I was recently told that an organization had tried to implement AI for forecasting in supply chain but had failed due to poor data. This got me thinking about exactly what the impacts of poor data would be. And whether the approaches I had applied elsewhere could help.
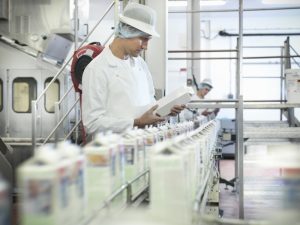
It's probably worth defining what we mean by demand forecasting, and why it's important right now. You can't miss all the articles in the media about the supply chain crisis. Certain materials are in short supply, making it hard to predict when a product can be manufactured.
With inflation rising at the same time, manufacturers can have real difficulties managing the costs of their supply chains. The traditional method of cost control was to use a “just in time” approach, where organizations held minimal stock throughout the supply chain. But with global uncertainties about availability, this approach has broken down.
Demand forecasting is one way to alleviate some of these issues. You can more accurately predict what you need, when you need it, and where it needs to be. With accurate, detailed demand forecasting, manufacturers can avoid stock-outs (if they forecast too low) and greatly increased inventory costs (if they forecast too high). This implies an agile, data-driven analytical approach. And that's what you need to respond to rapidly changing circumstances. The best example of this is in consumer demand sensing. This is a type of demand forecasting that allows organizations to rapidly pivot in reaction to sudden changes in consumer behavior.
What data do you need?
Rather than attempt to explain the mechanics of demand forecasting (see link below), I will concentrate on the data that feeds these techniques. This comes from different parts of the organization, as well as external sources:
- ERP systems, such as SAP, are the primary source of information about what has been manufactured, the products’ attributes, the hierarchies that they belong to, levels of stock and orders.
- The product reference database contains the master definition of the products’ characteristics and usually feeds the ERP system.
- Point of sale systems contain the amount of product sold to the consumer.
- Market data is usually brought in from data bureaus and contains information about market share and competitors.
- Sales and marketing records contain information about prices and which promotions you have applied to products.
You might use other source data, depending on the products. For example, you could use weather data, retail store characteristics, assortment planning or merchandising data. Where available, e-commerce systems can also have better versions of the data. This is because these systems typically cleanse data prior to use. It can contain elements such as detailed pack information and promotions.
If you would like to understand more about modern forecasting techniques using machine learning, as well as how to explain the impact of the different factors you are using in the forecasts that you generate, see this series of blog posts from my colleague Spiros Potamitis.
Where does poor data quality come from?
In theory, ERP systems should contain validated and consistent data, with checking done at the time of data entry. Unfortunately, this is not always completely the case. So what is entered into the system is subject to all the foibles of the human being doing so. Some errors are easy to spot (did you really mean to order 17 billion widgets?). But others are a lot less obvious. For example, accidentally confusing UK and US date formats and assigning a promotion to 2 July instead of 7 February.
To make matters worse, not all data starts in an ERP system. Excel is the most common source, especially in sales and marketing. Unless the organization built the spreadsheet using something like VB macros, data entry validation will be minimal at best.
Mistyping is only one aspect of poor quality. Inconsistent terminology is another issue that can be much harder to manage. With a variety of different groups providing the data, from both within and outside the organization, it is perhaps unsurprising that the same concept is described differently across disparate sources. For example, the sales team might refer to the product by its full brand name in a promotion. But the supply chain planners might abbreviate. Even something as simple as replacing “Head and Shoulders” with “Head&Shoulders” could cause a problem if systems use it as a key to join their data.
The importance of hierarchies
Hierarchies are another potential cause of problems. This is because some parts of the business typically see their data differently from others. Supply chain could look at a product in a hierarchy that focuses on where it is made and the type of materials used in manufacturing. Whereas sales and marketing could see the same product in terms of sales geography, market segment and pack size.
These hierarchies are particularly important in forecasting projects. Supply chain needs to understand the demand for a product at the lowest level so that it can be made in the right place, the right volume and the right time. Whereas sales and marketing typically needs to forecast at the product line, pack size and geography levels. This usually leads to a mapping and transformation process to provide consistent data suitable for forecasting, a process made much harder if the source data is incorrect or inconsistent.
What can be done to fix the problem?
Assessment
This will normally involve inserting some sort of data quality assessment system into the process between capture and use in demand forecasting. Data quality assessment can either be prospective or deterministic. In prospective quality assessment, you use techniques such as data profiling to identify quality issues that you have not previously encountered. This is a useful technique you should repeat regularly to ensure that new variants of poor quality haven’t appeared!
In deterministic quality assessment, you will check for issues that you have previously seen and that you know have a significant adverse impact on the results of your downstream processes. For example, when implementing forecasting that involves machine learning, missing data in your top predictors can seriously reduce the effectiveness of the model. You will therefore flag missing data in those data items and prevent potentially incorrect decisions being made.
Mitigation
There is an old adage in data quality that the closer you are to the source when you fix poor data quality the cheaper it will be. If you are capturing data in ERP systems, you should ideally spend time ensuring that data quality controls are in place at the point of entry. However, you may well find that the practicalities of changing the ERP system are too costly. So you must find a pragmatic solution.
Standardization is one technique that often has a positive impact, where you define the standard version of a value and pass the result into the analysis-ready data. This can be done in bulk for some data types (such as names, addresses, dates) and using look-ups for data that is specific to your business (for example “Head&Shoulders” or “Head and Shoulders” should always be recorded as “Head & Shoulders”).
This means you can use the values from different parts of the business to join the data. And this is critical in getting to a functional demand forecast. Standardization also allows the creation of consistent cross-department hierarchies. For example, permitting summarization across geographies and product lines would make sense to both supply chain and sales.
You can prevent critical data issues from causing catastrophic impacts by imposing quality gates on the process. For example, you might define a threshold of 85% completeness in critical values to permit the forecast to run. To implement this, you would run an automated battery of checks across your key data summarize up to a number of quality thresholds. Then you put a prerequisite on the forecast calculation process, with a notification to the accountable owner if you don't achieve the threshold.
Cultural factors
Perhaps the greatest challenge in data quality is making people understand its importance. And this can require some creative selling to persuade people to take it seriously. Unfortunately, data quality and data governance are topics that can make people switch off rapidly. So the use of anecdotes, business value cases and (in a face-to-face environment) free cookies can all play a part in getting attention. A good example that people can relate to is invaluable. And you will usually discover these when you profile data.
For example, I was once sent an invoice for a holiday booking with a date of 30 December 1899. Obviously, a default missing date. But if that had been fed into a calculation, it could have led to some serious interest charges! If you can ensure that people generating the data realize its importance, you should see an improvement in its quality and gain more accurate analytics as a result.
Conclusion
Demand forecasting projects with SAS will typically increase forecast accuracy by 10%-25% and increase revenues and gross margin by 5%-7%. You must take action to improve data quality. Otherwise, the fundamental building blocks of forecasting and AI techniques will be absent, and you won't realize the benefits. Systematic measures will alleviate many data quality issues. But you need to reinforce them with governance initiatives and clarity of ownership to truly succeed.