Organisations need to make crucial business decisions every day. The use of AI and analytical models to determine the likelihood of future events as part of that decision-making process is becoming increasingly important. In my previous blog post, I looked at the differences between building an AI model and effectively deriving insights from it. The three fundamental reasons for this chasm are that more decisions are now needed compared to before, AI models are increasingly sophisticated and decisions are increasingly complex.
For example, previously it would have been adequate to build a logistic regression model run overnight to decide whether Mr Smith would respond to a buy-one-get-one-free offer. This is no longer the case. Instead, shinier AI models have replaced the logistic regression model, including gradient boosting, neural networks, etc. And the frequency of the decision is not overnight but in real time (whilst Mr Smith shops) and often in stream. Moreover, it is not just the response to one BOGO campaign, but deciding which of five campaigns will yield the best response. Whilst we’re doing that, are we also able to check stock levels of the product we wish to sell him?
In this post, I will look at how intelligent decisioning can help solve these problems, with real-life examples.
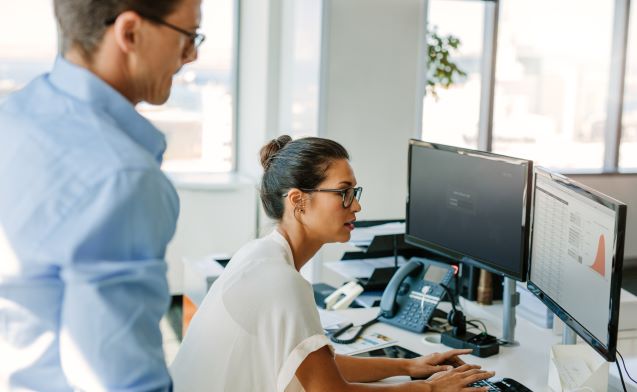
1. More decisions
There was a time not so long ago when the frequency of decisions needed to derive valuable insights for an organisation was more manageable. Consider a retailer needing to know who to target for its latest campaign. For this scenario, it could run an analytical model in batch (for example, overnight) with a file of targeted customers pushed into the marketing system.
Today a batch run decision is simply not enough. SAS worked with an insurance company to help it make a decision on whether to offer a customer a policy. When customers buy a policy, they can often choose to pay the premium in total or in instalments. Said insurer wished to amend the instalment profile depending on the riskiness of the customer.
Thus the insurer would offer a customer with a high-risk profile, with an increased likelihood of defaulting on a payment, a policy where most of the premium was due earlier, perhaps in four months instead of 12. Conversely, the company would offer a low-risk customer a premium spread over 12 months. A batch job would not provide the frequency of decisions needed for the instalment options, yet SAS was able to work with the insurer to provide policy premiums as the customer applied in real time.
2. Even more complex models
It is not just the frequency of decisions which has evolved. The types of models organizations build to feed into decisions have too. For example, previously a logistic regression model would be adequate to generate a credit risk model to ascertain the riskiness of a customer. The logistic regression would generate a value between 0 and 1 to denote the probability of the customer being "bad" and likely to default. The closer to 0, the less likely to default, the closer to 1, the more likely to default. It was achievable, though not straightforward, to put the model into a production environment and run it against real-world data to make decisions. Analysts would initially convert those probabilities into scorecard points, which is a set of business logic with points allocated for each category, e.g., age group. The second stage would require a lot of manual recoding.
The availability of more complex AI and machine learning models – such as gradient boosting, neural networks and random forest (the latter essentially creates a plethora of decision trees) – unsurprisingly creates an abundance of code. It is not as easy to interpret or understand. The same recoding process is significantly more difficult, if not impossible.
SAS is working with an insurance company that wanted to improve its risk-based pricing of policies. Using more enhanced modelling techniques, such as gradient boosting (see my previous post for details), the company increased its model accuracy. However, there was an issue using it with the existing decisioning engine. By leveraging intelligent decisioning, the company was able to run an advanced algorithm and provide more accurate pricing, leading to a 1% reduction in loss ratio.
3. More, more, more
Sometimes straightforward decisions are adequate – should a loan be approved or not? And you can use simple rules, along with an analytical model, to make that decision. Increasingly, though, these decisions are getting more complex. For example, when deciding on a loan application, you may decide to apply different AI models depending on which segment the applicant falls into. More complicated decisions, combined with the need for more complex models and faster decisions, mean times have changed.
Becoming a supermodel with intelligent decisioning
This post has highlighted some examples of what many organisations struggle with – incorporating complex analytical and AI models with business rules in an operational system to drive timely decisions.
Intelligent decisioning gives you the ability to integrate AI models, both SAS and open source, with business rules. You can build, test and deploy models and business rules all within the same interface. The deployment process is simplified. Furthermore, it gives you the ability to understand the impact of any changes prior to deployment into the operational world.
Intelligent decisioning can help organisations that struggle with incorporating complex analytical and AI models with business rules in an operational system to drive timely decisions. Click To TweetFor example, SAS worked with a major global bank to help close the chasm between models and decisions. The bank was able to complete the entire end-to-end process – from data, to the latest AI models, to decisioning – to make the optimum loan application decision swiftly.
The bank was able to make use of complex AI models and apply multifaceted rules, increasing the precision of the application decision. And it could support all these decisions with auditing capabilities. This is important because banks need to be able to demonstrate how they have made a decision to accept or reject a loan application.
A unified platform
Intelligent decisioning can meet these needs with a unified platform that allows access to a multitude of data sources to build clever AI models and make optimised decisions quickly all within one environment. And since organisations are increasingly making use of both proprietary and open source models, the platform also allows you to get those important decisions irrespective of what language the models have been built in.
At SAS, we’re partnering with companies across Europe to help them realise the benefits of intelligent decisioning. To learn more, please visit our website.