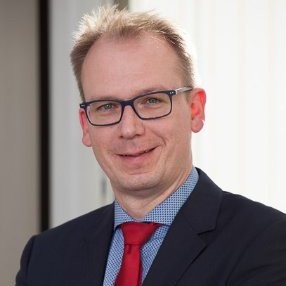
Whether we talk about improving customer experience, applying chat bots, preventing fraud, realising IoT applications like predictive maintenance, implementing credit scoring or claims management, or just automating internal processes, analytics and eventually AI will have a profound impact on these large and small decisions. Across all industries the discipline of ‘decision management’ is seeing explosive change. It is also a topic that seems to defy consistent definition, especially with organisations progressing with analytics with varying levels of maturity. Andreas Becks is our expert on the re-imagination of decision management, so ten minutes with him seemed like a good idea.
Surely enterprise decision management has already been automated with the arrival of computerisation?
Yes it has. But the integration of analytics, and especially the coming learning algorithms, will move things to a completely different level. Operational decisions based on the combination of analytics (or machine learning, if you will), organisational business rules, and integrated decision logic has proven to be more relevant, faster and more valuable. For example, a European telco provider has implemented automated next best customer actions to make offers in the context of recent customer activity using an integrated decision management framework. A major international airline implemented decision management technology to offer their customers truly personalised products and services as a complete and exciting passenger experience.
How long does it take to implement decision management technology to offer customers truly personalised products and services? @becks_andreas on #DecisionManagement Click To TweetThese look complicated to set-up. How long does it normally take to build such analytical decision rules?
Faster time to delivery is one of the strengths of an integrated (or platform-driven?) approach. Models and business rules can be deployed to data ‘at rest’ or ‘data in motion’ within the customers’ operative decision-making processes by the push of a button rather than through manual or semi-automatic re-coding and code staging. This significantly lowers time-to-market and prevents the analytical result from becoming obsolete before they can be utilised. When data changes over time, models can quickly be adapted to stay relevant and performing
Usually fast also means expensive.
Not with a robust analytics platform. Process costs are significantly lowered due to automatization while at the same time sources of errors are reduced. Models can be refined and executed without manual coding. All process steps regarding data, models, and rules are transparently documented, and rule execution is centrally administered. This leads to error reduction, less reliance on key employees and, finally, significantly reduces overall IT costs.
But what about model accuracy?
We have thought of that too. Models, they need to be ‘fresh’ to be most effective. Technically speaking, the patterns in the data that models have been adapted to need to be relevant. Because this may change over time, quicker time to deployment and model application leads to more accuracy. Moreover, better model monitoring (with automatically generated reports) ensures that the need for retraining and model improvement can be detected earlier. Automatic retraining or alerts for the data scientist close the loop in the analytical lifecycle and lead to more sustainability of models and, thus, to better decisions.
This is all fabulous for the data science community. But how does the proposed approach to decision management make it into business workflows?
Better analytical effectiveness has been at the core of our thinking. The ability to combine analytics and business rules helps customers to design and execute complex decision flows. Deployment of analytical models and business rules via a centrally governed analytics platform provides control over how analytics is utilised. Stakeholders should ask for a decision management platform that is an open environment where models and business rules can be automatically deployed to any destination (in-database, in-stream, in-device), and monitored in an automated and personalised flow. Automated deployment, improved monitoring/auditing tools, transparency and flexibility will have a significant impact on the efficiency of the business.
How does this enable trust in the algorithms?
You are right, trust has become a major factor. At any given point in time it is transparent which decision was drawn based on which rules, on what data and what version of a model. Customers, therefore, have their audit trails ready and can apply governance to automatic decision making.
So the key enablers for better decisions are are speed, accuracy and governance?
Yes, and more. You also need scalability, readiness for AI and a package that integrates all that matters. Many software tools, including the growing spectrum of open source ones, offer the ability for data scientists to build models, but there are not many that allow them to share and deploy them. Organisations are struggling with how to automate more of the steps of the analytical lifecycle to relieve the burden on their staff and reduce time to value for their investments in analytics. Realising that the business value rests on making better decisions faster should be the credo. Customers should demand that their analytics platform helps them harvest the value of efficient decision making.