.@philsimon on the downside of the Band-Aid approach.
Tag: data management for analytics
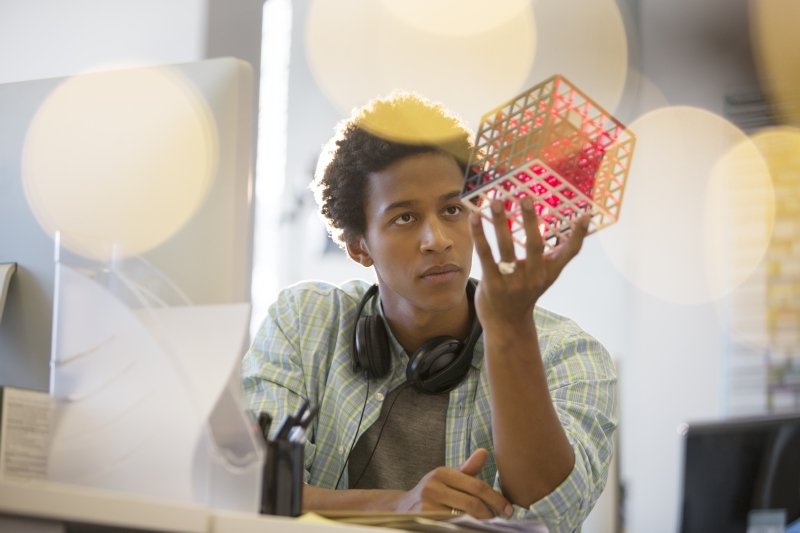
Critical business applications depend on the enterprise creating and maintaining high-quality data. So, whenever new data is received – especially from a new source – it’s great when that source can provide data without defects or other data quality issues. The recent rise in self-service data preparation options has definitely improved the quality of
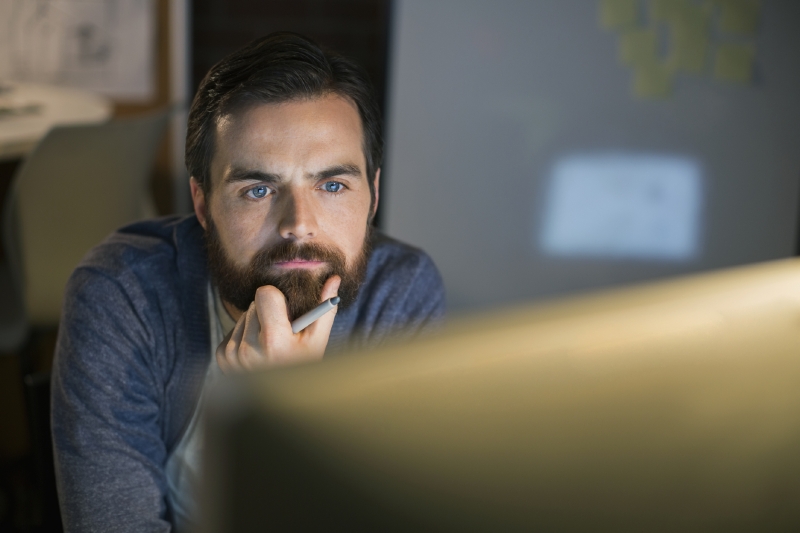
Hadoop has driven an enormous amount of data analytics activity lately. And this poses a problem for many practitioners coming from the traditional relational database management system (RDBMS) world. Hadoop is well known for having lots of variety in the structure of data it stores and processes. But it's fair to
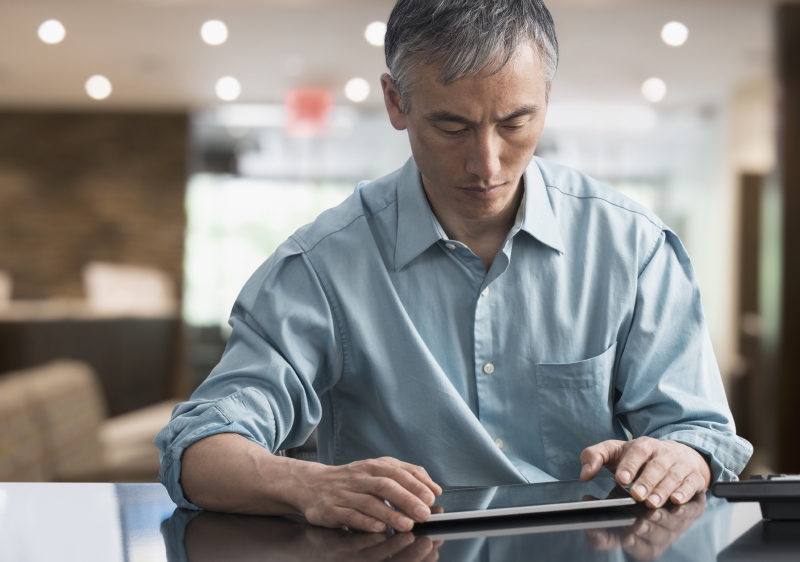
.@philsimon continues his series on data prep and anlytics.
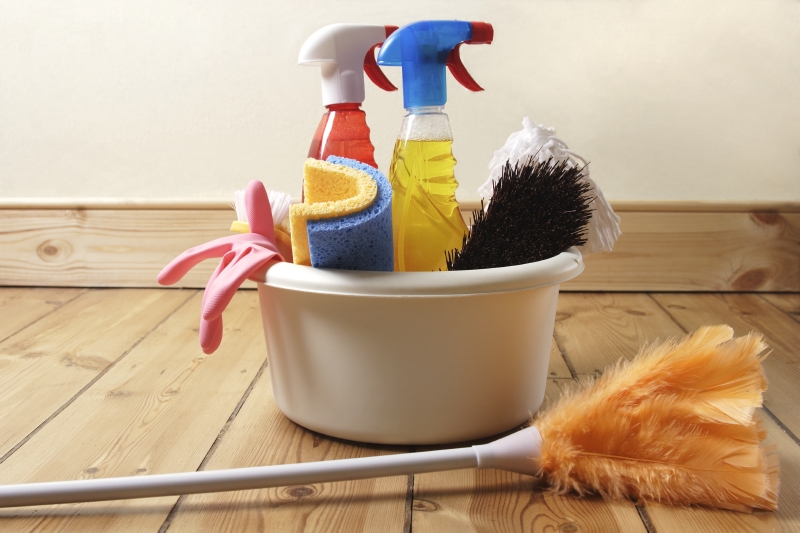
In my last post, I talked about how data still needs to be cleaned up – and data strategy still needs to be re-evaluated – as we start to work with nontraditional databases and other new technologies. There are lots of ways to use these new platforms (like Hadoop). For example, many
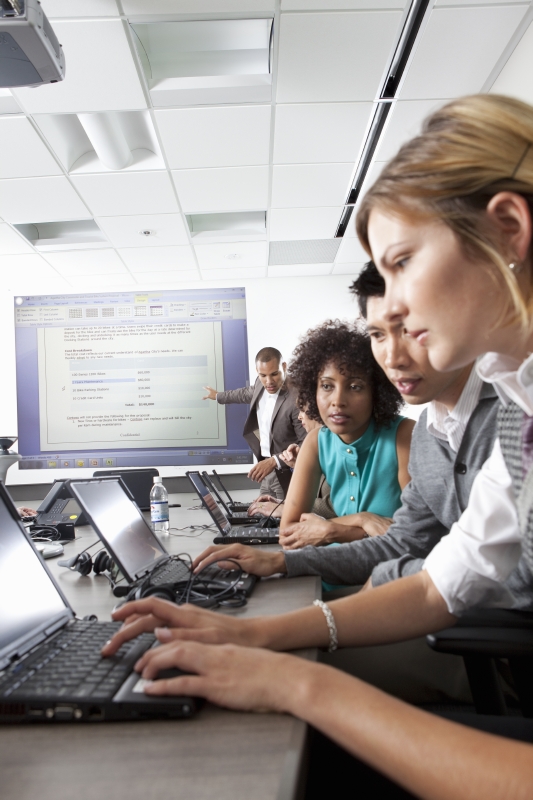
I'm hard-pressed to think of a trendier yet more amorphous term today than analytics. It seems that every organization wants to take advantage of analytics, but few really are doing that – at least to the extent possible. This topic interests me quite a bit, and I hope to explore
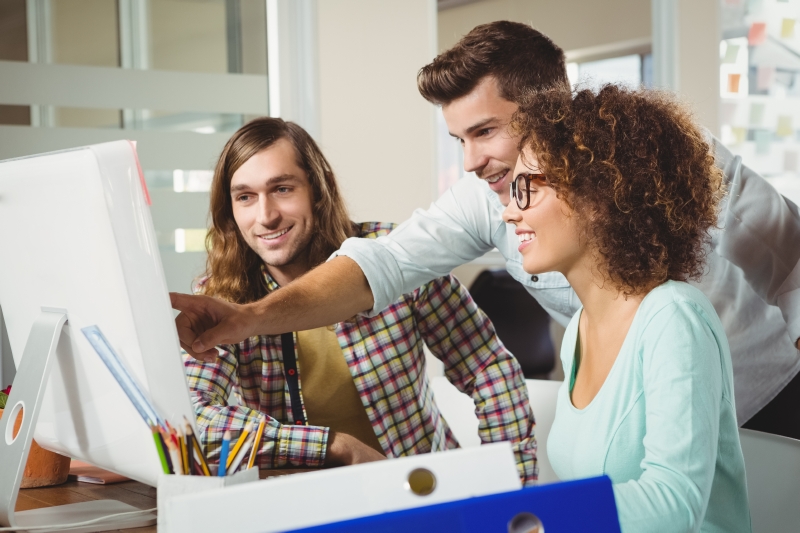
What does it really mean when we talk about the concept of a data asset? For the purposes of this discussion, let's say that a data asset is a manifestation of information that can be monetized. In my last post we explored how bringing many data artifacts together in a
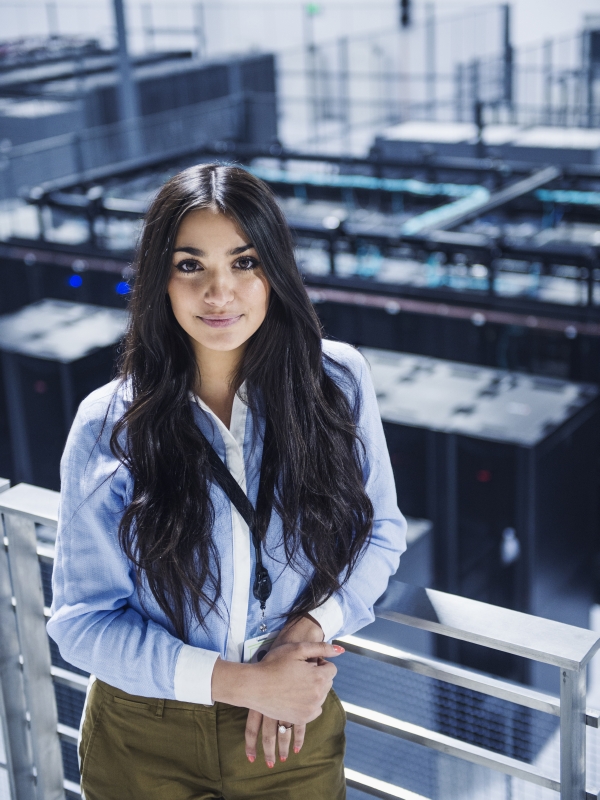
If your enterprise is working with Hadoop, MongoDB or other nontraditional databases, then you need to evaluate your data strategy. A data strategy must adapt to current data trends based on business requirements. So am I still the clean-up woman? The answer is YES! I still work on the quality of the data.
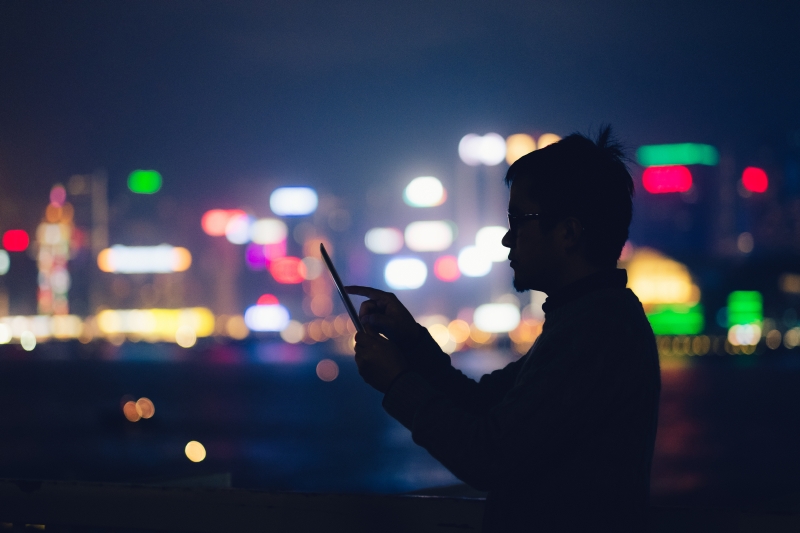
The demand for data preparation solutions is at an all-time high, and it's primarily driven by the demand for self-service analytics. Ten years ago, if you were a business leader that wanted to get more in-depth information on a particular KPI, you would typically issue a reporting request to IT
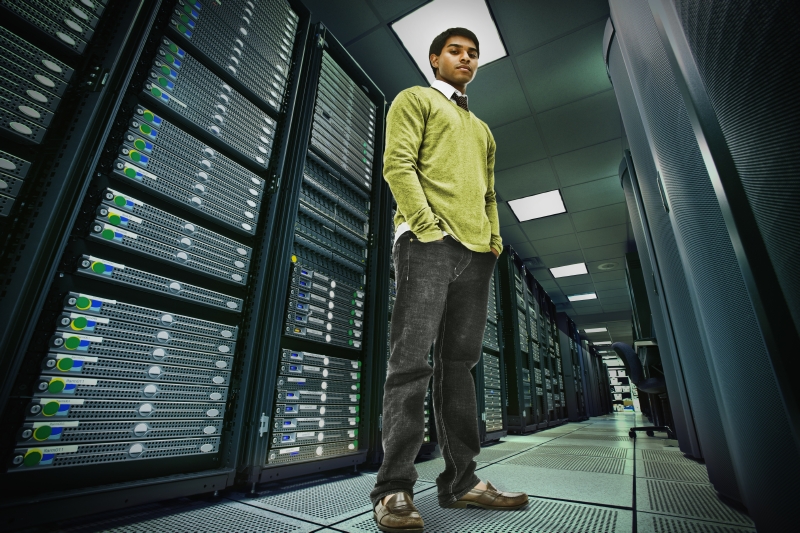
A long time ago, I worked for a company that had positioned itself as basically a third-party “data trust” to perform collaborative analytics. The business proposition was to engage different types of organizations whose customer bases overlapped, ingest their data sets, and perform a number of analyses using the accumulated