In this blog series, I am exploring if it’s wise to crowdsource data improvement, and if the power of the crowd can enable organizations to incorporate better enterprise data quality practices.
In Part 1, I provided a high-level definition of crowdsourcing and explained that while it can be applied to a wide range of projects and activities, applying crowdsourcing to data improvement involves three aspects: type of data, kind of crowd, and form of improvement. Part 2 focuses on type of data and kind of crowd.
Type of data
Crowdsourcing is limited to data the crowd can access, typically via the Internet. You can remember this concept with the phrase: crowds use clouds. And since most often it will be a public cloud, use this as a general guideline: data you would not put on a public cloud should not be outsourced to a crowd.
Some enterprise data will therefore not be suited for outsourcing to an external crowd. An internal crowd of employees can be afforded more data access, but security and privacy issues will still prevent sensitive data from being crowdsourced (e.g., customer data containing personal financial information or patient data containing personal health information). However, the less sensitive aspects (i.e., non-personally-identifiable information) of sensitive data can be crowdsourced, as long as the sensitive aspects are obscured or omitted.
One example of data that’s ripe for crowdsourcing is paper-based forms filled out by hand. In this case, crowdsourcing provides low-cost outsourcing of data entry. For example, last week as part of my research for this post I spent one hour on Mechanical Turk entering data, for which I was paid $0.69. It’s worth noting that all of the scanned forms provided for my data entry obscured sensitive information (e.g., social security number).
Kind of crowd
After you decide what data to crowdsource, the next challenge is crowd composition, which is more complicated than external versus internal. There’s also debates about experts versus novices in internal crowds, and the danger of relying on the unknown knowledge, skills, and experience of anonymous amateurs in external crowds.
Though the era of big data has made the crowd a seamless part of our everyday experience (e.g., Facebook likes, up/down votes on Reddit, five-star ratings on Netflix), enterprise data management seems like it should be left to subject matter experts, and domain expertise is occasionally an absolute necessity.
However, crowdsourcing opponents often criticize the crowd’s unknown (or lack of) qualifications while leaving experts unchallenged, assuming they’re better because they’re experts. It’s easy to say experts will outperform the crowd, but crowdsourcing can prove whether that’s true. Many studies across numerous disciplines have shown the aggregate of a large, and largely amateur, crowd often meets, and sometimes exceeds, expert performance.
Comparing how often an individual agrees with the rest of the crowd, and how often the crowd agrees with the experts, can be used to establish
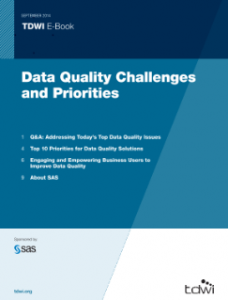
performance benchmarks and identify the best individual contributors, internally and externally. Testing the performance of the crowd (regardless of composition) also provides the added advantages of helping the enterprise identify biases and preferences and overcome not-so-humble opinions.
One last note about expertise. Experts are those closest to data’s source or subject, which sometimes is beyond the walls of the organization and beyond the
direct experience of its employees. Examples include international postal address verification and multilingual representations of products and services. While enterprises often have a global reach, expertise is always local. A globally dispersed crowd adds local expertise to data.
Add your voice to improve data’s crowd
If you have an experience or perspective to share about crowdsourcing data improvement, or a specific question you want to see answered by this blog series, then please post a comment below.