The Business Forecasting Deal
Exposing bad practices and offering practical solutions in business forecasting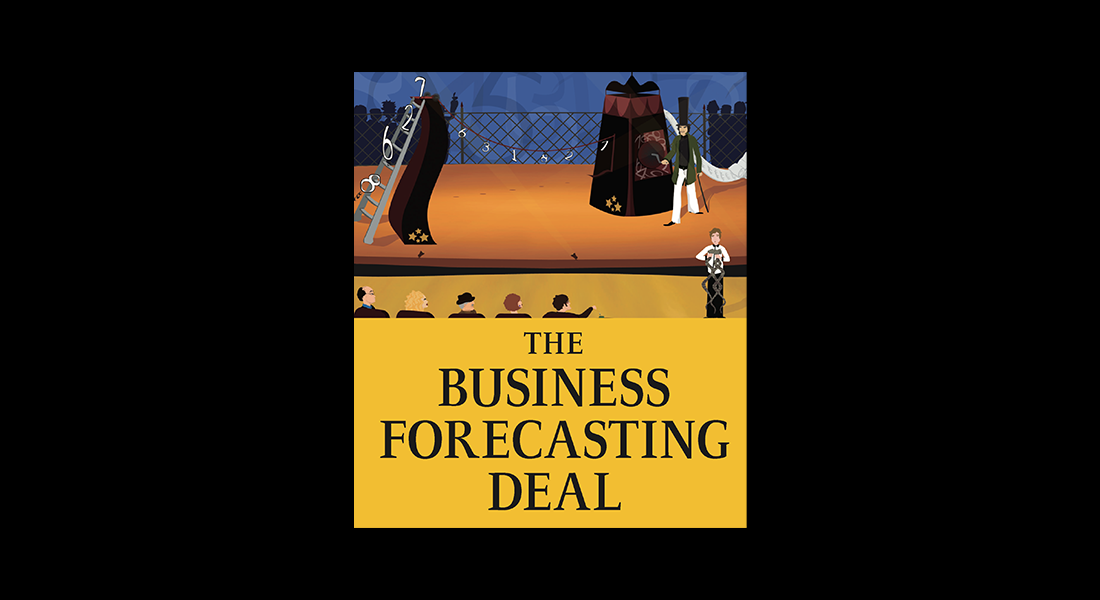
It has been my great pleasure writing this blog for the past 12 years, with over 370 posts. In doing so, I have enjoyed the support of my exceptional SAS managers over this time: From Anne Milley and Renee Nocker way back when it started, to Susan Kahler and David
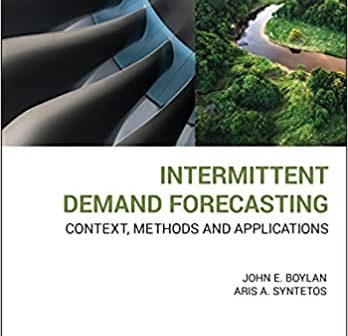
I've never been much of a fan of forecasting approaches to intermittent demand. In situations like intermittent demand (or other areas where we have little hope of reasonably accurate forecasts), my thinking is "why bother?" If we can't expect to solve the problem with forecasting, we need a different approach.

Following is editor Len Tashman's preview of the 2021-Q4 issue of Foresight: The International Journal of Applied Forecasting. Preview of Foresight #63 (2021-Q4) FORESIGHT HALL OF FAME Adopting the idea from other journals that recognize outstanding contributions to the field through best paper awards, we are pleased to announce that
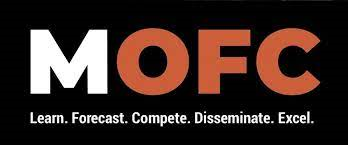
M6 Financial Forecasting Competition The Makridakis Open Forecasting Center has announced the M6 Financial Forecasting Competition, to begin in February 2022. This will be a "live" competition running through February 2023, with a focus on forecasts of stock price (returns) and risk, and on investment decisions based on the forecasts.
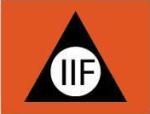
The International Institute of Forecasters and SAS® are funding two $10,000 grants to support research on forecasting. Per the announcement: For the eighteenth year, the IIF, in collaboration with SAS®, is proud to announce financial support for research on improving forecasting methods and business forecasting practice. The award for this year will be
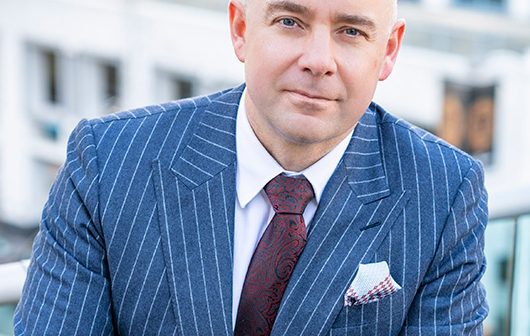
On September 2 (3pm UTC / 11am EDT), I'll be joining Jonathon Karelse, CEO of NorthFind Management, for an interactive "fireside chat" on the application of Behavioral Economics in demand planning. This is part of the Foresight Webinar Series, and registration is free. Since we first met at an Institute