Combining analytical resources and expertise from across the organisation is a prerequisite for effective, well-informed decisioning. How can your bank break down analytics silos and inspire a culture of collaboration? Organisational silos are holding banks back from driving innovation and meeting customer demands. When your customers expect convenient, personalised and ultraresponsive services, any delays in decision making can lead to damaging publicity on social media or even customer attrition.
For most banks, the story is the same. Legacy systems and inefficient processes built around traditional analytics functions such as fraud prevention, credit risk scoring and marketing tend to reinforce silos within the organisation. The key to bringing different teams and departments together is to establish a central analytics function that can play the role of the brain in your organisation and drive more effective decision making companywide.
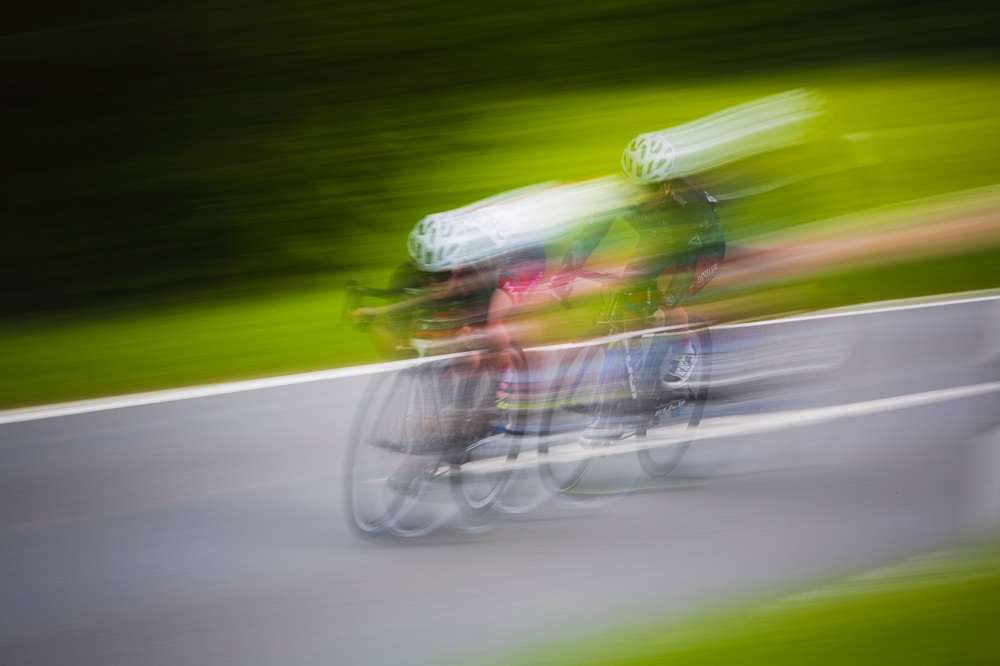
Turning data into action
Creating a single enterprise analytics platform requires much more than just aggregating and storing data in one place. This aggregated data, and also the analytical results, need to be deployed at the point of decision making so that organisations can use it to take appropriate action. Much to their detriment, many banks and other large businesses still struggle to fill the operational gap between storing and deploying data across multiple departments.
That difference is illustrated in a blog post by my colleague Gerhard Svolba, where he tells the story of Mrs Cerny, a small greengrocer operating on a residential street. Mrs Cerny understood her customers well. She knew which of her neighbours were living on a tight budget, and which had more expendable income. Collecting and analysing this type of information about customers is a perfect example of how organisations store data. At this stage, it has the potential to yield positive results, but the organisation is not actually leveraging it.
When Mrs Cerny acts on this information to provide personalised offers to each of her customers, she is deploying her insights. By offering wealthier customers more expensive products she is increasing sales revenue per item sold. And by offering better deals to savers she encourages them to frequently visit her store. For Mrs Cerny, deploying her data achieves the specific purpose of her analysis: maximising her sales revenue over the long term.
A central hub
Before the advent of the internet and mobile technology, it made sense for banks to store important analytical information, such as credit risk scores and marketing data, for other internal teams to use at a later date. Back then, banks simply didn’t need to act fast. But today, with more competitors entering the market and offering customers near-real-time decisions on loans and mortgages via mobile apps, the ability to make timely, accurate and reliable decisions has become much more important than ever before.
Establishing a central hub for analytics
Before you decide which technology to use to build a unified enterprise analytics platform, we recommend evaluating different solutions according to three key criteria: enterprise usability, openness and integrability.
1. Enterprise usability
For an enterprise analytics platform to work well within your company, every department in your organisation needs to be able to use it for its own unique purposes. Any platform that you implement must be flexible enough to meet the niche needs of different departments.
Many banks and other large businesses still struggle to fill the operational gap between storing and deploying data across multiple departments. Click To TweetSiloed analytics capabilities severely limit an organisation’s ability to foster collaboration between different departments. This is because the data, systems and processes used by separate teams are often incompatible or too time-consuming to aggregate. By contrast, a flexible enterprise analytics platform can help organisations stay agile even when internal restructuring occurs. This flexibility enables banks and financial institutions to adapt their businesses in the face of market changes without having to invest a huge amount of time and resources in integrating siloed systems.
2. Openness
Even the most flexible enterprise analytics platform might not be able to accommodate all of the unique, highly specialised functions that the analysts from various departments need to perform. This is why it is essential that any analytics solutions you deploy can work well with solutions from other vendors. This includes both open source and proprietary technology.
With an open analytics platform, organisations can keep the total cost of ownership of their analytics environment low by harnessing open source technology and avoiding vendor lock-in. Ensuring openness will also enable organisations to evolve their enterprise analytics platforms more quickly and cost-effectively in the future.
3. Integrability
The enterprise analytics platform that you select for your analytical needs is only one part of the picture. Any solutions that you implement must also integrate with your internal systems, data and processes. That includes your credit scoring and segmentation models, credit policy and fraud identification rules. And also the various technology and IT systems used by different departments.
You must ensure that your enterprise analytics tools can integrate seamlessly with ancillary processes and fit neatly within the larger enterprise IT ecosystem. This enables your entire analytics division to run like clockwork, helping to reduce the latency between discovering new insights and acting upon them strategically. With an efficient, centralised analytics hub, businesses can spend more time improving their products and services.
Opening new opportunities
Once banks and financial institutions have developed a core enterprise analytics platform, they are then much better placed to explore the potential value that other technologies – such as artificial intelligence (AI) and machine learning – can deliver.
Banks that have been through the process of developing a core analytics platform are already beginning to see the benefits of integrating decisioning systems powered by machine learning and AI to improve the customer experience. Equipped with accessible, reliable and timely analytics tools, departments across your organisation will be much better placed to collaborate and develop next-generation products and services. And that helps your bank keep pace with evolving customer expectations and defend against market disruption.
What can you do next?
If you would like to discuss any of the topics raised in this blog post, please do get in touch on LinkedIn or via email at ashutosh.kumar@sas.com.