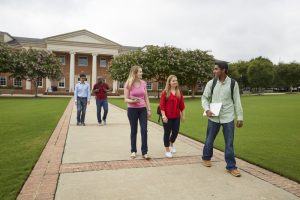
The SAS Data Science and Advanced Analytics Forum brings together businesses and universities, and at a recent event, universities described courses that focused on providing students with technical skills and competencies to handle data, together with some understanding of how data scientists use software. Businesses, however, emphasised the importance of data scientists understanding business areas.
There is, therefore, something of a mismatch between the way data science is taught in universities, and the needs of businesses employing data scientists. As a recent graduate now working as a data scientist, this is a subject close to my heart, so I was excited to join a #saschat on this very subject, along with other recent graduates and some data science students.
The discussion was illuminating, exposing as it did some gaps in expectations, and also some ideas for addressing these.
Defining expectations
The answers to the very first question confirmed the gap in expectations. Students and academics stressed the importance of understanding and mastering technical skills. Those working in business stressed the importance of “real-world” skills and experience, for example, having been exposed to genuine poor-quality data, rather than carefully prepared samples for academic work.
Having recently started work at SAS, I can see both sides of this question. Some understanding of business is important for data scientists, as is the concept of real-world situations versus the ideal of the ivory tower.
But without the basic technical skills, you cannot put yourself forward for work, whether an internship or a job. I think those who have been working for a while may tend to forget this need for basic skills. All the data scientists they see have those skills, so it is hard to remember that they must be learned somewhere. Data science is, however, not routinely taught in schools, and not often in undergraduate courses. Master’s courses, therefore, have to spend time on those technical skills to give their students a chance to get a foot in the door. To be able to attract the widest range of potential students, they need to provide a solid grounding in data science skills, rather than assuming knowledge. That must be the basis of any data science course, whether undergraduate or graduate.
Taking that as the basic starting point, then, what can be done to help universities ensure that data science courses successfully bridge the gap between academia and business, and turn out students who are prepared for work?
What can be done to help universities ensure that data science courses successfully bridge the gap between academia and business? #DataScience Click To TweetIntroducing real life to academia
Participants in the #saschat had plenty of ideas to address this. First and foremost, there was general agreement that data science courses should use real-world data to introduce students early on to the idea that data sets are not perfect. Master classes from industry specialists would also help to bridge the gap, just as many business schools make a point of employing people who are actively working in industry and management, and draw on case studies to emphasise the application of theory to practice. This requires universities to work more closely with organisations and companies employing data scientists, but I think the business school model would repay further examination.
There is another issue, however. I think there must be some onus on students themselves to keep up to date with developments in the field. It cannot only be about what universities should teach. Employers and businesses like SAS put a lot of effort into trying to help students bridge the gap between academia and work, including through building communities and setting up mentoring schemes. There are a range of opportunities both formal and informal, including meetups, mentoring, MOOCs, hackathons and hands-on experience via internships. Students need to grasp these opportunities with both hands.
A question of focus?
There is, of course, a question about what is really needed for data science students. Data science is very prone to cycles of hype, and it can be easy to get sucked into new ideas, and potentially waste time learning something that turns out to be a blind alley.
But is it really a waste of time? I would argue not. Taking the occasional wrong turn is part of working life, and learning that early on is likely to be very useful. Time spent learning a new skill is also unlikely to be completely wasted, even if you never use that skill. Detours and diversions are important in both life and learning, and few experiences are totally wasted. An open mind might turn out to be the most important qualification for working life.