Anomalies: The Beginning of a Crisis
While even trained scientists can fail to see things that fall outside what they are looking for, anomalies eventually start to get noticed. But still, for a long time, anomalies within an existing paradigm are seen as mere “violations of expectation.” The response within the community is to figure out what went wrong – was there a problem with the measurement, or instrumentation, or was there just error in the way the observation was being interpreted?
But eventually, when there are enough observations that cannot be explained under the existing paradigm, then science is thrown into a crisis. It is a period of intense research, and the examination of alternatives.
The only way out of the crisis is the adoption of a new paradigm – a paradigm shift – a phrase that is now in the vernacular thanks to Kuhn’s book.
The Crisis in Business Forecasting
I don’t think we’re yet in this crisis state in business forecasting – although maybe we should be. And maybe readers of this blog can help provoke one.
To a large degree, the Offensive paradigm esteems the mastery of mathematics over an understanding of how business forecasting actually works.* The question is not where the Offensive paradigm has taken us, or whether it has been successful. The Offensive paradigm has been wildly successful, and taken us a very long way over the last 60 years.
The question is where to go next.
Can big data, more esoteric modeling, and more elaborate forecasting processes take us to the next level of improvement in real-life business forecasting? That is my concern. What we’ve failed to see, the anomaly that’s right in front of our noses, is that there is scant evidence this approach can continue to be counted on to substantially improve our forecasts.
High on Complexity
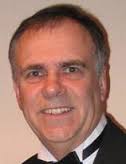
In a 2011 article** published in Foresight: The International Journal of Applied Forecasting, Paul Goodwin referred to this as a love affair with complexity. Goodwin's article pointed to several recent examples of papers…papers that are almost comical to look at.
Here are a couple of the newly proposed techniques, and the evidence offered for them:
- Analytical Network Process – a technique based on relatively complex mathematics that allowed experts to systematically structure their knowledge of the key drivers of sales to make their judgmental forecasts more consistent and accurate.
So it is a way to assist in making judgmental forecasts.
The approach yielded forecasts with a 1.3% error, which was minimal compared to a benchmark of six common statistical techniques applied to the data. This looks great! I’ve been involved in forecasting for over 30 years and I never reach a 1.3% error.
But Goodwin pointed out that the six common techniques the method was compared to were not really appropriate for the type of data they were forecasting, so it isn’t surprising they didn’t do very well. What’s worse, the authors failed to make the most obvious and useful comparison – to judgmental forecasts unaided by their analytical network process. Further, the methods had only been tested on one sales figure! But this didn’t stop the authors from providing 33 pages of discussion and 9 tables of results – including two 13x13 matrices containing figures to five decimal places.
- Seasonal Hybrid Procedure -- a technique that uses an adaptive particle-swarm optimization algorithm to forecast electricity demand.
The model was fit to 57 past monthly observations, and used to generate 9 months of out of sample forecasts. The authors asserted their model was “an effective forecasting technique for seasonal time series with nonlinear trend.” They asserted this without even testing it over a full 12 months!
Goodwin’s conclusion was that “If the name of the method contains more words than the number of observations that were used to test it, then it’s wise to put any plans to adopt the method on hold.” Whoever said that forecasting professors couldn’t be funny?
*This phrase was adapted from John Naughton's 2012 article "Thomas Kuhn: The man who changed the way the world looks at science," in a shrewd comment on the 2008 financial crisis: "...social scientists saw the adoption of a paradigm as a route to respectability and research funding, which in due course led to the emergence of pathological paradigms in fields such as economics, which came to esteem mastery of mathematics over an understanding of how banking actually works, with the consequences that we now have to endure."
**Paul Goodwin, "High on Complexity, Low on Evidence: Are Advanced Forecasting Methods Always as Good as they Seem?" Foresight 23 (Fall 2011), 10-12.
[See all 12 posts in the business forecasting paradigms series.]