Deploying AI insights isn't just about pushing buttons and hoping for the best.
The deployment phase is a pivotal moment where technology and ethics meet. When transitioning AI models from development to real-world use, prioritizing trustworthiness remains important.
It’s not just about algorithms; it’s about how AI impacts people and societies based on the principles governing their implementation.
Deploying AI insights is the fourth step in a series of blog posts detailing the five pivotal steps of the AI life cycle. These steps – questioning, managing data, developing the models, deploying insights and decisioning – represent the stages where thoughtful consideration paves the way for an AI ecosystem that aligns with ethical and societal expectations.
This phase demands more than a technical lens; it necessitates a comprehensive exploration of the broader ethical dimensions intricately woven into the life cycle of AI.
The concept of transparency is essential here– how can we ensure a clear understanding of the processes involved in implementing AI insights, and more importantly, how can this transparency be consistently maintained throughout the entire deployment life cycle?
Questions like those and the following five should be asked to pursue a smooth and safe model deployment:
How do you monitor the AI’s appropriate performance metrics, such as accuracy after deployment?
When deploying AI Insights, it's crucial not to launch and leave it. This question highlights the importance of ongoing vigilance. After your AI model goes live, how do you monitor key performance indicators like accuracy? It's about ensuring that the model doesn't just perform well on day one but continues to deliver reliable results over time. Monitoring these metrics allows you to catch and correct drifts or biases early, maintaining the trustworthiness and effectiveness of your AI system. It's an essential practice for keeping your AI aligned with its intended purpose and ethical standards.
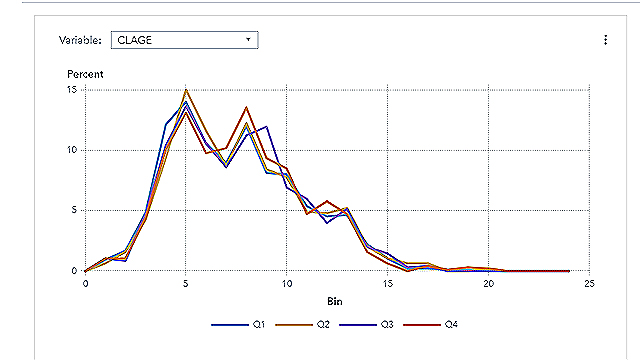
As time passes and conditions change, are you evaluating training data to be still representative of the operational environment?
Over time, as conditions evolve, it becomes imperative to revisit and reevaluate your training data. Is it still reflective of the current operational environment? This is about recognizing that the world doesn't stand still. New data comes in, trends shift and what was once a perfect training set can become outdated. Regularly assessing your data for its ongoing representativeness ensures that your AI system remains relevant, accurate and fair, adapting to changes rather than being left behind. It's a critical step for maintaining the integrity and effectiveness of your AI deployment.
What actions will you take to ensure your model's reliability and transparency throughout its life cycle?
Ensuring your model's reliability and transparency isn't a one-time task; it's a commitment that spans the entire life cycle of the AI. What specific steps will you implement to maintain these critical qualities? This could involve regular updates based on new data, thorough documentation of changes and decisions, and open channels for feedback and audits. It's about creating a continuous dialogue between the model's performance and stakeholders, ensuring it performs consistently and remains understandable and accountable to those it serves.
Fig 2: Trustworthy AI life cycle workflow
How will you test and strengthen your model's defenses against adversarial attacks or manipulations?
Think of adversarial testing, like giving your AI model a crash course in self-defense. Just like teaching someone how to block a punch or dodge a kick, you're putting your model through the paces to ensure it can handle the sneaky moves users might throw at it. You identify and shore up vulnerabilities by simulating attacks and manipulations, enhancing the model's defenses.
This continuous process of testing and strengthening is vital for maintaining the model's integrity and ensuring it remains a trustworthy tool for users, effectively preparing it to resist and recover from the inevitable attempts at exploitation in the wild.
Did you think about a way to roll back the model, if necessary?
Imagine launching your AI model like sending a rocket into space, but with a parachute attached, just in case. Your plan should be to safely bring your model back to Earth if things go sideways! If something goes wrong after deployment, do you plan to revert your AI model to a previous, stable version?
This is about anticipating and preparing for scenarios where the model might not perform as expected or could cause unintended consequences and harm.
A rollback strategy ensures you can quickly restore service and maintain trust with your users, minimizing potential harm or disruption. This is a critical part of risk management, ensuring that your deployed model is not only forward-thinking but also prepared to step back when necessary to safeguard the reliability and integrity of your AI Insights.