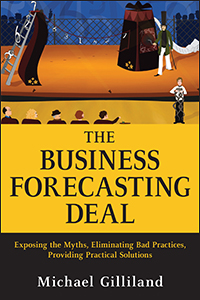
To make it easy to identify non-value adding areas, you can build a simple application using SAS® Visual Analytics software. Such an application lets you point and click your way through the organization’s forecasting hierarchy, and at each point view performance of the Naïve, Manual, Statistical, and Automated forecasts (or