In recent posts (March 26, April 21) we've looked at forecasting in the face of chaos and disruption. We've seen that traditional time series forecasting methods (used during "normal" times) can be creatively augmented with additional methods like clustering, similarity analysis, epidemiologic models, and simulation.
While it is unreasonable to expect highly accurate forecasts in these chaotic circumstances, our objective is to identify a range of plausible (and likely) outcomes, so we can make better decisions.
For example, with no changes in human behavior in the midst of a worldwide viral outbreak, there were plausible projections of an overwhelmed health care system. Government issued "stay at home" orders are a decision based on projected outcomes.
In business we use forecasts in the same way -- to decide what actions to take in the face of uncertainty. It may be a decision on how much to produce, or how much to keep in inventory. Or, we may want to change behavior, so we decide to run a promotion to increase demand.
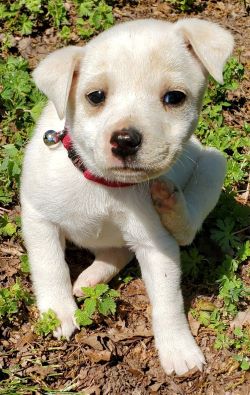
Forecasting During Denial
Beyond chaos and disruption, we also face another forecasting challenge: How do you forecast in the face of denial -- when management can't handle the truth?
I suspect most forecasters have been in this situation. Using the most appropriate practices learned in your scientific training you've prepared the data, run the analysis, generated your forecasts, and delivered the results.
But then management doesn't like it. The one person you have to convince is either unconvinced by your science, or the message your science-based forecast conveys is not the message that person wants to convey.
For example, suppose there is extremely high demand for your product, yet you lack the capacity to supply it either now or in the foreseeable future. Since the forecast should represent an "unbiased best guess at what is really going to happen," the forecast should be that you won't be able to fulfill the demand. Yet your manager doesn't like that message and wants to assert "anybody that wants the product can get the product."
Is there anything you can do?
There is, but only by applying more science -- Forecast Value Added analysis.
By comparing the treatment (management's override) to the placebo (your forecast), you can determine whether management's override has an effect (good or bad) on forecast accuracy, or perhaps no distinguishable effect at all.
Of course, if your manager rejects science, even FVA won't help!