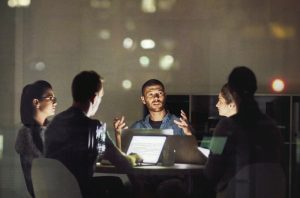
Innovation labs remain an important way of introducing both innovation and analytics to business, especially as artificial intelligence becomes more important. I caught up with Prenton Chetty, Head of Analytics at Nedbank, to discuss how he sees his role changing in the future.
Tell us about your current role at Nedbank.
I head the Advanced Analytics team, and we support both retail and business banking, mostly with predictive modelling. We started off as a statistical area, and now we’re moving into machine learning and deep learning. We do a lot of propensity modelling, churn modelling, collections modelling and other sorts of predictive work, including scorecards, as well as customer insights.
What are your views on an innovation lab?
I think it is helpful to think of the data science team as the innovation lab for analytics in the company. We often have to ask for hardware and software that’s not traditionally used, especially in banking, so we are introducing it to the bank. I don’t think you need a formal innovation lab; what we’re doing is the kind of innovative stuff that should be happening in any data science area.
Do you still need to make a business case for new hardware or software?
Yes, but the process is pretty reasonable. We have to provide business cases for anything we want because we are heavily linked to business impact. We need to show that there’s a reason why we need the new kit. What we do is a bit like piloting, but we still need a justification for the pilot.
You mentioned solving business problems and deriving business value – tell me more.
We have techniques that have improved the accuracy of predictions by around 15 to 20 percent. We’re getting to scary levels of accuracy, especially on the attrition side. Banks have huge amounts of information about customer transactions and behaviour, so attrition modelling can get very accurate. The accuracy has also increased in propensity modelling, and we are using the same techniques to solve other problems and make the analysis easier. We are also finding that the increased accuracy has improved both targeting and business hit rates because we can provide more options. So, for example, the incentive plans you give your customers can be a lot more aggressive because of better accuracy on predicted behaviour and better understanding of the client.
Incentive plans you give your customers can be a lot more aggressive because of better accuracy on predicted behaviour and better understanding of the client.
Can you see a financial impact from these models?
We’re working in a large financial institution where a 1 percent difference can be a substantial amount of money. A move from 5 to 8 percent makes a big difference. I think we’ve seen the most impact on the collection side because traditionally we have not used a lot of analytics there. Being able to say which customers have the highest chance of paying you back means you can start prioritising, and also getting the incentives right. With accurate modelling, you can say, for example, what are the chances of a particular person leaving us, and therefore know how big an incentive we have to offer to keep them – and the cost of doing so. So we can weigh up the cost of keeping them against the profit we expect to make from them, and upfront know the expected impact of our actions. This allows us to do earlier targeting when costs may be lower. We just couldn’t have these kinds of conversations before.
How has this changed the way that the bank makes decisions?
With predictive analytics getting so accurate, we are moving towards prescriptive analytics. That is, we now know with such accuracy what is likely to happen that we can suggest what action to take. This means we can look at the chances of something happening and the most effective action to take as a result. This has definitely changed the decision-making process.
The data science team know with such accuracy what is likely to happen that we can suggest what action to take. #DataScience Click To TweetAre there any softer benefits as well as financial, such as improved customer experience?
Yes, for example, one of our models can look at the products that customers are using and the stage that they’re at, and predict the next product that they might need. This really helps customers, because we can often identify the customer need even before the customer does. It’s more than general life stage analysis, though, because it recognises that individuals may be at different stages even at the same age. This helps us to identify the best option for each client, and that means we can treat them more fairly, and even identify when they are on the wrong product for them. It is very much not about trying to make the most money from each customer, but maximising the benefits and value for them as well as us.