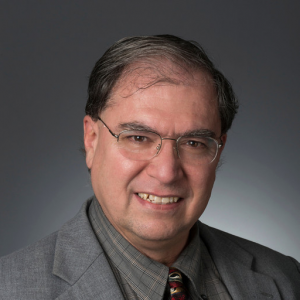
"Practical AI" might seem like an oxymoron to some. But that’s only if you view artificial intelligence as a futuristic and unrealistic pursuit. Kirk Borne, PhD, decidedly does not. Borne is the Principal Data Scientist and an Executive Advisor at global technology and consulting firm Booz Allen Hamilton.
In this interview, Borne describes a number of practical AI applications in use today and offers tips on how to deploy AI for data scientists and nontechnical users. Borne will be attending SAS Global Forum this week, and we look forward to hearing more from him there.
Let’s start by talking about what types of problems lend themselves to an AI solution. And what types of problems should not be approached with AI.
Kirk Borne: Problems that AI most easily addresses are those that are repeated and repeatable.
In other words, AI is not useful for a one-time-only solution that a human can do and be finished with faster than a machine learning model can be trained, if there even exists some training data. Those problems should be avoided, since they are not productive for an AI investment.
Thus, AI is most useful for repeated activities and repeatable tasks. This means the tasks that you want to have the same outcome every time, unaffected by human factors, such as tiredness, wrong expertise or having the wrong person on the task.
Some examples of repeatable tasks include medical diagnoses, predictive engine maintenance, customer service response, and voice/language transcription and translation.
On the more complex end of the spectrum, certain problems should not be approached with AI, such as those that require deep domain expertise, critical thinking, human empathy, and cognitive analysis that only a person can provide.
Can you describe in more detail a few practical AI applications that you see being deployed in organizations today?
Borne: Among the many practical applications of AI, two that are currently at the top of my list are applying computer vision to satellite images and applying natural language processing to assist in research for analysts.
Classification and prediction of features in satellite images can be used for agricultural applications, forestation and deforestation, climate change, coastal erosion, urban development, whale tracking, people movements like migration, and more. The algorithms are getting much better at classifying, locating, time-stamping, and providing unique identifiers in remote sensed imagery. For example, even specific whales can be identified in images of our oceans to help with animal conservation.
AI-assisted analysts, which we call Analyst 2.0 in my organization, can help manage the data flood or infoglut that analysts must ingest, digest, and analyze every day. The AI performs an initial deep information triage that classifies, labels, sorts, and ranks the incoming data and information content into manageable containers for analysts to do their most important analyses efficiently. This allows us to do more work in the same time and do it more effectively, so that more of the right work can be done.
How can companies make AI more practical and accessible?
Borne: You can’t do AI without cultural willingness, data and infrastructure readiness, the right workforce skill sets and a plan for ethics, risk and compliance.
Successful AI projects need to align with the overall mission and focus on outcomes first. AI’s transformative power is not in the technology itself but rather in the way companies use it to meet their goals. AI can’t solve all of an organization’s challenges but it can accelerate progress if it provides value, achieves goals and helps the organization deliver its services or products better.
I’ve written a lot about this recently. If you want to learn more, start with my article titled, Why culture is so important to AI adoption.
That sounds like a great read. What combination of tools and training can broaden the use of AI for nontechnical users, like citizen data scientists?
Borne: The fuel for AI algorithms is data, lots of data. The citizen data scientists need access to and understanding of the data. So, data strategies, data access rules (governance), data catalogs (metadata), and data-sharing are all needed.
The fuel for AI algorithms is data, lots of data. Click To TweetThe tools include self-service tools that require no coding to build, software to test and deploy models, databases and data repositories, visualization tools to communicate results, and platforms for sharing results and sharing conversations around results.
Training must focus on all of those technologies, but in most cases no coding is needed. That helps to broaden the use of AI across the workforce. For hardcore data scientists, coding is required and therefore training is required.
What are the biggest challenges that organizations face in deploying practical AI? What will help them move past the discovery phase to successful deployment of models into operational practice?
Borne: Challenges for deploying AI range from cultural issues to data and technology issues. Culture, technology, skill sets, data integrity, data quality, data completeness, data accessibility and technical debt are all important.
That last one, technical debt, is HUGE. It causes many organizations to avoid investing in new IT due to the costs of maintaining and carrying forward legacy IT assets. This points to another challenge: deciding who owns data science work, including AI and analytics. If the IT department currently owns it, that’s a big challenge, since the lines of business should be driving it, informing it, using it, and measuring its impact. But IT has to be involved, while also managing and maintaining so much else in the technology stack.
The best way to move from the discovery phase to a successful deployment of models and operational AI practice is to follow an agile process called DataOps. This is an iterative, fail-fast, learn-fast, agile process that creates proofs of value, including the minimal viable product and the minimum lovable product. The process involves frequent interactions between the end-users who provide requirements, the developers who build, test, and deploy the technology, and the business owners who provide metrics and evaluation.
I love that range from viable to lovable. Thanks so much for talking with us today, and we’ll see you soon at SAS Global Forum. Which sessions and activities are you most looking forward to there?
Borne: At SAS Global Forum, I am looking forward to the customer sessions, including their testimonials, applications, use cases, success stories, best practices, lessons learned, hurdles, solutions – and more.