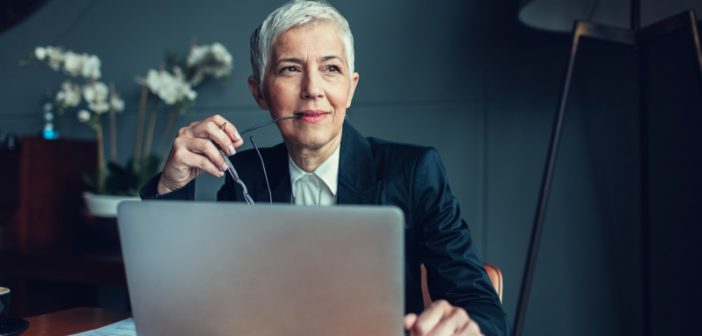
Jim Harris addresses some of the most common questions and challenges big data poses for data quality.
Jim Harris addresses some of the most common questions and challenges big data poses for data quality.
.@philsimon on bridging the IT-business divide once and for all.
As a youngster in the 70s and 80s, Star Trek inspired my imagination and fostered a great love for science, technology and reading. (See the embedded Star Trek infographic for some interesting factoids – did you know that there were 28 crew member deaths by those wearing red shirts?) Captain Kirk and the
Data integration, on any project, can be very complex – and it requires a tremendous amount of detail. The person I would pick for my data integration team would have the following skills and characteristics: Has an enterprise perspective of data integration, data quality and extraction, transformation and load (ETL): Understands
Integrating big data into existing data management processes and programs has become something of a siren call for organizations on the odyssey to become 21st century data-driven enterprises. To help save some lost time, this post offers a few tips for successful big data integration.
There is a time and a place for everything, but the time and place for data quality (DQ) in data integration (DI) efforts always seems like a thing everyone’s not quite sure about. I have previously blogged about the dangers of waiting until the middle of DI to consider, or become forced
Bigger doesn’t always mean better. And that’s often the case with big data. Your data quality (DQ) problem – no denial, please – often only magnifies when you get bigger data sets. Having more unstructured data adds another level of complexity. The need for data quality on Hadoop is shown by user
.@philsimon on whether companies should apply some radical tactics to DG.
If your organization is large enough, it probably has multiple data-related initiatives going on at any given time. Perhaps a new data warehouse is planned, an ERP upgrade is imminent or a data quality project is underway. Whatever the initiative, it may raise questions around data governance – closely followed by discussions about the
In recent years, we practitioners in the data management world have been pretty quick to conflate “data governance” with “data quality” and “metadata.” Many tools marketed under "data governance" have emerged – yet when you inspect their capabilities, you see that in many ways these tools largely encompass data validation and data standardization. Unfortunately, we