A recent survey by Forbes found that 73 percent of organisations are already piloting, experimenting or planning to deploy artificial intelligence (AI) algorithms. AI is driving a transformation across industries. In banking, for example, natural language processing is being used to explore sources of unstructured data such as customer comments, and learning algorithms are also being deployed to combat fraud and improve credit scoring, as well as to manage ATMs more efficiently.
There is, however, one important issue. In total, 60 percent of people surveyed by Forbes were concerned about the impact of AI-driven decisions on customer engagement. There are clearly big questions to explore here, especially in a highly regulated sector like banking, where decisions are required by law to be explainable and justifiable.
Understanding customers
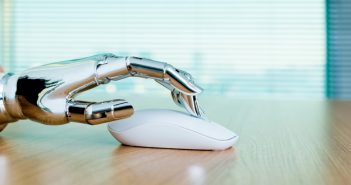
The clue may be in the phrasing: “AI-driven.” Computers and machines are not going to take over the world without permission. AI systems may be able to learn, but only in defined fields. Used correctly – that is, in partnership with humans in a way that enhances the abilities of both humans and algorithms – AI should be a tool that enables banks to understand more about their customers. This, in turn, should improve the interaction and communication with customers, making it more targeted and more relevant, and therefore improving the customer experience.
Automated systems can take on much of the basic work, freeing up bank employees to deal with more complex issues. Chatbots, for example, can already deal with several simple queries. Call centre employees can therefore focus on the more complicated issues, reducing customer waiting times. Algorithms are also improving the ability to coordinate across channels. They can identify the same customer using a different channel, and almost instantly draw down all the data about previous contacts, providing all the required context and making decisions more relevant. Options like “next-best offer” are revolutionising customer contact and experience across multiple channels.
In other words, AI is already improving customer experience by enabling banks to respond to customers more effectively, whether that is by managing their queries swiftly and efficiently or passing them on to a human partner when necessary. Customers may express reluctance to “talk” to a machine, but this is likely to change as they see improved speed and efficiency because of doing so. After all, we are prepared to withdraw cash from an ATM instead of queuing in the bank to see a human teller. We are generally prepared to use the easiest, most efficient option to get what we want, and evidence suggests that people are happy to consider using chatbots and other AI options – if they really do deliver improved speed and efficiency.
Ensuring quality
Quality of delivery, therefore, is the main issue: AI in banking will probably be acceptable to customers if it delivers. That is where humans come in. It seems likely that new roles will emerge working with AI algorithms to ensure that they are properly trained, and that they continue to deliver the “right” outcomes. Training algorithms require both a lot of data and the right data. It is easy for the unconscious biases of the trainers to inadvertently influence the algorithm by their choice of training data. Once those biases are installed, as it were, they are likely to be amplified, as the algorithm learns from its experience. Algorithm trainers need to monitor their own biases, but also be prepared to carry out checks and audits for themselves and others.
#AI in #banking will be acceptable to customers if it delivers. That is where humans come in as algorithm trainers. #CustomerExperience #cx Click To TweetAlgorithm audit is therefore an important issue. Banks will need to carry out regular audits of their algorithms. These will, of course, be necessary for compliance, because of the need to understand and be able to explain what is behind decisions that affect customers. A black-box approach is no longer acceptable. There are also ethical considerations to ensure that decisions remain fair and unbiased.
There is, though, another reason why audits might be necessary: to understand consumer preference changes and ensure that they are woven into the model. We want algorithms to learn and change based on customer preferences and behaviour. This is, fundamentally, the power of AI. We do, however, also need to understand how they are changing, and in response to what. Without that understanding, it is unlikely that algorithms will remain compliant with the law. This may require some imaginative use of data, and perhaps even completely new sources of data. Thinking outside the (black) box is essential.