“There are known knowns … There are known unknowns … There are also unknown unknowns”
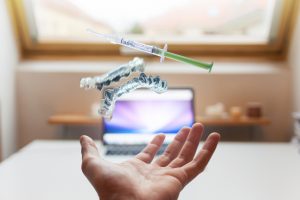
This statement by Donald Rumsfeld is famous from the world of politics. But the phrase “unknown unknowns” was actually coined by a NASA data scientist to describe the huge possibilities presented to us by big data – possibilities that go beyond our current understanding of the world.
It refers to how data can be mined for more than just evidence to support specific hypotheses (the known knowns and known unknowns) to find new patterns and relationships that allow the pursuit of entirely new strategies (the unknown unknowns).
This is the new data world that pharma companies are now tapping into, moving from operational inquiry-based analytics to embrace new possibilities from data science. And the stats back it up. It’s been reported that data science roles have grown over 650 percent since 2012. And the Harvard Business Review has even gone so far as to say that data scientists have the sexiest job of the 21st century.
But despite all the noise, there’s often a lack of clarity on what data science really is – and how it differs from traditional data analytics.
Defining the difference
In a nutshell, data analytics supports operational decisioning and is focused on business process. It is useful for relating trends and patterns to specific business questions, and for supporting specific hypotheses in the context of “known knowns” and “known unknowns.” Data analytics typically uses historical data to provide insight on what’s already happened, and is used to measure events in the past, present or future to address specific business questions or evidence delivery of business goals.
Data science, on the other hand, presents completely new ways of supporting the pharma business model. It exploits the vastness of scientific research and business information to provide actionable insights that have a meaningful impact on business strategy. Data science delves into the world of the “unknown unknowns,” attempting to discover previously unseen patterns and relationships across often-disparate data sets that offer future strategic value to the business.
#DataScience presents completely new ways of supporting the #pharma business model. Find out how #RealTimeData & intelligent systems can support safety research in #healthcare and #lifescience. Click To TweetDevelopment, discovery and differentiation
These higher-level strategic insights, often driven by machine learning, present a huge opportunity for pharma companies to differentiate themselves in the marketplace through earlier discovery of breakthrough new medicines and treatments. Data science can reveal insights across all areas of the business:
Clinical development. Drawing on multiple repositories of molecular, clinical and real-world evidence data (e.g., from research papers, patents, clinical trials and patient records), data science can discover unseen relationships between your chemistry and biology IP and new disease targets.
Drug discovery. Searching across more than a billion known and inferred relationships between biological entities (e.g., genes, symptoms, diseases, proteins, tissues, species and candidate drugs), data science offers massive potential for rational drug discovery and competitive differentiation.
Drug development. Using self-organising maps and dimensionality reduction to identify similar patents, data science can be applied forensically in the patent space to avoid litigation and accelerate drug development.
From trials to treatments
Beyond early development, data science is also impacting late-stage research and subsequent commercial activity when drugs go to market:
Clinical trials. Monitoring trials in real time can help identify safety or operational issues that require rapid action to avoid adverse events or unnecessary and costly delays.
Patient adherence and brand loyalty. Valuable market insights can be extracted from sales field data and physician health records using natural language processing (NLP), which can lead to better patient adherence and fewer health care practitioners (HCPs) switching brands.
Commercial optimisation. Data science can also improve patient-doctor matching for better targeting, provide 360-degree views of patient treatment histories and detect commercial fraud by examining issues such as off-label drug use.
Based on these new insights driven by data science, pharma companies are transforming their business models, seeking to develop new market strategies and delivery mechanisms for the therapies they wish to introduce to HCPs and patients. For example, SMS-oncology is augmenting its cancer research with analytics, using data science to simplify large quantities of results data and better analyse the effectiveness of treatments.
Applying data science to data streaming
The benefits of data science don’t stop at the analysis of disparate “batch” data sets. Machine learning is now capable of touching streaming data at the edge, too – i.e., doing analytics in real time at the point where data is generated by sensors and equipment. This will allow insights to be garnered from the temporal nature of data, and enable business decisions to be made proactively in the moment, eliminating the latency of data having to be transferred back to a central system before analytics can be applied.
The full extent of these emerging capabilities is relatively “unknown” at this stage, but exciting possibilities are starting to emerge:
Real-time demand forecasting. Modern medicines that are sensitive to transport, time and temperature require careful management of inventory levels and distribution. Real-time data analysis will enable far more accurate demand forecasting of those drugs, allowing companies to manage their supply chain more efficiently and generate additional revenue.
Internet of Medical Things (IoMT). The widespread adoption of connected medical devices and apps, including Apple Watches, iPhones and Samsung Gear smartwatches, provide whole new data sets and digital health markers for organisations to work with in real time. This will inform new treatment development, allow better monitoring of drug adherence, encourage lifestyle changes that maximise drug performance and improve drug safety by identifying adverse reactions in real time.
A big challenge for pharma will be how to make best use of all the innovation happening at the edge. Organisations will need to expand their data science architecture so they can perform real-time analytics on incoming data streams and eliminate the latency that occurs when analysing data at rest.
If successful, this will allow more immediate actions to be taken that create positive outcomes for both patients and the business. It’s what we refer to as “multiphase” analytics, using machine learning and automated responses to take traditional analytics to the next level.
If you are interested in finding out more, I can recommend this webinar, led by my colleague Mark Wolff, who is Chief Health Analytics Strategist at SAS. Mark’s current work is entirely focused on this area, exploring how real-time data and intelligent systems can support safety research in health care and life sciences.
I’d also be more than happy to connect for a discussion on your data science strategy – you can reach me at mark.hanson@sas.com.