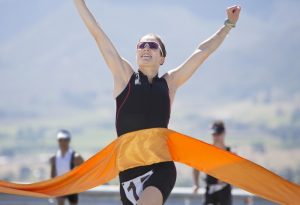
As more and more organisations embrace advanced analytics, we are starting to see several very successful implementations emerging, on both a small and large scale. At the same time, however, many organisations are not getting value from their expensive investments in analytics systems. And while failing may provide useful lessons, it is probably better to learn before failure. By comparing the two groups, it is possible to draw some conclusions about what makes analytics projects more likely to succeed or fail.
There are four main areas that top teams and managers should pay attention to if they want to succeed with their advanced analytics projects.
1. Strategic engagement and understanding
It goes without saying that top managers need to be behind any analytics investment, but it is not enough for them simply to decide that they want the organisation to use advanced analytics or declare that it is now data-driven. Any kind of analytics project must be set within a clear vision for the company’s future, business direction and goals.
Managers must also be clear about the future of the analytics project, and how it will be scaled up and rolled out further. Without this clarity, it is likely to remain a pilot forever, albeit perhaps a useful one in its small context.
Linked to this, and part of strategic understanding, is that businesses also need to be clear about how the new analytics project will fit with existing structures and systems, both organisational and IT – such as legacy systems. This does not mean that it is essential to upgrade all legacy systems before any investment – quite the reverse, in fact. It simply means being clear about what needs to interact with what, and how this will be made to happen. Having one platform to manage the entire analytics life cycle can help with consistency, collaboration and governance.
2. A clear and relentless focus on value generation
Many companies fail to generate value from their analytics investment because they focus on the wrong problems or projects. It is essential that major investments, such as in analytics systems, are focused where they can add most value, most quickly. In other words, potential use cases should be carefully assessed to make sure that they are focused on strategic issues and big problems. A focus on value generation also means measuring outcomes and results to ensure that your investment is delivering.
This may sound theoretical, but you should also do the least possible work to generate value. For example, having clean, high-quality data is essential to generate useful insights with analytics. However, you probably do not need to clean all your data – just what you need at any given time. Clean what you need, when you need it – but make sure that the clean data is available for future work too.
Failing may provide useful lessons, but it is probably better to learn before failure. 4 tactics to make #analytics projects likely to succeed. Click To Tweet3. Clarity about internal roles and tasks, and the skills needed to deliver
There are several aspects to this, but they all relate to internal issues and management. The first is to have clarity about need, and how analytics will fit within your organisation. It is all very well deciding to recruit data scientists, but are you sure you know what they are going to do? And are you really sure that data scientists are what you need?
Rather than focusing on job titles, it is better to be clear about the tasks that need doing, and the skills required to do those. This will include having people who can “translate” between business users and analysts – whether part of either group or not. Someone needs to be responsible for ensuring that business needs are heard and understood, and that analytics projects are delivering to business requirements.
4. A clear view of the wider context
You might consider the wider context is part of the overall strategic view, but it is worth mentioning separately. There are an enormous number of things that can be done with advanced analytics, but some of them may not be legal within your regulatory context. The General Data Protection Regulation (GDPR), for example, requires executives to be able to explain the basis of decisions about individuals. This means that “black box” algorithms are likely to be inadvisable for companies that might be affected. “Computer says no” will not be enough, so you need to be confident that your analytics output is clearer than that. GDPR also means that it is likely to be better to use anonymised data, so that you do not have to change everything each time someone asks to be forgotten. Forgetting the regulatory context could be an expensive mistake.
Empowering stakeholders
Analytics success impacts a broadening spectrum of stakeholders. As analytics focus shifts from point-applications to enterprise-wide capability, decision management becomes more effective. Join our #saschat discussion on 7th December, kicking off at 15hrs CET, 14hrs UK and 9hrs ET, to explore these points:
- How do you define 'enterprise-wide analytics'?
- What are your favourite use case examples of enterprise wide analytics?
- How does the analytics platform support enterprise wide analytics?
- What are the most important attributes of an analytics platform?
- What are the biggest organisational or cultural issues to navigate for effective enterprise-wide analytics success?
- How are more mature users demonstrating benefits of enterprise wide analytics?
2 Comments
Combine with covert and overt intelligence asset, tasked and co-ordinated effectively can transform performance. It’s not either or.
Great blog! I see point #1 as being about the alignment between analytics strategy and business strategy. It’s where we create the analytics vision, map analytical capabilities to business goals or objectives and define the analytics platform. Point #2 is about priorities, delivering use cases and getting some bang for the buck. In point #3 we start to explore the topic of operating model – processes, roles and organisational structures. Point #4 is a refreshing perspective as it gets us to step outside the box and consider the wider implications. This is a very practical approach for success in analytics.