Artificial intelligence often seems misunderstood, especially in fraud. The same is true of machine learning. One of the amazing things about them is they ask the unasked questions. This occurs as artificial intelligence (AI) and machine learning (ML) go about their daily work.
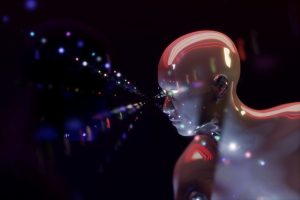
So, what is the unasked question?
Too often, it remains unasked because it remains unknown. AI and ML are finding new and unexpected answers to questions that you didn't know you had. Artificial intelligence and machine learning can filter through enormous amounts of data, and find the proverbial needle in the haystack by looking at every piece of hay. A person couldn't do this efficiently, if at all.
You might have millions of pieces of data in your haystack. AI and ML can be trained to review each piece and do much more. With advanced data analytics, these tools can find patterns in the data that you didn’t know existed – the unasked question.
Using AI and ML to fight fraud
A data analyst or fraud investigator may know what types of bad things they want to find in the data, which is the questions they know to ask. For example, did the system make payments on healthcare claims for dead people? That’s an obvious error. Once these analysts and investigators know that a healthcare claims payment system doesn’t get updated with a date of death right away, they learn the system will pay claims that look normal but occur after the date of death.
This is the nature of rule-based claims payment engines, where some data used for edits arrives after the claims are already paid. That’s just the nature of the process. At worst, fraudsters will exploit such weaknesses and disappear before the pay-and-chase models can catch them. At best, this is a government approved interest-free loan where providers repay the claims years later.
By recognizing patterns in the data that have potential meaning, AI and ML propose the unasked questions. They may find a pattern of providers who consistently bill for services provided after the date of death. Further, AI and ML might discover that a portion of these, or other, providers bill claims for dead people even after the claims system knows the patients’ dates of death. These providers may only bill codes the system allows to be paid due to programming errors. That seems like fraud.
AI helps payers get more than rules-based edits
These data analytics algorithms may find strange patterns like providers with a large percentage of claims which repeatedly get denied. Yet, the providers never rebill corrected claims later. Why not? This happens when fraudsters are “fishing” a rules-based claims payment engine to see which codes can be abused. Then, the provider keeps billing those codes. Thus, the game of pay-and-chase continues, but it is the bad provider who generally wins.
An example might be injectable drugs where one vial contains a normal dose of 40 mg. A claim should be billed for 1 unit (vial), but the provider bills 40 units. If the claims payment system doesn’t have an automated rule in place to catch this, the system may reimburse the provider 40 times for the rate of the one vial. A provider committing fraud or abuse keeps billing that way and collecting large overpayments hoping that nobody notices.
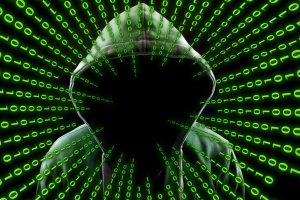
Once caught, even the fraudulent providers will say they thought this was okay since the claims system paid it. AI and ML algorithms can easily find such issues and identify who is committing them. So, investigators can take timely action, rules-edits can be fixed, and dollars lost can be limited. Recovering healthcare overpayments is like solving a murder, investigating sooner produces the best results. I have seen some Medicaid programs pay back millions of dollars to CMS for allowing these types of simple errors to go on undetected for years. Pre-payment analytics can prevent this and detect errors faster to limit losses and unnecessary costly repayments.
AI and ML fight fraud with adaptive learning
Machine learning algorithms benefit from adaptive learning. It’s more complex, but I think of adaptive learning like e-mail spam filters. With the origination of e-mail decades ago, we got many spam e-mails. As a user identified an e-mail as spam, the machine learning algorithm built into the spam filtering software began to learn what spam looks like. The software then moved these into a spam folder for the user to review and confirm. The more the user identified spam, the better the filtering became.
Modern adaptive learning in ML can do even more. With machine learning, the algorithm can be trained to look for potential fraud and then assign a risk score based on claim, provider or member. The various pieces of data reviewed can be weighted to affect the scoring. The analytics software can run thousands of models adjusting the scoring weights in various combinations to determine which is most accurate. The best model is implemented in production. A human couldn’t do this.
With SAS fraud solutions, the algorithm model can go through adaptive learning. In production, these fraud alerts can be assigned a final disposition such as probable fraud, review later or not fraud. Here is where machine learning can be very powerful. The ML algorithm learns and adapts based on this new information. It can re-run the scoring models thousands of times to see if the new information identifies an even better model. If so, that new model can be implemented. This is like a quality feedback loop where the scoring gets better and better over time. It is one reason why SAS enterprise fraud solutions and data analytics are so highly ranked by industry analysts like Forrester.
Fraud in the news
November 11-17, 2018 is International Fraud Awareness Week, which is a global effort to minimize the impact of fraud by promoting anti-fraud awareness and education! Show your support and join the effort to stop fraud by sharing your voice on Twitter for a #SASChat. We will explore the topic of identify fraud with the help of The Identity Theft Resource Center and the ACFE. Mark your calendar for Friday, November 16th at 10 am eastern. We'll be asking these questions:
- We hear a lot about identity theft in the news. What is the size and nature of the problem?
- What is synthetic identity theft and who is most at risk? How can we protect ourselves?
- Let’s specifically look at child identity theft. What steps should families be taking to protect their children?
- Let’s talk about impact. What are the major kinds of economic losses created by identity fraud, and also, could you give us a sense of the non-economic impact of this crime?
- What’s the good news? How can consumers and businesses fight back against fraudsters?
Here's everything you need to know to participate in this #SASChat celebrating #FraudWeek! We hope to see you online!
1 Comment
Pingback: Common ground for future fraud detection techniques - Hidden Insights