My last blog post discussed the applications of AI in retail and how retailers were starting to use solutions like image recognition to improve customer experience. However, implementing AI is not without its challenges. This post continues the conversation with Shailesh Jain, Group Head of Analytics at Landmark Group, about the use of AI in retail.
Aneshan: What are the biggest challenges that retailers face when implementing AI solutions?
Shailesh: There is no shortage of tech companies developing AI tools and solutions. However, actually embedding these tools into processes requires a lot more work. For example, they need to be trained first using your customer data, which means you will have to get your data tagged first before you can start.
Also, a lot of the AI solutions are focused on specific tasks. This means that they are good at doing what they do and nothing else. E.g., online personalisation is only good for online personalisation. To move beyond a specific confined scope and have an AI engine sit in harmony with your existing platforms requires lot of development effort internally. And AI talent being in extremely short supply makes it even more difficult for retailers to embark on any kind of AI project.
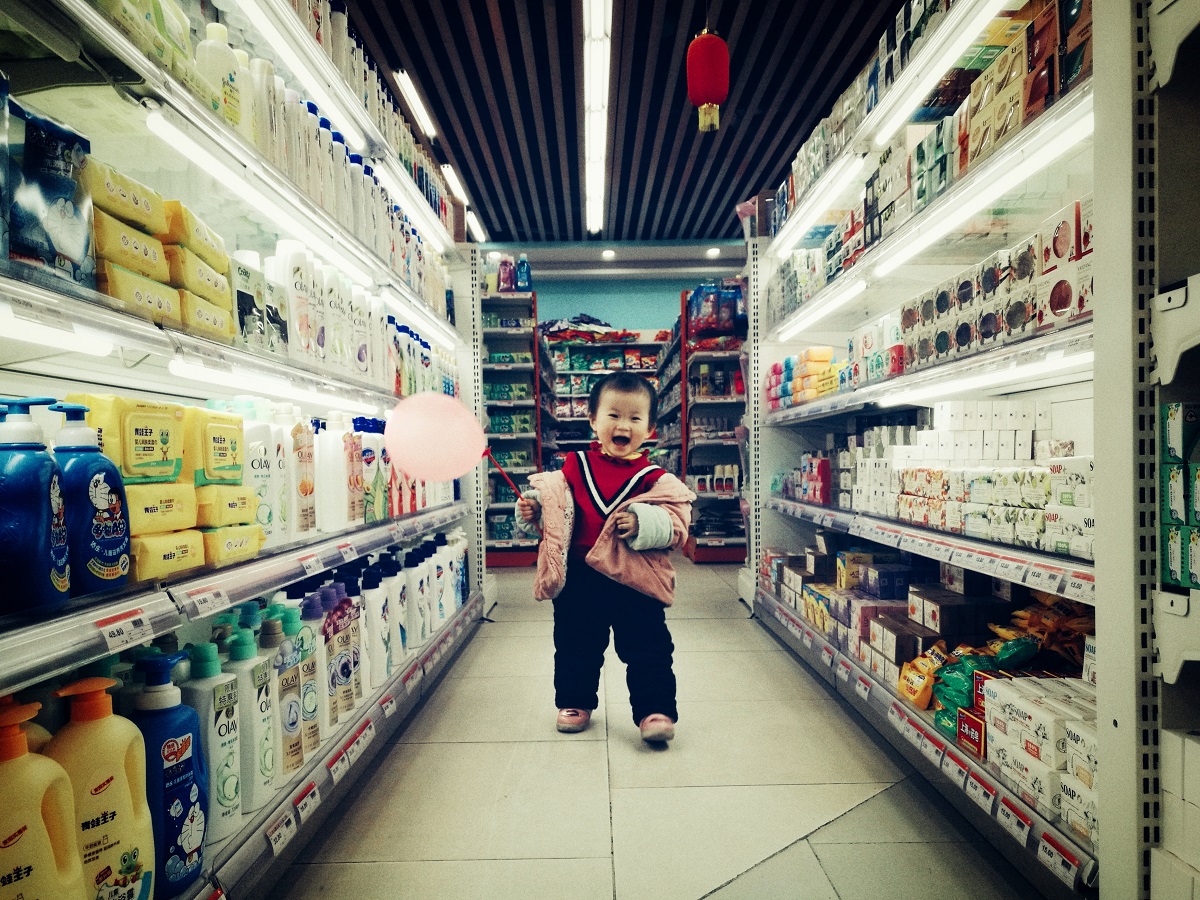
Aneshan: What advice would you give to analytics and AI practitioners when embarking on data science projects?
Shailesh: My number one piece of advice would be not to develop something just because it’s cool. Anything that you develop must create value for your customers, and it should also make commercial sense for the business. You need a clear measure of success defined upfront, with realistic cost and delivery estimates. It’s also a good idea to have an agreed return on investment and map out how the AI solution should be developed in harmony with your existing systems.
Aneshan: Do you find that machine learning techniques provide incremental value compared to traditional forecasting techniques?
Shailesh: Generally, machine learning models add great value, but opinion is divided on whether they are always superior to traditional methods. We have seen cases where ML models have produced extremely accurate forecasts, but I have seen other cases where a traditional technique, such as time series, has performed better than the ML models. ML models are currently going through the same phase as any statistical model did decades ago. Despite producing really good results, it's very hard to explain the model predictions. AI produces good results because it is better at handling noise in the data. But for more simple scenarios, I think traditional models may actually be better because we understand them better. We have overcome this issue of explainability with traditional models, and I am sure we will get there with AI, but not just yet.
Aneshan: How has SAS helped your organisation with regards to AI and getting insights out of your data?
Shailesh: I have to admit that we are not actually using SAS that much for AI. We tend to use other applications, but SAS does provide a very robust platform for us. The SAS Platform is extremely flexible and scalable. It’s also easy for us to use in conjunction with some of the other applications. Most importantly, the reason we like SAS is because it keeps on investing in R&D, which makes it a good partner.
Game changers in retail are facial, emotion and image recognition, says Shailesh Jain, Landmark Group Click To TweetAneshan: You mentioned having a robust analytics end-to-end platform. What, in your view, makes a good analytics platform?
Shailesh: It needs to be able to analyse both structured and unstructured data. Business is changing very rapidly, people have a lot of questions and they want the answers immediately. Secondly, you need a wide variety of tools as there is no one-size-fits-all approach. You're not going to find people with exactly the same skill sets across industries. You need to use a wide variety of tools, and more importantly you need to have access to all the libraries for various tools and applications available for analytics today. Lastly, your platform should also integrate well with your larger systems. The analytical platform cannot operate in isolation. It needs to connect with these platforms and not just for data extraction purposes – it also has to be able to put the intelligence back into these systems.
Aneshan: Finally, Shailesh, what inspires you?
Shailesh: This is an easy one. We hear about AI innovations happening all around us and not just in retail. I think we are lucky to be part of this journey and actually be involved in creating the wave, not just riding on it. This is extremely exciting for me. I can't wait to get up every day to have another shot at developing new solutions that are going to create more value for our customers.
SAS conducted a global survey together with Futurum including more than 1’000 consumers and brands from Europe, the Middle East & Africa to understand what defines customer experience today and how it’s evolving through the year 2030. Find the whitepaper here, and experience today, what customers require in 2030.
2 Comments
Hmmm ... a less sophisticated chatbot?
j'aime merci pour le poste