It’s been over a year since my previous blog post, so it seemed like a good time for a refresh. Also, it’s just over a year since the EU implemented GDPR. This regulation promised users could take back control of their data and opt-in/opt-outs, while businesses could get fined up to 4% of their annual turnover.
I wish I could say we could say there have been great improvements. Yet consumers’ data is handed over in what seems to be a largely uncontrolled manner, as cookie settings on websites are either confusing or difficult or both. Have a look here at one individual’s attempt to understand how his data gets used. In his case, his robot vacuum sends the layout of his house back to servers in the Far East. Then the servers share that information with other organisations. Another example is how multiple organizations share information about the use of a smart light.
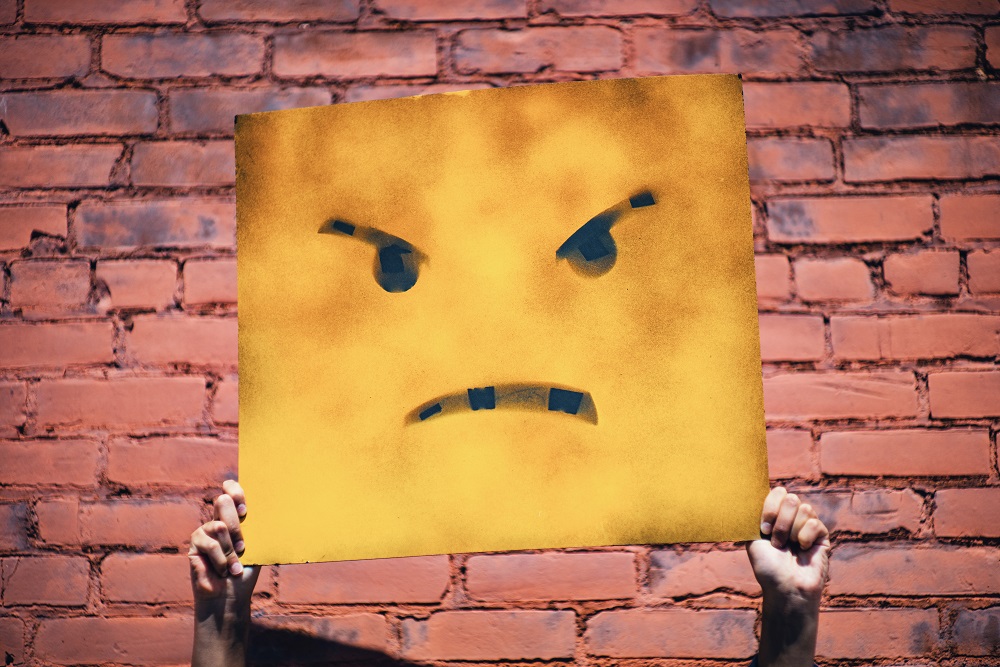
Concern about facial recognition
Facial recognition has attracted a lot of attention in the last year, from law enforcement to retailers. At the recent SAS Global Forum, we demonstrated the capability with a number of volunteers. We could argue the rights and wrongs about the use of such technology. For example, retailers have used CCTV at shop entrances for many years to prevent crime. But retailers can now store, retrieve and compare individual faces across all members in the consortium.

My concern relates to the algorithms behind such techniques as they’re often based on white males within certain limited age groups (I probably fall into this category). By definition, they often don’t reflect the diversity of the population, which ultimately results in bias. Given the number of faces used in training, they were probably not collected under ideal studio conditions. And they are almost certainly not being applied in ideal conditions, which only further increases error rates.
Two types of errors
Two types of errors can occur. False positives occur when individuals are wrongly identified as suspects. And false negatives happen when individuals are not identified as suspects when they actually are. Organisations can "tune" these models to reduce false positives OR false negatives, but not generally both at the same time. Studies have shown that “errors” are not evenly distributed across the population by gender or race. What could this mean in practice?
Police stop and question innocent suspects. In this case, there is at least some scrutiny and police officers can quickly check and confirm the identity of the individual.
Retailers refuse entry to a store. Will there be genuine oversight on refusals and redress for individuals affected?
Note that organisations don’t need to (necessarily) store the actual face, just a mathematical representation, which actually may make it harder to prove innocence if it's just a set of numerical values.
My concern relates to the algorithms behind such techniques as they’re often built on white males within certain limited age groups (I probably fall into this category). By definition, they often don’t contain the diversity of the population. Click To TweetI am not a number – I am a person
In some jurisdictions, the use of facial recognition has been banned, whereas in others it is used overtly for monitoring and social reward schemes. Like many technology genies, the bottle is well and truly open, and what we need is democratic debate and discussion. Here are some areas for consideration:
- Standardised data sets to allow training of data across representative population groups.
- Published statistics of recognition matches and false positive/false negative rates on a regular basis.
- Deletion of match/nonmatch after a period of time – for example, 20 days for a match and one hour for a nonmatch.
Keep watching this space!
1 Comment
Agree with your sentiments. But I also feel a bit powerless and frustrated that even the hint of GDPR regulations do not seem to be catching on in the US.
BTW, is that an obscure reference to The Prisoner? https://en.wikipedia.org/wiki/Opening_and_closing_sequences_of_The_Prisoner