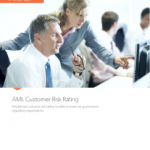
Financial institutions have been managing their AML models to meet regulatory expectations for some time. But what about customer risk rating models? We’re seeing a trend where firms are re-evaluating whether their heuristic, rules-based customer risk rating models can withstand regulatory expectations.
Rules-based models follow simple analytical formulas, such as, “If a customer is a resident of a certain country then assign them a certain number of risk points towards their overall score.” Statistical models, on the other hand, profile the data take the most relevant risk factors and apply them to them model.
Already, early adopters are finding that statistically-based customer risk rating models, especially ordinal logistic regression, offer the following benefits:
- More effectively assigns risk.
- More justifiable to the regulators.
- Easier to update, validate and maintain.
Comparing heuristic rules-based and statistical models
A heuristic, rules-based model is a simple analytical formula whereas statistically based models have an established framework that has been tested and proven in the academic space. The following table compares the differences between the two approaches.
Heuristic rules-based models | Statistical models |
Individual variable importance generally unknown. | Variable importance is known and measurable. |
Constructed by expert judgement or user intuition. | Constructed by well-established methodologies which have been tested and proven. |
No underlying methodology to follow hence there is an endless supply of model design and scoring options. | Established methodology that requires certain assumptions be met to accurately assess the modeling framework with a degree of measurable confidence. |
Difficult to validate and prove due to lack of established methodology. | Proven methodology allows for easier validation and explanation. |
Modernizing customer risk rating using statistically based models
The main issues with rules-based models are that they’re not repeatable, the effectiveness is difficult to measure and they’re more challenging to explain and defend to regulators.
Firms are moving towards analytically and statistically driven models to reduce the costs of managing rules-based models, and are finding that their development, testing and validation is more straightforward – especially given that the regulators understand and have experience with this approach.
Learn more about assessing customer risk in the paper, AML Customer Risk Rating.
1 Comment
Pingback: What do crime shows and data science have in common? - SAS Voices