In my previous blog post, I talked about how both retailers and consumers can benefit from applications of artificial intelligence and discussed some compelling use cases. I would like to take this post a step further and delve into a particular bugbear of mine: the struggle to find the right size. In addition, I would like to discuss how analytically driven solutions can play an integral role in solving this problem.
As I mentioned in my earlier post, I avoid spending a lot of time shopping. So when I do go out, I really hope I can get what I am looking for. In particular, it’s not only the style or brand that I hope to find – the size has to be right.
A few weeks ago, I went into a large retailer and was looking for some trainers. To my disappointment, they only had the extreme ends of sizes available – very small and extra large. I had this experience on many occasions, and so did my friends and many consumers, no doubt. I thought to myself, How do retailers consistently get this wrong? How can they truly understand customer demand so that this can be mitigated?
The financial impacts
Let’s look at the financial impacts for retailers of not being able to meet customer demand for the right size.
Consider the case of being understocked. This translates into significant amounts in lost sales and more importantly, unhappy customers – which can lead to even bigger revenue loss in the future. The problem with lost sales is that it’s difficult for retailers to track, as they can’t effectively measure it (i.e., what percentage of customers walk out as a result of not finding the right size).
The other side is when retailers have excess stock. In this case, they are forced to massively discount (by markdown) to get rid of excess stock. This also has a negative effect on bottom-line profitably due to the margin reduction.
The analytically driven approach to solving this problem
The first step is to accurately forecast future customer demand right down to SKU/size level. A key thing to note is that historical sales and filtered sales do not capture customer size demand on their own.
The figure below shows the difference between historical sales for women’s ballet shoes compared to the “true size demand.” When we take into account lost sales (which can be done using imputation) due to inventory constraints, we then get the beautiful green line that shows the revised demand (true size demand). The incremental difference between the two (historical and revised) is where the golden nuggets are for retailers. They can use this to calculate what the upside is and how to avoid stock-outs.
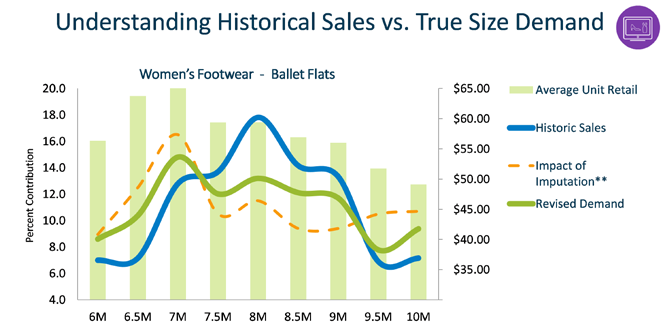
Predictive analytics powered by machine learning can impute (estimate) what could have sold if there were no constraints on inventory. This is shown by the dotted orange line. Optimisation techniques can then redistribute the contribution by size accordingly while keeping inventory levels neutral. For this to be of value, it needs to be done at a granular level of product, location, etc.
The data tells us powerful story. We actually see greater demand for the smaller sizes: 6, 6.5 and 7. This is where stock-outs and unfilled demand occurred. The law of supply and demand justifies this, as we see higher average prices associated with these sizes. When we look at the core sizes (7.5 to 9), we see the effect of markdowns to reduce stock and once again validated by lower average selling price.
The next step is to generate demand-based size profiles across multiple levels of the hierarchy (region, store, size). The idea is to keep inventory levels neutral by providing the optimal ratio of size distribution based on the true size demand. Just consider a typical fashion retailer with a large combination of sizes and stores (this can go into the millions).
The key to getting this right is the ability to do this at scale and with accuracy.
ORDER AND ALLOCATION THE RIGHT SIZES FOR THE RIGHT LOCATION AND CHANNEL
Massive benefits for retailers and customers
For the retailer:
- Increase in profitability through fewer markdowns by reducing excess stock at stores.
- Increase in revenue by meeting customer demand, having the right sizes available at stores.
- Better assortment planning and store operations.
For the customer:
- Better customer experience by having the right sizes.
I found this on-demand webinar video very relevant to the above topic. There we see industry experts tackle this ubiquitous problem for retailers. Webinar: Get Apparel Sizes Right to Solve Markdown Probl
There is also a new white paper as recommended read: Size Optimization Made Easy With Machine Learning and Analytics
1 Comment
Very interesting info!Perfect just what I was searching
for!