Change is the only constant, and it doesn’t happen overnight. This is particularly true in the world of data analytics. As organizations are looking to become more digital, resilient and profitable, executives are going back to the whiteboard to reconsider how they’re using data and analytics to transform their business. But what should it look like? What should they be aiming for? And how will they get there?
Business, IT, data and analytics leaders might have a vision, an idea of the overall direction they want to take for their digital transformation. Still, the exact destination is something that they will probably have to refine along the way.
And that’s OK.
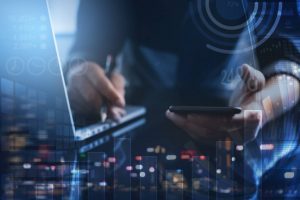
Experimentation, failure and adaptation are all part of the journey. Like when a captain sets sail to a paradise island, it will never be a straight line to get there. There will be currents, waves, storms and technical hurdles. You might not get to the exact location you had dreamed of, but it will be equally nice and warm.
When it comes to digital transformation, the paradise island is to be “data-driven,” which usually implies using AI technologies to make better decisions. But what does it mean exactly to be data-driven? Buzzwords can hide many different realities.
Regardless of specific market conditions, business priorities and technical choices, all future-fit, data-driven organizations share five characteristics.
1. Every business decision is fueled by predictive and prescriptive analytics
Data has always been a key part of decision making, even before we had computers. What changed is the sophistication of systems that we now use to extract insight from data, make predictions and inform decisions.
All the technology is here to achieve amazing things, but in reality, the use of predictive systems remains generally sporadic and experimental. There are various reasons for this: the complexity of the technical ecosystem, a lack of awareness or understanding at the board level, the multiplication of disjointed tools and technologies, and beyond the technology, a need to change the culture.
What the most successful organizations manage to do is embed data insight, predictions and recommendations as part of every decision, in all aspects of running a business. Every customer touch point, across all channels, is an opportunity to provide better personalization of services, increase loyalty, stop high-value customers from going to the competition, and offer new products and services that are the most relevant to them.
Those organizations have gone beyond making predictions and estimating the likelihood of a specific event happening. They have used that insight to determine the best course of action, effectively moving from predictive analytics to prescriptive analytics, fully integrated into the decision-making process at the front line of the business.
2. Insight-as-a-service enables real-time decision making and business process automation
The second characteristic of data-driven organizations is processing data and making decisions in real time. This is critical to provide better customer experience and deliver more excellent business outcomes. For example, when a mobile store manager can identify a high-value customer with a high retention risk and make a counteroffer immediately, before they have time to cross the street and check on the competition.
Organizations make better-informed decisions and also automate those decisions to streamline business processes. For example, they can achieve straight-through processing of insurance claims without any human intervention or to provide an immediate response to a loan application. Automated processes have the advantage of reducing the cost of running a business and providing customers with a truly digital and seamless experience.
To achieve this, future-fit organizations must have the ability to combine streaming, low-latency data from various sources (social networks, connected things) with corporate data for real-time analysis. Then they can connect insight from predictive and prescriptive models with business rules, triggering events and informing customer interactions.
3. DevOps principles are used to industrialize the end-to-end analytics life cycle
Today, most organizations are only scratching the surface of what they can achieve with analytics. The approach often remains experimental and handcrafted. Every step of the process – data preparation, modeling, deployment and monitoring – takes too much time and effort. And the experimentation doesn’t always lead to a productionalized solution.
According to IDC, organizations indicate that only 35% of analytical models are fully deployed in production. And it can take months, if not years, to deploy a new model in production.
Future-fit organizations have radically changed how they produce and deploy analytics to improve actionability, scalability and productivity, moving from a handcrafted "cottage industry” approach to a fully industrialized, highly automated system. To do this, they combine several methods and frameworks, such as DataOps and ModelOps, providing:
- Continuous integration and delivery with automated data and model pipelines.
- Formal orchestration of the development and deployment processes, with clear roles and responsibilities for effective collaboration between teams.
- Proactive monitoring of models in production to avoid pulling valuable resources in day-to-day maintenance tasks.
Underpinning this industrialized approach is a unified analytics platform, providing a trusted environment to effectively develop and deploy innovative use cases, leveraging open-source resources and skills with enterprise-ready governance.
4. High degree of data literacy achieved through democratization of data analytics
The fourth characteristic shared by future-fit organizations is a high level of data literacy, supported by self-service capabilities.
Data is becoming a product, readily available from the shelf. Data consumers can browse the catalog of available data assets and access and explore those data sets without relying on a data warehouse team. They use point-and-click user interfaces that incorporate security and privacy rules and prevent the endless replication of data sets on local databases.
The skills gap is addressed by providing low-code/no-code capabilities to complement the open-source programming approach, enabling a wider community of citizen data scientists.
“Analytics translators” provide the missing link between the business and the data science teams.
A Data and Analytics Center of Excellence proactively identifies new use cases, fosters reuse and collaboration, supports innovation and experimentation, facilitates the operationalization of models in the decisioning process, and supports skills development through training and coaching programs.
5. Proactive AI governance ensures tangible and responsible business outcomes
Every single organization has the ambition to become data-driven and use AI to innovate. Only the most innovative ones have a proactive, organized, strategic approach toward data and analytics. What does that mean in practice?
First, it means that there is a data and analytics strategy that has been formalized in a document and shared across the organization.
Second, it means that you have an organization to support the execution of that strategy. That could be a CDO, or maybe a center of excellence or steering committee. But at a minimum, some kind of governance structure spans departments and lines of business, with clear roles and responsibilities.
In the most regulated industries, such as financial services and pharma, compliance already requires lots of governance. As a result, organisations in those industries tend to be ahead of the curve. They need to leverage the great foundation they have to focus not only on compliance but also on digital innovation.
This governance framework should aim to make data and analytics more productive and actionable. Also, it should establish some ethical oversight to make sure organisations use AI technologies responsibly, with the right level of fairness, accountability, transparency and explainability.
How to fast track your digital transformation
Here are three critical recommendations to bear in mind.
Integrate your approach, from data and insights to action
Data is useless without great analytics. But similarly, analytics is useless without a trusted data foundation, and only if it’s fully deployed in the decision-making process. As a result, it is critical to embrace the entire journey from data provisioning to modeling. And to deploy analytics into the front line of the business, where actions are taken and value is created. Ensure you connect these steps and they don’t operate in isolation.
Reverse the race
Organisations often spend lots of energy in the early stages of the race to access and prepare data. When you get to the interesting part (the decisions made based on data insight), you’re exhausted. And most initiatives don’t see the finish line. Instead, run the race in reverse. Start by defining what you want to do with the data and the business outcomes you’re looking to achieve. And then work out what insight you will need to deliver those results and what data you need to create that insight. With this approach, you can prioritize efforts and avoid boiling the ocean (or the data lake), focusing on what matters.
Efforts vs. value
Typically, most organisations focus on low-value, basic data querying, reporting and dashboarding. And they spend very little time on high-value advanced analytics usages. By democratizing analytics to a broader community of users, you can make better use of the talents and skills available to you and focus your efforts on data usage that can yield more remarkable business outcomes.
Like to learn more? Watch the on-demand webinar Take Analytics to the Next Level and Future-Proof Your Organization and explore how to modernize analytics to accelerate digital transformation.