In two previous posts (Part 1 and Part 2), I explored some of the challenges of managing data beyond enterprise boundaries. These posts focused on issues around managing and governing extra-enterprise data. Let’s focus a bit on one specific challenge now – satisfying the need for business users to rapidly ingest new data sources.
Sophisticated business users recognize the potential for extracting value from different types of externally sourced data. But those data sets are configured in many different ways. In some cases, the data appears in a traditional structured format, such as collections of records with comma-separated values. Other times the data is free-form, like text in emails or other documents. Sometimes there are structural hints embedded in unstructured data – for example, when character strings with hashtags are embedded in small messages sent via social media. And sometimes data sources combine text, images, video, etc. These are even more complex from a structural perspective.
Whenever a data source is identified as having some value to the business, you need to work quickly. Because you have to figure out what structure it has (if any), what valuable information it carries, the best way to capture the information, and how fast the data can be absorbed.
In some cases, the data source can be subjected to what might be deemed a modified data profile. While profiling has been used to scan data sets and assess suitability for use, adaptations to that approach take a different route. Adaptations may blend traditional statistical analysis with analytical utilities such as text analytics, data value imputation (that is, replacing missing data with statistically valid values) and other data manipulation techniques. Blended techniques provide a broader approach to data preparation than traditional methods.
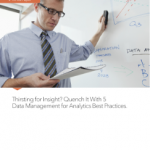
But what really distinguishes these data preparation tools is that they are meant for business users, not IT staff. Data preparation tools give end users access to raw data. They give business users more say in interpreting semantic structure and meaning based on their expectations. At the same time, this direct exposure to raw data demonstrates the “glitches” in the data that would have traditionally hampered the IT team’s ability to ingest and integrate the data sets. The result of using such data preparation tools is that business users can communicate more effectively to data practitioners when they describe which standardizations and transformations need to be performed.
In other words, user-oriented data preparation tools engage business users, encourage conversations between business experts and IT teams, and speed the process of developing applications for data ingestion and integration. This reflects a core tenet of the agile development approach: increased collaboration between IT and business experts. An implication is that sophisticated data management technologies are necessary for speeding data ingestion. Ultimately, this approach reduces the it takes to make external data available for use in analytics.