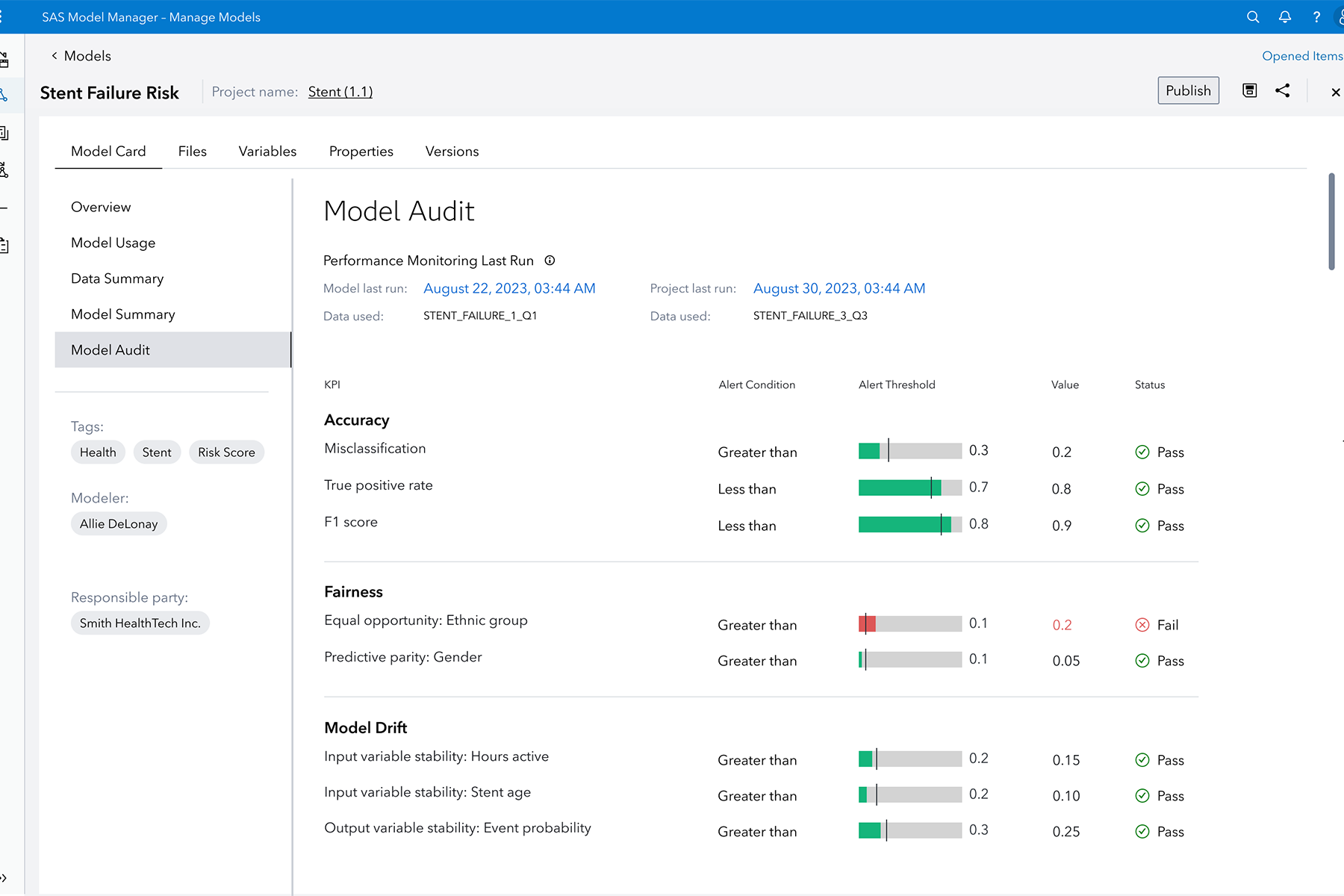
Model cards serve as essential transparency tools for AI models. They provide crucial information about an AI model's “ingredients,” such as its training data, development process, performance metrics and limitations. Understanding these details helps users make informed and responsible decisions about how to use AI effectively.
Discover how to work with AI responsibly, consistently and in a trustworthy way
WANT MORE GREAT INSIGHTS MONTHLY? | SUBSCRIBE TO THE SAS INSIGHTS NEWSLETTER