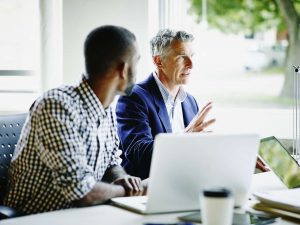
Analytics in the big data era means more data to feed models, more data points to correlate and more insights to uncover. But without detailed knowledge about the data analyzed, can the analytical results be trusted? This is why lineage is an essential element of data management for analytics. Lineage involves knowing not just where data came from but also whether it came directly from a source or passed through a process, system or application before analysis. While data quality issues can definitely skew analytical results, it’s also possible data could be excluded, intentionally or accidentally, from analysis. This can be an issue when analytics is driving business decisions such as determining funding, assessing risk, calculating liability or awarding claims.
With the rapid adoption of data analytics throughout the enterprise, data flows in many directions across the business – this makes it difficult to understand where the data was created and how it got there. With data lakes being increasingly used to centralize enterprise information – including data that originates from a variety of internal and external sources – the importance of data lineage when managing data for analytics is growing.
To get the full technical functionality and business value from data, lineage must include multiple forms of metadata, which provides a definition, description or context for the data. Technical metadata provides the language that applications and their proprietary interfaces use to describe data and its structure. Business metadata provides the glossary of human language descriptions of data that business people can understand. Operational metadata provides details such as source, copies and movement. This is important in today’s hybrid data ecosystems where data moves around a lot in multi-platform environments, from landing and staging to sandboxes to analytics tools, and then out to reports or to load target databases such as a data warehouse. Further, operational metadata assists when governance requires a record of who (or what tool) accessed which data and when. When data preparation is properly performed, all of this metadata is centrally cataloged and readily available for data lineage.
Analytics enables data-driven organizations to make better decisions and produce better outcomes. Before you get dazzled by data visualizations or seduced by statistical algorithms, make sure you learn the lineage of the data that fed the analysis. By providing an audit trail, lineage is key to post-decision defensibility and post-outcome accountability – increasingly necessary for regulatory compliance.
Download a TDWI Checklist Report on becoming data-driven