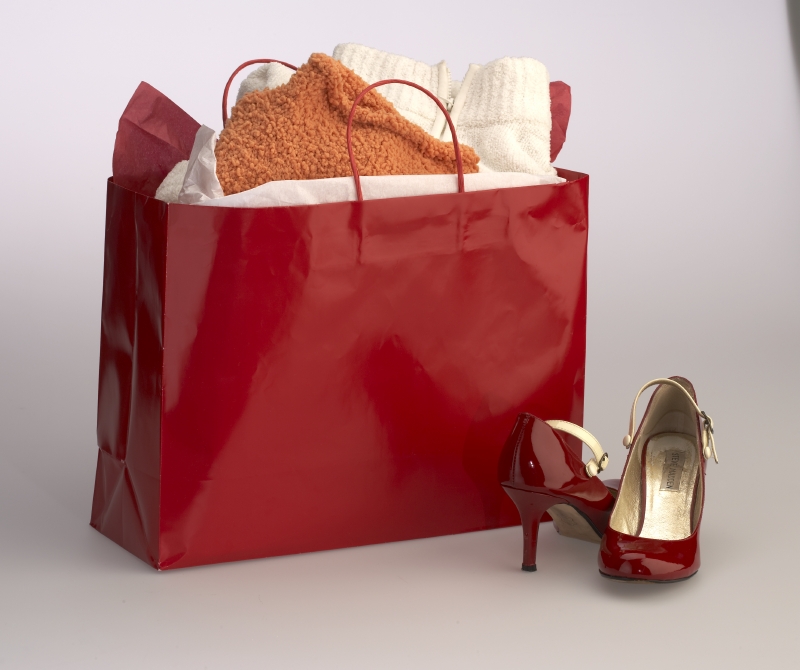
How many times have you gone onto a website, put a few things in a shopping cart, and then exited the Internet? I do it all the time. Sometimes when I log on to that site during my next visit, those same items are still in my cart – ready for purchase. I find