So now you're ready to make decisions with your models. You’ve asked many questions along the way and should now understand what’s all at play. But how can you ensure these decisions are trustworthy and ethical?
Transparency is crucial. Sharing the reasoning behind our choices in relationships, whether at home or work, even difficult ones, fosters trust and open communication. This transparency helps people to understand the "why" and feel invested in the decisions that impact them.
Transparency is equally important in AI-driven decisions. Human oversight plays a vital role in explaining AI recommendations. While AI can improve decision-making, trust requires transparent algorithms and human oversight for ethical outcomes.
In this blog series, decisioning is the last of the five pivotal steps of the AI life cycle. These steps – questioning, managing data, developing the models, deploying insights and decisioning – represent the stages where thoughtful consideration paves the way for an AI ecosystem that aligns with ethical and societal expectations.
I've identified five questions we should ask when making decisions based on AI-powered insights.
1. Have you identified if an AI system made the decision?
Imagine you apply for a loan and are rejected. You must understand if a human loan officer or an AI algorithm made this decision. An AI-based system might deny your request based on pre-set criteria that don't consider your specific situation (job change, medical bills). A human review of the AI's decision allows for that nuance.Social media platforms often personalize your news feed based on AI algorithms. Knowing your feed is curated by AI lets you be aware of potential bias and actively seek out diverse viewpoints to avoid an echo chamber of information. Identifying AI decisions promotes transparency. It helps to understand the reasoning and potential biases behind outcomes.
2. Is the decisioning process fully explainable?
If a doctor presents a diagnosis based on AI analysis of medical scans, it's important to know why. This helps a patient ask questions about the AI's confidence level and limitations and ensures the doctor considers other factors beyond the AI's output. Explainable AI sheds light on why AI systems make certain decisions, fostering trust and allowing humans to identify potential biases. This transparency improves decision making by making it accountable and understandable.
3. What mechanisms are in place to check for unintended consequences from an AI-based decisioning system?
Several mechanisms can help us identify unintended consequences of AI decisions, and having some in place is important. Mechanisms like impact assessments can predict potential social and ethical issues before deployment. We can also use counterfactual explanations to understand how different inputs would have changed the AI's decision. Finally, monitoring and auditing using model cards for real-world outcomes helps in adjusting after deployment.
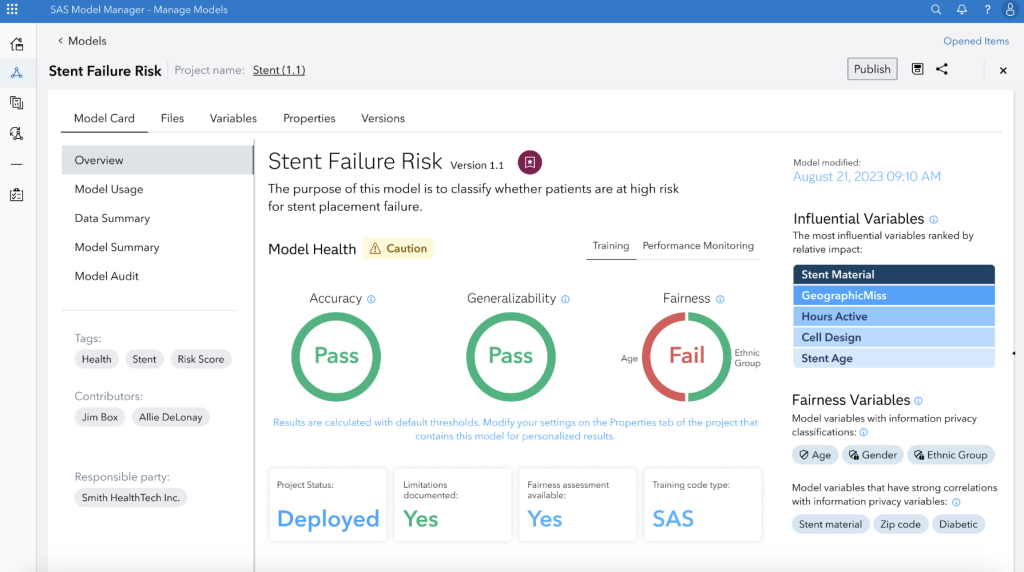
4. Does your system have the capability to intervene or override the AI system's decisions, when necessary, by human operators?
Human intervention in AI decision making acts as a critical safety net. AI excels at analyzing vast amounts of data and identifying patterns, but it can need help with the complexities of ethics and unforeseen consequences. Humans bring moral judgment and a broader understanding of the situation. This enables us to override AI decisions that might be discriminatory, unfair or illogical based on unexpected scenarios.
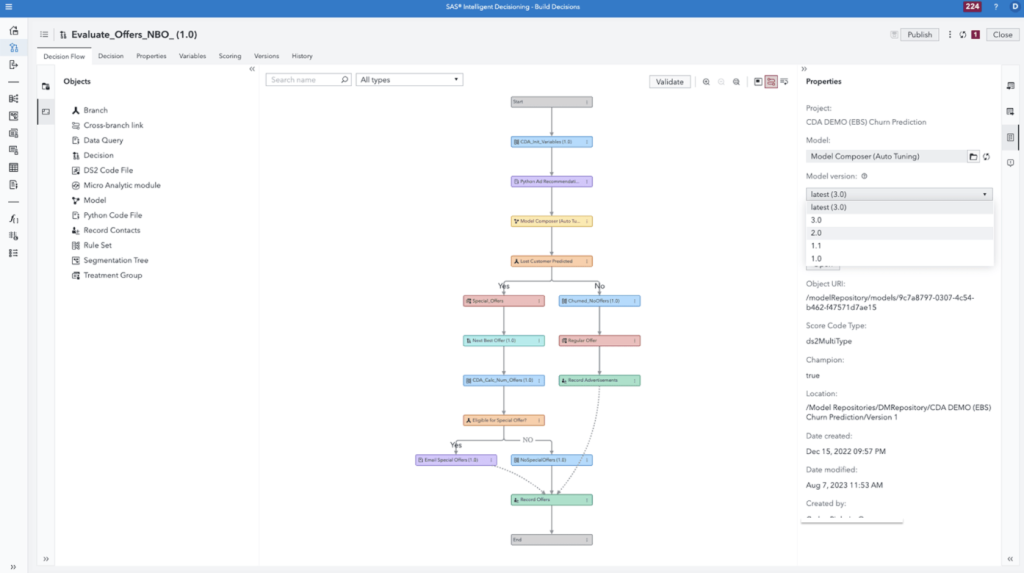
5. Is there a mechanism for incorporating user preferences or input into the decision making process?
User inputs in AI decision making systems improve accuracy and fairness by allowing users to provide real-world context and correct potential biases in the AI's training data.
For instance, in ride-sharing apps, AI algorithms factor in traffic patterns and rider demand to estimate fares and route efficiency. However, users can provide feedback on their experience, influencing future pricing and routing decisions. Social media platforms use AI to flag potentially harmful content, but human moderators decide what is removed.
Users can flag offensive content, which helps train the AI and provides human oversight. AI can analyze medical scans to identify potential abnormalities, but doctors use their expertise and knowledge of the patient's medical history to diagnose.