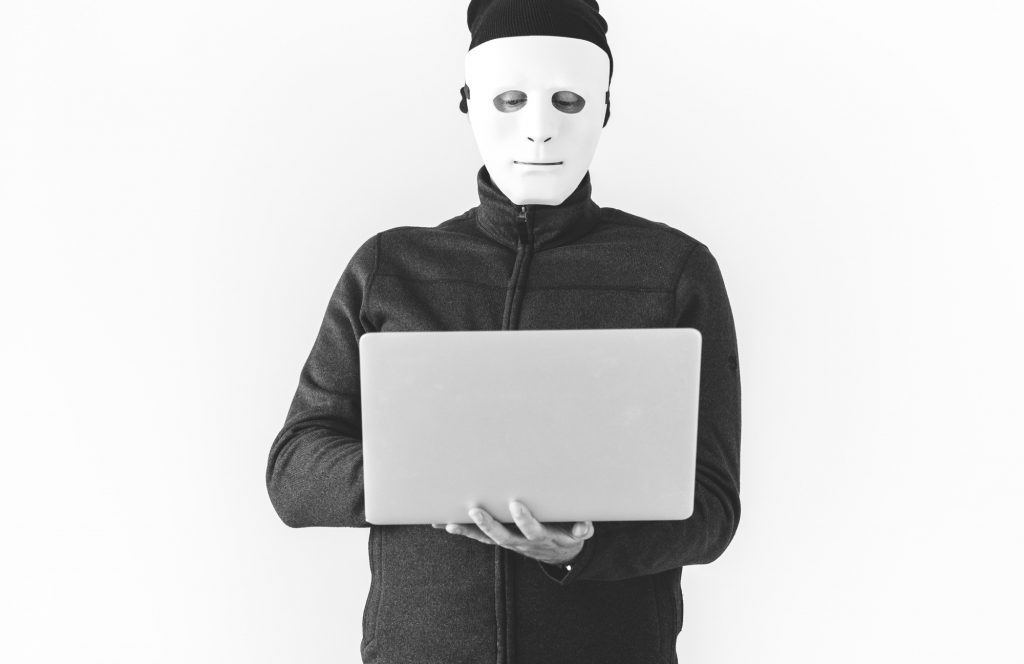
AI is everywhere these days, whether in reality or just as a hyped-up label for some simple rules based decisioning, and this has led to some interesting problems. The first of these is mistrust, as noted by the incoming president of the British Science Association, Professor Jim Al-Khalili: “There's a real danger of a public backlash against AI, potentially similar to the one we had with GM back in the early days of the millennium”. In essence, he warns that without greater transparency and public engagement the full potential of AI may not be accepted.
The second potential issue is that of control; if models are truly left to run without monitoring and control then there is potential for poor decisions. An example of this could be the “Flash Crash” in 2010 when the US Stock market dropped about 9% for 36 minutes. Although the regulators blamed a single trader spoofing the market, algorithmic trading systems were at least in part to blame for the depth of the crash.
That said, AI has huge potential for good, whether providing better cancer diagnoses through more efficient screening of tumour images or protecting endangered species by interpreting images of animal footprints in the wild. The challenge is to ensure that these benefits are realised, and this is where the FATE (Fairness, Accountability, Transparency and Explainable) framework comes in, which is designed to ensure that AI is appropriately used. I will focus on the Transparency aspects, where Data Management has the greatest impact.
AI application processing requirements
AI can only ever be as good as the data that feeds it. This was a running theme in a survey that SAS carried out at C-Level in 2018, “AI: Momentum, Maturity and Models for Success”.
To build and use an AI application requires a number of data specific phases:
- Data quality cleansing to ensure that modelling is not performed on data which contains irrelevant or incorrect items
- Transforming, joining and enhancing data before the modelling process begins
- Deployment, which takes the model and applies it to the organisation’s data to drive decision making
Each of these will add value but also potentially alter the results of the AI process. For example, if the data quality process removes outliers it may have very different impacts. If the outlier removal is appropriate the result will be a model which reflects the majority of data very well. On the other hand, it might ignore a rare but critical circumstance and miss the opportunity to bring real benefit.
This was shown in Dame Jocelyn Bell Burnell’s discovery of Pulsars, a type of rotating neutron star. She was examining literally miles of printout data from a radio telescope and noticed a small signal in one in every 100,000 data points. Despite her supervisor telling her it was man-made interference, she persisted and proved their existence by successfully looking for similar signals elsewhere. Had the outliers been removed she would not have made the discovery.
Data Quality should also be applied to prevent embarrassing decisions. If Bank of America had checked the validity of their Name data, they might not have sent a credit card offer to “Lisa Is A Slut McIntire” in 2014. They had acquired the data from Golden Key International Honour Society, which recognises academic achievement. An unknown individual had edited her name in the register of members.
Data processing is an integral part of the #AI process which will have a significant impact on the results of AI. Click To TweetData transformations
The data journey then continues with transformations to prepare the data for modelling; source systems are typically highly normalised and have information stored in multiple tables, whereas data scientists like a single square table to analyse. They will often need to add derived variables to help their analysis. These are usually defined initially in an ad-hoc data preparation environment by the data scientist but will need to be moved to a more controlled environment for production purposes.
The impact of this data transformation stage can be huge. Firstly, it is important to understand which data sources are being used in the analysis. This may be in relation to regulatory concerns such as whether personal data is being used, or simply to ensure that the correct data source is being accessed. Secondly it is important to understand whether the transformation has been appropriate and correctly implemented; errors in implementation can be just as damaging as poor-quality data.
The final data process that directly impacts on AI is deployment, ensuring that the correct data is fed into the model and using the results to make decisions which directly impact on the organisations’ performance. Models have a definite shelf life during which time they accurately predict the real world, so if it takes too long to deploy models into production they will not deliver their full value.
Stay compliant
A controlled deployment process is also an essential component of meeting the requirements of GDPR Article 22. This article restricts the use of analytical profiling on personal data unless strict conditions are met (for example fully informed consent). Controlled deployment allows full understanding of which data have been used in the AI process and which analytical models have been applied to the data at any one time, essential in determining whether the regulation has been breached.
In conclusion, data processing is an integral part of the AI process which will have a significant impact on the results of AI. Understanding how that processing was performed is a key part of maintaining transparency, one of the key pillars of fair, trusted and effective AI.
Join our AI experts on the “Data Management for Successful AI” webinar to learn how AI can help secure the future of your organisation. Sign up here.
It is also possible to sign up for the recording of the webinar here.