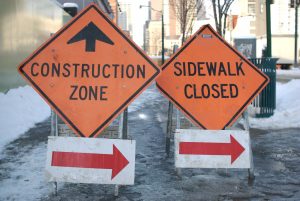
If a cinderblock falls from the 4th floor of a building and crushes a construction worker’s foot, it’s terrible. If it lands on his head, it’s truly tragic. But the difference is sheer luck.
Every employee deserves to return home safely from work. Unfortunately, accidents happen, which is why employers must carry mandatory workers’ compensation insurance for their employees. Workers’ compensation insurers strive to reduce accidents and promote safe working conditions. Like many departments, they have limited resources and thus need to target high-risk policy holders to get best bang for their buck.
Achieving a more detailed view of workplace risk
Employers have their own safety programs and initiatives which tend to have more data available than worker’s compensation insurers do. The most straight forward way to identify high safety risk is using past high-cost claims for serious injuries. There’s correlation between high-cost claims and risk, but such an analysis fails to account for less serious incidents that could have easily been a lot worse. Details matter.
This is where analytics comes into play. Using the cinderblock example for earlier, a claim severity model can be developed using historical claim information to assign a safety severity score. In our previous example, the cinderblock hitting the worker in the head or back might yield a severity score of 97 on a scale of 0-100. The less severe incident, though still serious, might score an 85.
By being more specific in the scoring we can arrive at a more accurate and nuanced view of risk.
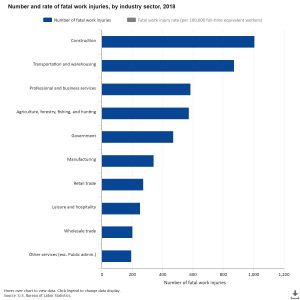
Looking beyond accidents and injuries to a more holistic risk perspective
With more data we can look beyond employee safety risk. For instance, is there a relationship between high safety risk and elevated risk in other areas like fraud or non-compliance?
Policy premiums are based on classification of work, which reflects the injury risk. It can be National Council of Compensation Insurance classification or something companies have developed themselves, but they all work under the same principle.
Let’s use farming as an example. Hand picking typically carries a lower risk than machine operation, so they’re classified differently. An employer might report some machine operators as hand pickers in order to lower their workers’ compensation premiums.
The safety severity model can be used to identify these unscrupulous employers based on claims history, especially the ones that have avoided a more thorough scrutiny of their policy. A safety risk model that accounts for more detailed examination of injuries will more effectively capture an employer’s risk.
The employer that misclassifies employees at lower risk will have a lot of explaining to do when the model says their risk score is much higher, based on their workers compensation claims. It will be particularly glaring when they are compared to their peers whose claim history properly aligns to the reported classification. As any farmer knows, it’s not good to be the weed standing tall amongst the grass.
Safer workplaces, lower premiums, healthy employees
Following the rules, while guided by a more precise safety severity models, benefits everyone involved. Safety analysis and fraud detection go hand-in-hand, using the same datasets and analytics. The viewpoint is just different. Working together, safety and compliance departments not only both improve their operations but do so cost effectively. The employers win too by improving the safety of their workplaces, which leads to reductions in premiums. And, most importantly, the employees win. Improved safety conditions help them return to their loved ones in the same condition they left. And should an accident happen, they are properly covered for the type of work they perform.
Effective analytic strategies help prevent incidents, accidents or even equipment failures using existing data. Learn how advanced analytics can help prevent the next safety incident in a webinar on employee safety and incident management.
1 Comment
That's some informative content on workplace accident and claims.