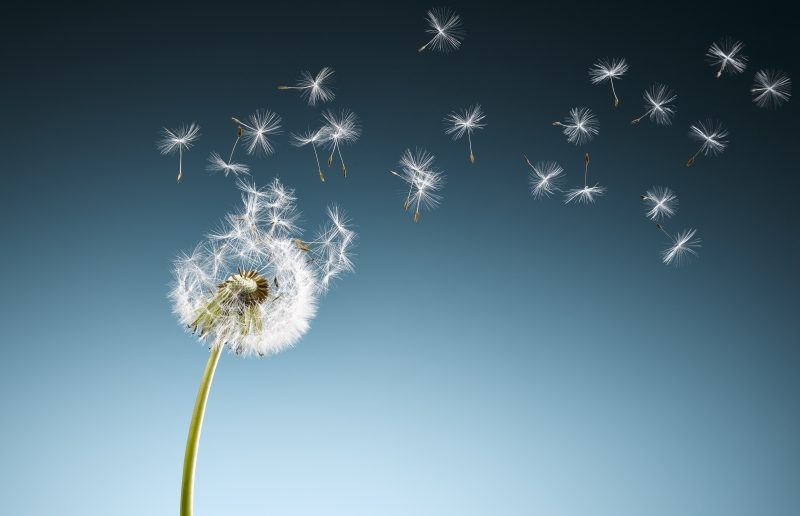
Over the last few years, a quiet and gradual, but nonetheless important, evolution has been taking place. There has been a move from traditional business intelligence or analytics software towards the use of open platforms that underpin analytics activity, and can integrate customers’ own solutions, reducing reliance on proprietary software. Today, analytics platforms are relatively mature, even if there is still a question about why they are not being more widely used.
Sowing the seeds for the analytics platform
The first few seeds of the analytics platform were probably sown around eight years ago, when a customer in Singapore complained of trouble with the amount of data that needed analysing. To be competitive, they needed to shorten their risk calculation batch dramatically, and traditional ways of handling massive computation were not sufficient. So a new solution was required, and the first steps of the analytics platform were born.
Today, far more organisations face the same problem. They have huge amounts of data and are struggling to store it accessibly and run analyses fast enough, or across geographic locations. Unsurprisingly, however, they still want to get full value from their data. It is hardly astonishing that these organisations are now turning to analytics platforms. A platform of today needs to utilise the benefits of a cloud architecture, which means that it can spin up the necessary required compute power fast enough to take on any load of data for transformation and computation.
Early solutions of parallel processing across a cluster of servers had the power to reduce an 18-hour analysis job to just under 15 minutes. More recent developments have embedded the parallel processing into a cloud-native architecture with scalable services, encryption and flexible deployments from bare-metal to cloud and container/kubernetes deployments. Massively parallel processing (MPP) and cloud-native architecture offer more resilience, reliability and scalability – more uptime and speed, in other words.
The joy of this kind of platform is its flexibility. Customers can run a wide range of systems, and it does not matter where they run them; it might be Amazon, Azure, on their own hardware or even a hybrid solution. Analytics platforms are ideal for large companies, even in highly regulated sectors like health care and banking, but they can also work effectively for smaller companies through managed analytics services providers, offering “software as a service” (SaaS).
Taking a platform approach
Our recent surveys suggest that fewer than one third of companies have a culture of data analysis. The least mature tend to run data science and analytics like a project. The best, however, use a platform approach, with an analytics platform underpinning everything that the company does. A full platform approach can seem complex to implement. Companies have found, however, that a platform is best thought of not as technology, but as a way to get things done. In particular, it allows the company to integrate and collaborate with data and analytics, across department functions, in a way that was not previously possible.
An #AnalyticsPlatform is best thought of not as technology, but as a way to get things done. #analytics Click To TweetThis, in turn, makes it possible to pull together data from a wide range of sources. Different functions and roles have access to the same data, and the company can therefore build a much more detailed and complete picture of each customer. Even more crucially, everyone has access to the same information and picture, and can therefore respond to customers appropriately. This is ideal because customers do not distinguish between channels; they merely see that they are in contact with a company in the way that is most convenient at the time.
Implementing an analytics platform has shown great promise in growing the strategic value of analytics and in fostering innovation. These are just two findings from new research consisting of in-depth interviews with professionals in 132 organizations and a global online survey. Download your copy here.
Adding artificial intelligence
As artificial intelligence is added, a platform will become even more essential. And AI is not only about creating intelligence that can be added to existing client solutions using APIs. It is just as much about utilising advanced analytics within the platform to simplify and speed up efficiency. When an analytical platform holds your data, it can start to look at your data at the minute you want to start to work with it. When the platform starts to provide suggestions and insight as to what your data looks like, how you can improve it and what variables have significance to what world problems you are seeking to solve, then you change the game of how you work with your data. And from the hundreds (or thousands in really complex AI models) of parameters you can use to tune your model, why not have the platform suggest them for you? Applying analytics on analytics and the complete analytical life cycle can improve your work dramatically.
I do not want to dwell too much on AI, because an analytics platform is actually so much more – and so much more immediately useful. It is easy to get lost in the hype of AI and forget that other algorithms are currently far more widespread, and provide the needed predictions, optimisation and answers that can give full value in the moment. However, it is also clear that AI algorithms need huge amounts of training data, and that an analytics platform is a very good way to manage massive amounts of data effectively, with the flexibility to scale processing power as needed.
Crucially, platforms offer a way to integrate and automate boring tasks like data sourcing and data management. This frees up time to do more complex analytics, but also to collaborate and cooperate on a far wider basis. Whatever you want to do with analytics, the time is coming when a platform will be considered an essential part of the toolkit and key to drive an “analytics by default” culture in the company.