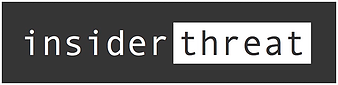
As the federal government’s ability to collect personally identifiable information has increased drastically in recent years, so have attacks targeting that information. Insider threats are of particular concern, according to the Government Accountability Office's 2015 bi-annual High Risk Report.
The GAO specifically states, “Risks to cyber assets can originate from unintentional and intentional threats. These include insider threats from disaffected or careless employees and business partners, escalating and emerging threats from around the globe, the ease of obtaining and using hacking tools, the steady advance in the sophistication of attack technology and the emergence of new and more destructive attacks.” With these trends forecast to worsen, the onus is on agencies to act now.
Fortunately, some agencies have already begun efforts to mitigate risks associated with insider attacks. For instance, the Departments of Justice and Health and Human Services have dedicated large portions of budgets for fighting cybercrime with a focus on tools that mitigate the risks of insider threats. We can also expect the newly created National Background Investigations Bureau (NBIB) to take an active role in overseeing the reform and implementation of automated insider threat detection capabilities across the federal government.
With efforts already underway to develop and implement an effective insider threat approach, agencies should be looking to include the following capabilities to augment current and future systems:
- Anomaly detection
Anomaly detection uncovers individual and aggregated abnormal patterns. By implementing anomaly detection and cluster analysis, agencies are able to gain an understanding of what constitutes baseline, normal behavior. From there, it's easy to highlight anomalies that may be indicative of malicious or negligent behavior taking place inside the network.
- Rules-based filtering
Rules filter activities and behaviors for known risks. Unlike traditional rules-based approaches, which are often plagued by false positives, an analytics-based method weighs the pertinence of possible anomalies. In other words, not all anomalies indicate something bad has happened. Rules-based filtering adds business context to sort and prioritize potential risks. This cuts down on the amount of time end-users need to spend chasing false positives.
- Predictive models
Predictive models identify emerging threats and threats never before encountered. They let the data tell the story of the unknown knowns and unknowns by applying historical data about known threats to create models that pinpoint and score new threat behaviors and patterns. This score can then be used to judge how likely it is that the instance is truly a threat. The more intelligent and learning-capable the model, the smarter, more accurate the analytical threat detection will be. This helps agencies to identify threats before they can do damage.
- Network analysis
Network analysis detects relational patterns and connections (for example, through transactions on a network). Network analysis solutions use a combination of risk scoring and automatic alert generation to notify agency personnel as soon as a new potential risk emerges. By notifying analysts of the possibility and severity of new threats as soon as they occur, investigators are able to focus their attention on the most serious issues and most likely offenders first.
Although each capability, on its own, can address a range of threat scenarios, no single solution can prevent all insider threats. Effective implementation of policy and human expertise that can evaluate alerts, will always be critical to thwarting insider threats.
To put themselves in the best position, agencies are encouraged to employ a comprehensive framework that incorporates all of the above capabilities to create a robust and extensible suite for detection, prevention and mitigation of insider threats.
If you're interested in learning more, visit us at the Insider Threat Summit, taking place March 29-30 in Monterey, CA.