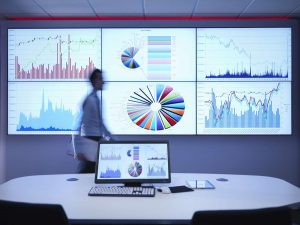
There's no doubt that analytics applications are rapidly becoming a necessity for an organization to establish any kind of competitive advantage. Yet this statement implies a paradox – because if all organizations are implementing analytics and integrating the results into their business frameworks, then doesn't that level the playing field? If everyone is doing it, how can it provide a competitive advantage? The answer may lie in the data management strategy for analytics – that is, the strategy the organization uses to design, develop and implement analytics.
Anyone can install an analytics tool suite, and even unsophisticated data analysts can use out-of-the-box analytical models to derive some business benefit. More knowledgeable data scientists might be able to develop analytical models that exploit more advanced algorithms. Yet again, the use of the algorithms does not establish the competitive advantage.
Balancing the data management strategy for analytics
A competitive advantage can only be unleashed as a result of having a holistic awareness about the entire extended information enterprise and developing a strategic approach to data management for analytics. This strategic approach balances all facets of the analytics process, including data architecture, data policy, analytics sophistication, problem-solving and systemic data awareness.
Data architecture
When I say data architecture, I mean a comprehensive approach to data organization. This goes beyond developing data models for data warehouses, or introducing data catalogs for data lakes. The data architecture includes these, and more – it encompasses all aspects of organizing and managing the ingestion, collection, storage, management, integration, quality and accessibility to data assets that are to be consumed for analytical purposes. The data architecture scopes how access to the right data for specific purposes is easily facilitated while ensuring the contextual data quality.
Data policy
A data policy specifies the defined guidelines for ensuring the usability of data assets for analytics that observe regulations, internal policies and other data obligations. Data quality assurance is just one part of data policy. Other aspects include:
- Assessment of data sensitivity and subsequent classification.
- Compliance with externally imposed restrictions for use (such as limitations on sharing data for users under the age of 13, or privacy restrictions imposed as part of the EU GDPR).
- Communication of data obligations to data sharing partners.
- Enforcement of similar data obligations conveyed with acquired/shared data.
Analytics sophistication
Analytics sophistication refers to having the right mix of analytical approaches and algorithmic techniques to accomplish the business needs. Having an arsenal of analytical tools is one thing – but knowing which technique to use in certain circumstances, as well as being aware of the limitations of each technique’s utility, is necessary to get the optimal results.
Problem-solving
One aspect of a data management strategy for analytics that is sometimes ignored is problem-solving. This entails processes for engaging business stakeholders, understanding their most critical challenges, identifying what data sets are needed to solve the problem, determining what data is and is not available, and finding the data sets that are not available as a prelude to developing an analytical solution. Many analysts rush to apply their tools before truly understanding what challenge they're trying to overcome. Problem-solving provides the context, reduces the complexity and streamlines the development of analytical solutions.
Data awareness
Finally, data awareness ensures that all individuals involved in analytics processes are familiar with and agreeable to abiding by their responsibilities for data usability. This includes harmonizing data semantics, abiding by data policies, and working together to enforce compliance with enterprise-wide data quality expectations.
A data management strategy for analytics can be challenging, especially at organizations accustomed to siloed business functions and specialized transactional or operational systems. An organization can't convert to being analytics-aware overnight, and attempts to jump-start the process without engineering the appropriate architecture will lead to frustration later on. Counter these issues by developing a data management strategy that simplifies the adoption of advanced analytics.
Find out how the SAS Platform supports data management for analytics