The emergence of COVID-19 and the resulting global pandemic keeps presenting new challenges and threats to global public health. While we were – and still are – battling the disruption caused by the pandemic, the fast development of highly efficient vaccines offers a hopeful prospect.
That`s an impressive achievement, considering that vaccine development processes take an average of 10 years or more. Only a small percentage of vaccines that are at the very start of the process ever make it to clinical testing. And regulators approve even fewer for widespread use. This controlled approach to development is in place to help us ensure vaccines are safe. And that mistakes made in the past do not happen again.
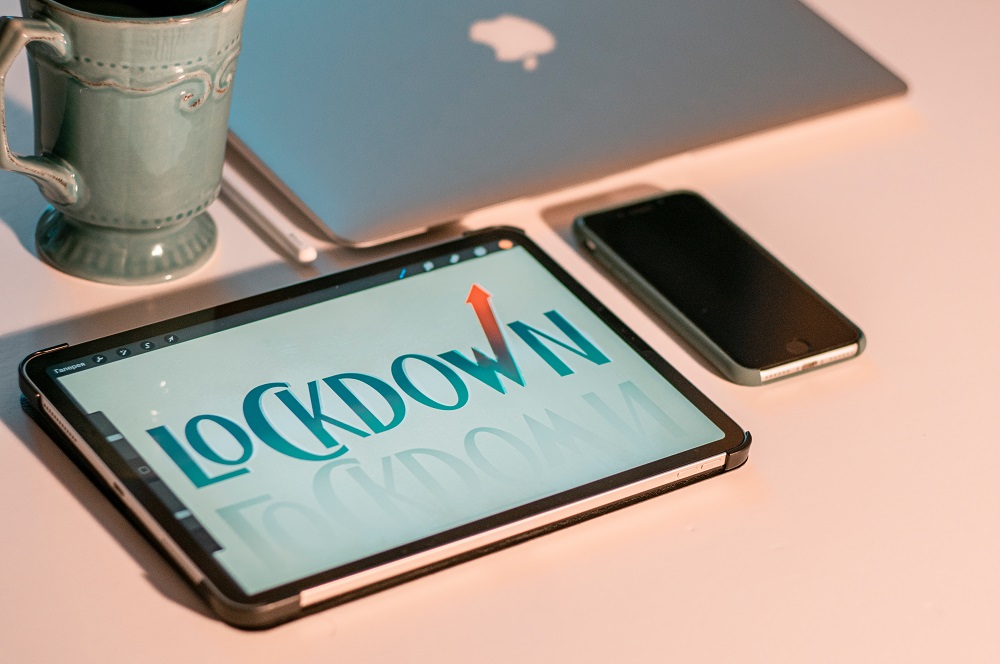
COVID-19 vaccine development was unique in that the development and approval time was far faster than what is the norm. However, despite the speed of the process, the level of rigour and care taken during testing and evaluation of data was not sacrificed.
Implications of generalizability and reproducibility in clinical research
The process of gaining approval for new drugs and treatments is rigorous for very good reasons. But what exactly are the approving bodies looking at? They evaluate not only the reproducibility of the clinical trial but also the generalizability. Reproducibility evaluates whether the results of our clinical trial can be reproduced in the same target population in another location.
Generalizability asks whether we can generalize the results of our trial from our original study population to another. For example, can a trial including only men be generalized to women? What about from one demographic to another? Or from one regional population to another region or country?
Failure to consider these two elements continues to come under scrutiny in clinical research. In the context of human disease, gender, age and race all affect individual susceptibility. This includes how the disease manifests and treatment outcomes. Failure to account for the differences between these groups has a misleading effect on the true efficacy of treatments vs. reported efficacy, uncertainty in required dosage by group, and more.
Considerations in other fields
Clinical research is not the only field that must consider how to structure studies, collect data and generalize the results. Almost all industries are considering and discussing the topic of responsible AI.
When we build models and make decisions based on them, we want to have a high enough degree of certainty that the decisions we are making are “correct." Here is where generalizability and reproducibility come into play.
Generalizability
Generalizability is important when we are making conclusions based on our model outside of the data (population) from the original study. There are two aspects of generalizability that may be important to consider:
- Do we have representative and unbiased training data?
- Does our model react appropriately to new and unseen data?
For example, if we are trying to understand the risk of an adverse effect for a medication, a model trained on data from patients all from the same demographic and region may not accurately determine risk in patients that belong to a different group. On the other end, we may also end up overfitting or building an overly complex model that does not properly predict patient risk for a new set of patients.
In both examples, assuming generalizability when it is not valid could potentially have disastrous consequences.
Reproducibility
Documentation, lineage, governance and an understanding of the model itself are crucial when considering reproducibility in AI. Even if we may not plan to recreate the model from scratch, we aim to understand at the very least why a certain decision is made and how we implemented it.
ModelOps can help govern and allow us to standardize our processes. But that is not the only step we need to take. Every part of the process is important here, from gathering the data to making use of our predictions.
How does responsible AI relate?
Responsible AI – and the guidelines and policies we are developing around this topic – focuses on ethics, transparency and accountability in every stage of the development process. Just like in clinical research, we need to ensure that we are acutely aware that while we have the opportunity to innovate and improve that there are also potential adverse effects if we do not have adequate control of our development and evaluation process.
If you want to hear more about responsible AI, join the virtual conference Artificial Intelligence and the Ethics Mandate.