A speaker at a recent major tech event quipped that “they will only call it AI until it is useful”. The point was that AI for the sake of AI is pointless, and can even be counter-productive. As soon as someone conceives and develops an actual use case, they name the technology after the problem or value it addressed. In other words, when there are real AI-using solutions, we won’t be talking about "health care AI", we’ll be talking about, for example, cutting hospital-acquired infections by 30%.
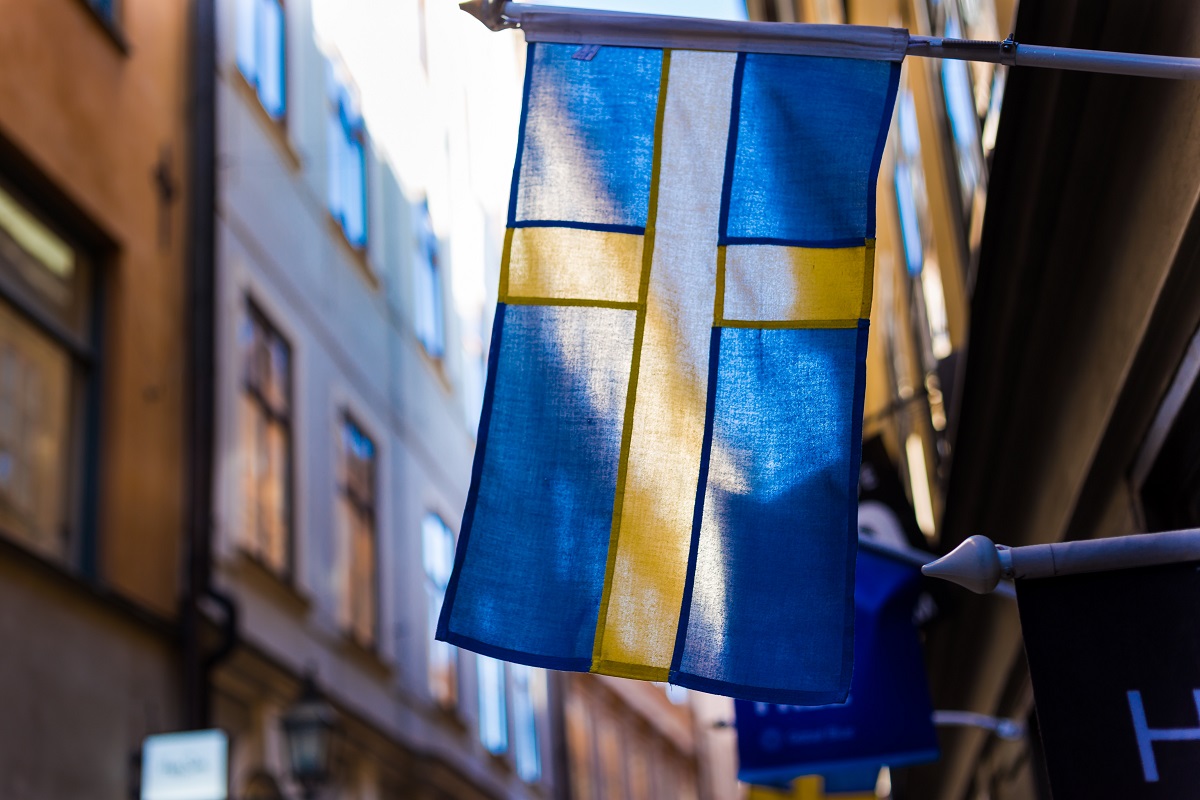
A year is a long time in politics (and technology)
I visited Almedalen for the first time in 2018. I wanted to understand the conversation around information-driven decisions, and particularly the use of AI, in the Swedish public sector. It was obvious then that we were still in the early stages—most of the conversations I heard were about the overall promises and concerns around AI. They touched on the nature of the technology, and whether it would create or destroy jobs.
The only actual use case discussed was image recognition in radiology. I don’t think a single other presentation described any use case, or successful implementation, of AI. The focus was on AI for the sake of AI. Very little time was spent analysing the value it would bring, or how to implement it to derive value.
It was therefore with some trepidation that I arrived for Almedalen 2019. How far would we have come in a year?
More than meets the eye
Actually, it turns out that there has been progress in the last year. The discussion had moved on, and was much more specific about the challenges of AI. Presenters raised issues including:
How do we deploy AI into production?
This issue was highlighted in East Sweden’s excellent session. Göran Lindsjö has suggested that our peers in the US sometimes see Europe as the “pilot graveyard”. The discussion in this session seemed to confirm this; there is a huge problem moving from promising pilots to a setting where models will produce reliable predictions at the right point in time and space to actually make a difference.
How do we manage models to avoid deterioration over time?
This was another issue Region Östergötland and East Sweden addressed. They engaged in an insightful discussion on the need for life cycle management of deployed AI models to avoid them becoming less accurate over time, as data and other factors slowly change.
How do we avoid training bias?
There were many sessions on ethics, and particularly how to avoid building models that inadvertently favour or discriminate against one gender or ethnicity. One discussion, however, was missing: how far to take "political correctness" so as not to get left behind when others deploy a more agile “shoot first and ask questions later” approach.
How do we ensure the safety of personal data?
This important topic was addressed by Tieto amongst others: How do we make sure that we do not violate personal integrity, or lose control of personal data, when we collect vast amounts of information for research into new AI applications? It is clear there is a lot to be done here. Other related concerns included the need for a structured use of quality register data, and nationwide informatics collaboration.
The term AI is still being used widely, but there were also voices calling for this to stop, and suggesting that the label ‘AI’ was counter-productive because it actually lowered interest. Click To TweetA maturing discussion
One thing, however, was still largely missing: actual, deployed solutions. It was therefore wonderfully refreshing to get a different view when Cleveland Clinic’s Chris Donovan gave us a tour of the projects at the clinic that have been labelled “augmented intelligence”. Donovan talked about the need to bring data together in a structured way (it turns out that the enterprise data warehouse is far from dead) and bridge analytic silos; a vision requiring a balanced view on people, processes, technology and data; the importance of collaboration; and how to construct a flexible but robust architecture. Finally, he talked about some use cases deployed and under construction. These included predicting and preventing readmissions, population health, personalized survival in cancer treatment, mortality after discharge, and resource optimization in operating theatres.
There is no question that the discussion has matured significantly over the past year. The term AI is still being used widely, but there were also voices suggesting that the label "AI" was counter-productive because it actually lowered interest. As the usefulness of AI becomes clearer, we need to stop using technology jargon and start talking about the far more important issue of business value.