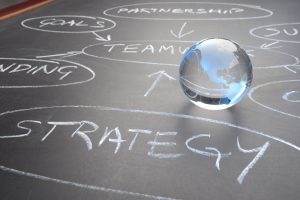
As your organization works to recover from the uncertainties and far-reaching implications of the COVID-19 pandemic, it’s important to ensure your business is resilient and can adapt quickly to changeable conditions. One way to create resilience is to connect data to decisions by creating a data analytics strategy that clearly links people, processes, technologies and data. Think of the analytics strategy as your north star – and your data strategy as the supporting framework.
How an analytics strategy works
An analytics strategy is designed to improve all the ways you design, deploy, monitor and govern analytics. It establishes the infrastructure, plumbing, processes and people needed to deliver the right analytics at the right time across your organization. Your analytics strategy should answer key questions related to:
- Business goals that analytics can inform.
- Measurements that will show you’ve achieved the goals.
- Data that’s available (or could be available) to support analytics.
- Infrastructure, or the multifaceted components needed to achieve your desired results.
- Insights, which involves determining how, when and where to deploy your insights to support decisioning processes.
Your organization can benefit from a data analytics strategy in four main ways.
- Your team can stay focused on high-value activities that lead to business results because you’ll have analytic models that are clearly aligned with specific business issues.
- You won’t have to rework or throw away models because you’ll know what it takes to deploy models into your decisioning processes.
- You can retrain or replace models quickly so they’ll continue to perform, because you’ll employ mechanisms to govern and monitor your analytics. You’ll also know when models have outlived their usefulness and need to be retired.
- You can focus on high-value business decisions that demonstrate ROI from analytics initiatives because you've defined key metrics to capture the business impact.
How a data strategy supports analytics to create resilience
A data strategy is the foundation for creating resilient analytic processes for your organization. The data strategy is vital because analytics relies on data for decisioning processes. The goal of the data strategy is to define how data is acquired, stored, managed, shared and used for analytics and decision making. Think of the data strategy as your road map for ensuring the right data is in the right place, at the right time.
The data strategy needs to address details around how data will support the analytics strategy. This type of strategy ensures that data will be identified, stored and provisioned in a way that both analytic and business communities can readily understand and use to expedite analytic and decisioning processes.
The data must be integrated in a consistent and cohesive way that adheres to governance policies and procedures. And the policies, procedures, data definitions and lineage surrounding the data should be transparent to data and analytics users. In turn, they’ll know that the correct data is being used in analytics to support data-driven decisions.
A solid data strategy builds trust because it ensures that the data being used for analytics is fit for its purpose. It builds confidence because it defines criteria for making sure the data can be easily explained and is transparent, defendable and justifiable for the business processes in which it’s used.
The role of DataOps and ModelOps
So, how do we execute on our strategies and build flexibility into our processes while reducing friction? This is where DataOps and ModelOps come into the picture. Creating these fundamental capabilities gives a solid foundation to your analytics, so you can scale and accelerate your efforts and be prepared to use ModelOps and DataOps effectively. What do we mean by these terms?
- DataOps focuses on shortening data cycle times and improving data quality – which includes sourcing and delivering data.
- ModelOps is focused on accelerating model building and deployment, focusing primarily on the work done to deploy models.
The goal of both efforts is to increase the capacity of analytics while drastically reducing the time it takes to go from concept to model deployment. The objective is to improve the usability of the models that are created. This works best when models are trusted. And models are more trustworthy when they’re built on high-quality data that’s governed well and managed by a data strategy. Such models will continue to perform well through monitoring and alerting.
These are all iterative processes. As data quality degrades, or when models no longer perform – or when the business situation changes – you’ll need to cycle back through the process. Keep in mind that there are no clear lines between DataOps and ModelOps. Because they are so closely aligned, the division between the ”ops” can be blurry. They also depend on your organization’s structure and culture.
Embracing “Ops” requires a shift in mindset
Speaking of culture, “ops” involves people, processes and enabling technology across various departments in your organization. Being resilient means breaking down those barriers and removing the silos they create. To succeed, you’ll need to focus on changing the culture and keeping everyone aligned to the same goals – which are focused on the what’s best for the company as opposed to an individual department.
This shift in mindset not only helps to eliminate silos – it also helps prevent situations where data or models are thrown over the wall without a thought as to the impact. A cultural change creates empathy between teams – including those focused on business, data, analytics and IT. It brings people together throughout the entire data-to-decision process.
When combined with a unified data analytics strategy, DataOps and ModelOps give you the capacity to create resilient analytics results. This type of strategy helps you readily adapt, focus on high-value questions, quickly deploy high-performing and trusted analytics into production, and have confidence in the decisions being made. These qualities are more valuable than ever as business work to recover from disruption and remain resilient.
Download an e-book about operationalizing analytics