It is a truth universally acknowledged – if not always acted upon – that advanced analytics, and AI in particular, need data. What’s more, it needs lots of data, and it needs to be relevant and of good quality. Companies with access to more data tend to perform better, which is why Amazon, for example, has been able to move so far ahead of any competitors, online or offline.
Why is this so? The algorithms behind our artificial intelligence systems, often different deep learning algorithms, need a lot of data. More data means better trained algorithms, which means more precise and therefore more accurate predictions. This, in turn, means better, evidence-based business decisions. Provided that the data is of good quality, of course, and not biased. AI is only as good as the data that powers it. These algorithms learn everything from data. If the data is reflecting bias, discrimination or similar, then so will the AI system. Considering that technology today enables AI systems to make decisions with incredible throughput, potential bias can quickly be amplified. Algorithm audits and more interpretability of machine learning models are crucial.
Not all companies are ready to use their data, even if sufficient data of adequate quality is available. All this boils down to a question of data management and governance, and it is one that many companies are still failing to grapple with successfully.
Same old, same old?
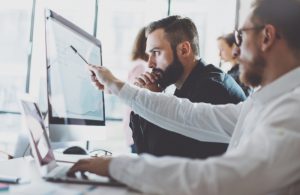
This is not a new issue. Colleagues have been pointing out for some time that there is a lot of hype about AI, but a shortage of practical use cases. There are a number of possible reasons for this, but it is feasible that part of the cause is that companies are still unable to use their existing data effectively. This makes it much harder to ramp up analytical efforts and take advantage of more sophisticated solutions. After all, if you cannot walk, you can hardly start running or dancing. If your existing processes do not give you the information that you need to manage customer relationships and experiences well, then automating them will not help, however much new data it generates.
Becoming data-driven is about far more than automation or introducing new technology. Instead, it requires companies to redefine their processes, looking at them from the customer’s perspective, and bringing together data from all sources that can help to map the customer journey. These can then be used to improve customer experience. Many companies, however, do not even know what data they are already holding because the information is spread across different silos and never brought together.
The General Data Protection Regulation (GDPR), which came into force last month, should already be acting as the catalyst to improve that situation. The vast majority of companies see that compliance is not just a requirement but could also provide benefits. But seeing the potential and achieving it are two very different things. A fair few companies are still assuming that some kind of miracle will happen that will magically convert their manually held data into insights. Unfortunately, there are no miracles in data governance, only hard work. Those companies that put the work in to align data governance will reap the benefits. Others will not, and will also be running the risk that their efforts are not GDPR-compliant.
Rethinking data
Suppose, however, that you have taken advantage of compliance to get on top of your data. Suppose that you now know what data you are holding, and where, and have started to bring your sources together. You should at this stage also be managing data adequately to assure the quality. It seems likely that you will have started to map data to the customer journey and noticed something. In fact, the most likely scenario is that you are now at least slightly aware of where there are gaps in your data and are wondering what to do about it.
The answer is to measure more. Things are only unquantifiable while you do not measure them. Once you start measuring, you will have more information, and be able to understand and generate more insights. This approach is clearly correct when you start to look at how sport is harnessing analytics, and, indeed, how analytics is shaping sport. Player performance, which used to be a matter of gut instinct and feel in football, for example, has become much more quantifiable with the use of cameras and image analysis that can show how a player moves around the pitch, how and when they make contact with the ball, and how they interact with other players and the game.
The question of readiness to use AI, therefore, must include data governance. First, defining what data you already have, and how it can be used, with required assurance. Second, working out what you are missing. Third, collecting that data, and being in a position to start using it to generate insights. Simple, but at the same time a huge challenge.
Josefin Rosén’s blog post was inspired by “Is Your Company’s Data Actually Valuable in the AI Era?” by Ajay Agrawal, Avi Goldfard, and Joshua Gans. Find the original article, as well as those about other important aspects of AI, in the Harvard Business Review report, “Risks and Rewards of Artificial Intelligence.”