In my previous blog post, I harked back to my first full-time job. My boss had a passion for using data and statistics to improve business operations. He introduced me to some of the theory behind this by sending me on a Deming course. I came away with the impression that the manufacturing industry had led the way in using data and statistics to drive continuous improvement.
Little did I suspect that my whole career would continue to focus on principles expounded by Deming. For the past 30 years, I’ve helped organisations create actionable insights using data and analytics. I’ve worked across just about every industry and with a wide range of public sector organisations. But strangely enough, until recently, I have not done much work with manufacturers. Why is that?
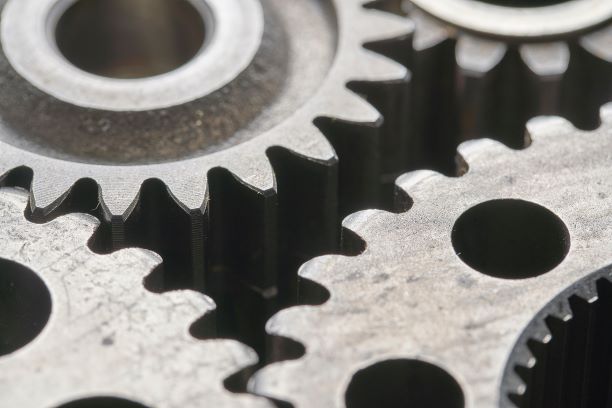
Opportunities for data analytics
In my experience, manufacturing has not made the same strides that other industries have made in harnessing data to proactively manage the business. This is also my impression of engineering-led industries that manage physical infrastructure, such as oil and gas and utility companies.
With the advent of the Internet of Things (IoT), it is easier than ever to measure all sorts of environmental and physical factors that affect a manufacturing line and its output. Yet I keep coming across manufacturers who still rely on physical inspection of samples of their product as they come off the line.
Far too often, organisations only use manufacturing process data to identify the cause of discrete problems after the event. Instead, they could take a proactive approach. There are likely to be complex patterns in the data that help identify the combination of factors that have a high probability of causing defects in products. Understanding early indicators of issues means manufacturers can take preventive action before problems occur.
Many manufacturers use Statistical Process Control measures to identify variations in equipment performance that can be early indicators of component failure. They may monitor things such as temperature and vibration. They are very likely to monitor usage-hours of equipment. But viewed in isolation, these measures only provide part of the picture.
Asset management businesses
I have also spoken with quite a few asset management businesses that use a "fix on failure" approach to maintenance. Bye-bye predictive analytics – we don’t need you. We can tell when it's broken, and when it's broken we fix it.
From manufacturers to physical asset operators, there is often little appetite to squeeze additional insight out of the data. Engineers tend to believe that there is little that the data can tell them that they don’t already know from experience. So what’s changed? Why am I now having a lot more conversations with engineering-led businesses that didn't express interest in predictive analytics before?
Technology has changed, that’s what. The IoT makes it easier to collect and access data about your assets and processes. Artificial intelligence and machine learning make it easier to make sense of that data to drive actionable insights.
I’ll talk more about this in my next and final post in this series. In the meantime, you might want to watch this webinar to find out more about how companies that use the powerful combination of AI and IoT, or the Artificial Intelligence of Things (AIoT), are more competitive and efficient than those using IoT alone.
Data collected during the manufacturing process is far too often only used to try to identify the cause of discrete problems after the event. Instead, manufacturers could take a more proactive approach. Click To Tweet