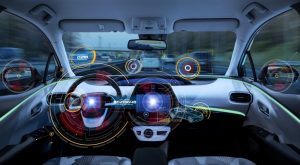
Blinking, I can barely see what’s in front of me. The sun is shining right in my face. The light is blinding and intense – I realise I have to be vigilant. I do my best to see what’s in front of me because I’m travelling at 60 kmh, and I feel like I can barely see anything. All around me are cyclists travelling the same direction as me. Some of them are quite close to my car, and they’re talking as they ride.
That’s kind of what it’s like in Heidelberg when we take a drive through the city centre under bright blue skies in the summer. And sometimes we’re in a hurry. The fact is, a whole lot of cyclists – and not just cyclists – are in constant motion all around us. What is happening when we drive a car? We make decisions while we keep on driving, we obey traffic laws, but we also act intuitively. If a bright sun on the horizon causes poor visibility, we drive very carefully. Will we remember the pedestrian crosswalk that comes right after we cross the bridge? We might even stop out of caution and take a good look to make absolutely certain that there aren’t any cyclists about to go through the crosswalk at high speed. We don’t want to kill or injure anyone!
But how does that work with self-driving cars? How can a computer make all these decisions so quickly? How does it know at what angle the sun is entering the vehicle’s sensors, which are located at various points around the entire exterior of the car? Is it based on 10,000 rules that a human being has determined in advance and given to the computer and its control unit to execute?
Was that the reason for the fatal accident when an autonomous vehicle on autopilot traveling approximately 64 kmh collided with a pedestrian in the US city of Tempe, Arizona – without even hitting the brakes? Police Chief Sylvia Moir told the San Francisco Chronicle that video footage from a camera of the Uber vehicle showed that the woman stepped right out of the shadows and into the vehicle’s path. “It’s very clear it would have been difficult to avoid this collision in any kind of mode (autonomous or human-driven).”
Learning - more and more, always better
But what’s behind the mathematics, artificial intelligence and machine learning that allows these vehicles from Google, Tesla, Uber or even Mercedes-Benz – which is already using autonomous vehicles in the Stuttgart Metropolitan region, according to the Frankfurter Allgemeine – to drive themselves?
What’s behind the mathematics, #AI and #MachineLearning that allows vehicles to drive themselves? #PredictiveModelling Click To TweetWhat’s being used is a type of mathematic formulas called predictive models. These models are able to learn from a very large quantity of collected data. And as more data is gathered, they keep on learning. How do they do it? By using iterative procedures that always require a whole lot of data as inputs and then processing those inputs according to an algorithm that a person, not a machine, has specified to have a better chance at achieving specific results, a partially predetermined result, or a completely open result – freestyle, so to speak.
Deep learning algorithms are one such process, and they’ve been the object of considerable hype in the media. I feed the entry layer of these neural networks with massive quantities of data until I achieve the desired quantity or quality of results. Which formula does that result in? For lots of business processes within a bank, that is exactly the problem. With processes that are dominated by regulatory considerations, for instance, these formulas are so long and complex that they are not able to clearly convey to people what they contain.
For a self-driving car, however, the transparency of the formula is not so important. What’s far more important is that these algorithms that we expect to function in the real world, on the road, are fed with lots of high-quality data. Let’s look at it this way: If I record data for a full year from a vehicle that has 10,000 sensors installed as it navigates all imaginable traffic situations, I can take this mountain of data and construct mathematic models. These models are able to supply an autonomous vehicle with correct predictions – for instance, when sunlight is shining into the sensor at a particular angle, there’s a shadow on a second sensor at the same time, and speed is higher than 20 kmh, then it should come to a complete stop. Of course, this is an oversimplified example.
And in banking?
Now let’s look at banking. If I record the mouse clicks of a bank clerk for two years while continuing to record the data that arrives at the clerk’s desk three times a day, then define what the clerk is supposed to do with this data and which decisions are made with it, I’ll have enough data to construct a predictive mathematical model.
For instance, let’s assume my job is to do double-entry bookkeeping for tax-related transactions, entering debits and credits into a journal. This tax-related information changes over time. My objective is to achieve the best possible tax outcome for my employer, taking into consideration current tax regulations. At this point the only question is which entries can be fully automated – because there is no latitude at all in how the transaction must be handled – and which entries require personal experience and intuition, i.e., which ones make me think.
Find out more in our report "How will AI transform banking?"
When algorithms map human intuition...
It’s precisely this intuition that I’ve been relying on for the last two years – and it’s all been recorded in the data. So now it can be replicated in the form of a predictive model. This is precisely where the opportunity is with machine learning – in a bank’s back-office processes. And it doesn’t matter one bit if the mathematical model is simply an assistant I can use as needed, or whether my job as a specialist can be completely replaced by it.
Don’t get me wrong: For my own personal situation, it certainly does matter! I would be afraid of losing my job, of being replaceable. But from a mathematical perspective, it’s irrelevant. The bank can decide for itself what standard or quality level is applied to determine when the predictive model works better and more efficiently than a human and whether it chooses to replace or assist its staff.
There’s no reason to not take another two years and continue testing to find out if I or my little assistant – let’s call him Robo A – gets better results. At that point, I may be able to put my specialised knowledge to better use completing much more valuable tasks while my little helper Robo A takes care of the grunt work for me.
Perhaps, when larger sums of money are involved, it would not be wise to, for instance, completely replace an investment broker with an algorithm. But just think if we had an assistant that was always there to give us a nod of approval (when we made the right trade) or warn us if it could predict that this trade will, in all likelihood, not have the desired outcome. And again, please don’t misunderstand me – no one is able to perfectly predict the stock market, not even with machine learning algorithms. But these algorithms are capable of replicating the intuition used in bank processes.