Authors: Subramanian (Subbu) Pazhani and Fang Liang
The SAS Strategic Supply Chain Optimization model is a comprehensive and adaptable solution that offers businesses numerous advantages. It provides the capability to optimally and simultaneously address the entire supply chain. This model enables organizations to proactively identify the best responses to disruptions across the supply chain. Additionally, it swiftly estimates the financial and operational impacts of various scenarios. This post will provide an overview of how the SAS Strategic Supply Chain Optimization model tackles complex supply chain challenges and makes prompt, accurate decisions to manage fluctuations and disruptions throughout the end-to-end supply chain.
Given the global nature of today’s businesses, supply chains have become increasingly fragile and constantly disrupted in recent years. These include geopolitical events, weather-related catastrophes, labor shortages, strained transportation capacity, and volatile demand patterns. Additionally, raw materials sourced internationally can have large lead times with high variability, ranging from a few weeks to a few months. This adds complexity to production planning and scheduling at the production facilities.
Currently, organizations are typically reactive and siloed in their ability to respond and manage supply chain disruptions. A reactive approach in managing disruptions will increase operating costs and lead to profit/revenue implications.
What makes the SAS Strategic Supply Chain Optimization model unique?
The SAS Strategic Supply Chain Optimization model uses mathematical optimization to optimize end-to-end supply chains. The objective is to maximize profit by balancing supply chain costs and business constraints. The model incorporates the demand plan from Sales & Operations Planning. It considers production capacities at the manufacturing facilities, inbound/outbound logistics, supplier capabilities, and costs related to purchasing, logistics, production, and inventories. The model delivers optimal plans for purchasing, production, transportation, inventory, deployment/distribution, and sales at weekly or monthly granularity.
Some key features include:
- Dynamic model framework: The model framework is dynamic by design and can be configured easily to model and analyze any type of supply chain network structure and size. Two example network structures are shown in Figure 1 and Figure 2. Figure 1 is an end-to-end supply chain network involving suppliers, manufacturing plants, distribution centers (DCs), warehouses, and retailers. Figure 2 shows a network structure without considering material purchasing and involves manufacturing plants, DCs, and retailers.
Figure 1: Example network structure 1
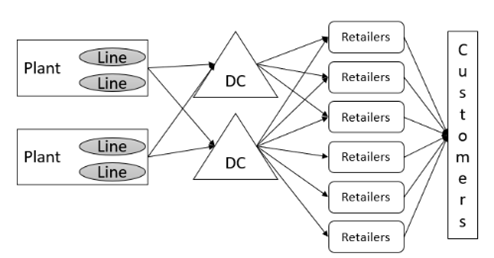
- Perishability of goods: The model can handle perishables for final products, intermediate materials, and raw materials. The model can also handle a mix of perishable and non-perishable goods in the supply chain. The optimal plans for purchasing raw materials, production, transportation, inventory, distribution, and sales are optimized based on the perishability of the goods.
- Alternate raw materials: The model can consider alternative raw materials for producing final products and intermediate materials. Given the cost fluctuation and availability constraints for certain raw materials, this feature in the model gives the business the flexibility to determine the best possible strategy for raw material sourcing.
- Product upgrade/substitution: The model can manage product substitutions due to product upgrades/engineering changes at the final product level.
- Connected production lines: The model can deal with various types of connections between production lines in manufacturing plants, where the production from the upstream line is consumed by the downstream lines.
- Multi-level BOM: The model can handle multi-level Bill-of-Material relationships with multiple raw materials, multiple intermediate products, and multiple finished products.
- Multi-period model: The model can take care of multiple time periods (could be as granular as a day) for operating the supply chain network, to satisfy demand for multiple finished products.
- Secondary production time, lane capacity, and location capacity: The model can manage overtime production in the manufacturing lines and regular time production. It also handles premium shipping (spot rates) for transportation lanes, along with regular shipping, as well as premium storage capacity at the storage facilities. The secondary production cost, storage cost, and shipping cost are higher than the regular cost. The user can specify secondary cost and the secondary available quantity, which will help cater to an increase in demand.
SAS Strategic Supply Chain Optimization model use cases
The SAS Strategic Supply Chain Optimization model can be used in various ways to solve supply-planning problems for various types of businesses or parts of the supply-planning process. The dynamic model framework enables you to use this model to suit their needs. Some supply planning use cases include optimizing the end-to-end process from suppliers to the end users, optimizing a business without considering external material purchasing, and optimizing a business with contract manufacturing facilities.
Potential scenarios
Given its flexibility and unique features, the model can be used to analyze various scenarios in the supply chain. Some of the possible what-if analysis scenarios include:
- Significant shifts in demand due to new promotional strategies or market changes. An example would be when demand for PPEs and sanitizers increased exponentially when COVID hit. How can you best meet the increased demand signal and cost vs revenue tradeoff?
- Production lines are unavailable for unplanned maintenance. A critical machine breakdown in a manufacturing line is causing a significant capacity impact. How do you quickly strategize alternative production plans to meet the demand without impacting revenue?
- A warehouse is temporarily shutting down during the Thanksgiving season due to a hurricane. How do you change your logistics/distribution plans to deliver products to retailers and ensure on-shelf product availability?
- Manufacturing capacity decreases to 75% due to labor constraints caused by a labor strike. How do you quickly strategize alternative production plans to meet the demand without impacting revenue?
- A major competitor is exiting the marketplace. This will increase demand beyond the initial forecast and plan. How can you best meet the increased demand signal, cost vs revenue tradeoff, overtime, premium storage, spot rate transportation, and so on?
- Procuring 80% of a raw material due to supplier scarcity. Imagine a natural hazard or supplier production disruption affecting the supply of absorbent core from your primary supplier. How do you quickly react to minimize disruption to your supply chain? Purchase the material from your secondary supplier source? What is the cost impact and revenue impact? Can we renegotiate the contract with the secondary supplier source?
How does the model work?
SAS Strategic Supply Chain Optimization is a containerized independent model. In other words, you do not need SAS to use the model. The offering comes with a REST API to interact with the model. By using REST API endpoints, the API creates asynchronous tasks that run in a process separate from the API server. The offering includes all dependencies needed for the model and the REST API.
You have the flexibility to call the API from any client, for example, SAS or Python. Thus, you can stay within your environment and seamlessly integrate the model into your pipeline to enhance your decision-making.
What are the results of the model?
The SAS Strategic Supply Chain Optimization model delivers the following key decisions for an end-to-end supply chain to meet customers’ demand:
- Purchasing decisions: Quantities of raw materials to be purchased from suppliers by the manufacturing plants.
- Production decisions: Quantities of intermediate and finished products to be produced in each line within every manufacturing plant.
- Logistics/distribution decisions: Quantities of products shipped in the supply chain to fulfill customers’ or retailers’ demand.
- Inventory decisions: Quantities of products stored as inventory in the supply chain.
- Sales decisions: Quantities of products sold to the end users.
Summary
In summary, this model offers powerful and intuitive optimization capabilities, featuring a streamlined mathematical optimization model tuned for performance. It provides a flexible framework designed to address and resolve complex supply chain problems swiftly. The model employs a data-driven approach, integrating data from both internal and external sources, and applying proven data preparation and validation techniques. This approach allows for the rapid execution of various recovery scenarios. By identifying the best supply chain responses to numerous scenarios, the model enables quick and accurate decision-making. The agile, iterative process from data to analytical insights is crucial for making prompt and accurate decisions during times of uncertainty.
The SAS Strategic Supply Chain Optimization model supports businesses in optimizing their end-to-end supply chain to reduce cost and increase responsiveness, leading to customer satisfaction. It supports the company’s long-term business growth, market expansion, and resilience to disruptions.